A New Insight on Augmented Lagrangian Method with Applications in Machine Learning
Journal of Scientific Computing(2024)
摘要
By exploiting double-penalty terms for the primal subproblem, we develop a novel relaxed augmented Lagrangian method for solving a family of convex optimization problems subject to equality or inequality constraints. The method is then extended to solve a general multi-block separable convex optimization problem, and two related primal-dual hybrid gradient algorithms are also discussed. Convergence results about the sublinear and linear convergence rates are established by variational characterizations for both the saddle-point of the problem and the first-order optimality conditions of involved subproblems. A large number of experiments on testing the linear support vector machine problem and the robust principal component analysis problem arising from machine learning indicate that our proposed algorithms perform much better than several state-of-the-art algorithms.
更多查看译文
关键词
Convex optimization,Augmented Lagrangian method,Relaxation step,Convergence complexity,Machine learning,65K10,65Y20,90C25
AI 理解论文
溯源树
样例
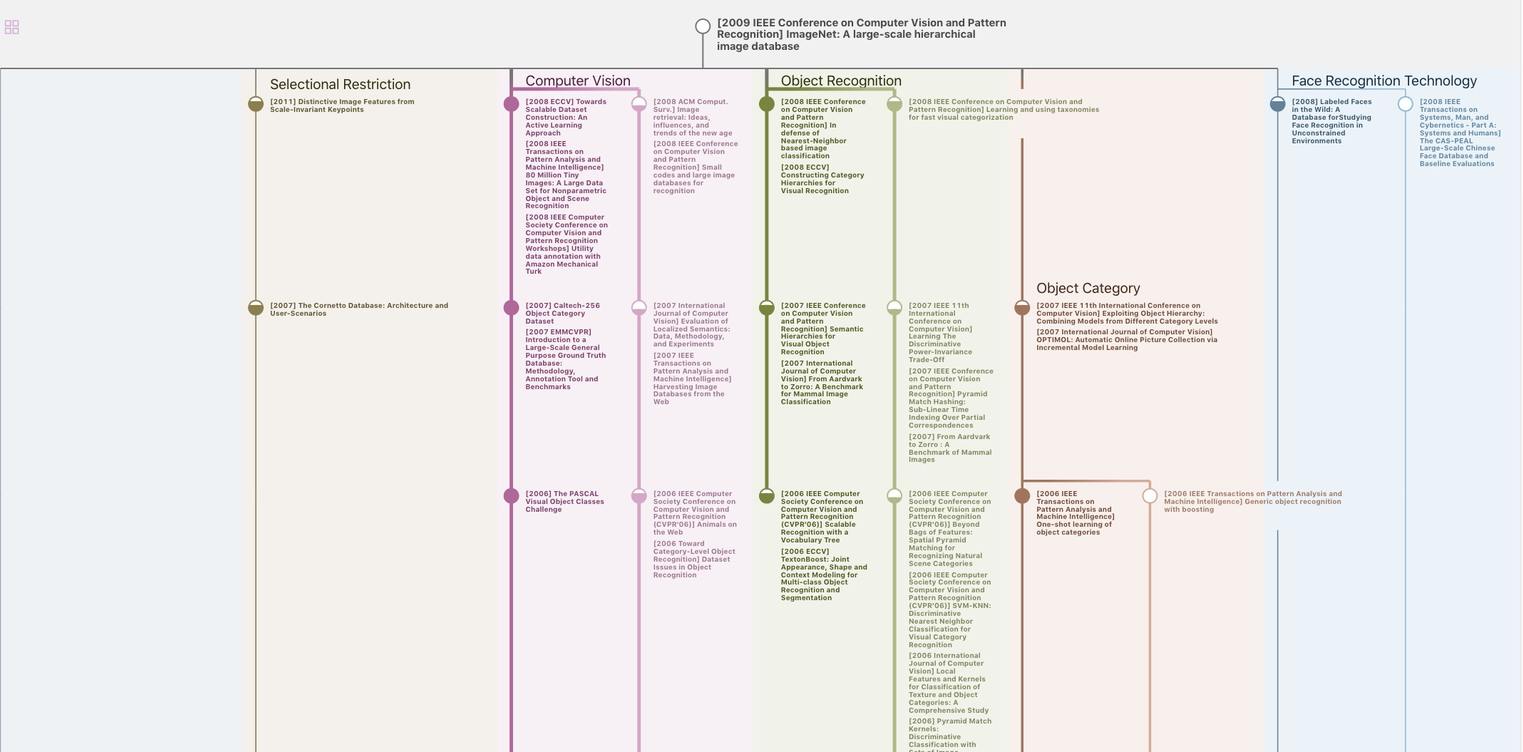
生成溯源树,研究论文发展脉络
Chat Paper
正在生成论文摘要