Home-based resistance training performed at either fast or slow speeds improves power output in older adults
Experimental Gerontology(2024)
摘要
Purpose
We investigated the effect of an unsupervised, body mass- home-based resistance training program in older adults performed at either a fast or slow contractile speed on changes to muscle-power, -volume, -architecture, and fatigue resistance of the knee extensors.
Methods
Thirty-two male older adults (age 65–88 years) were separated into 1) fast-speed exercise (Fast-group), 2) slow-speed exercise (Slow-group), and 3) no exercise (Control-group) groups. Participants in the exercise groups performed 30–45 repetitions of knee-extension and sit-to-stand exercises 3 times a week for 8 weeks with different exercise speed between the groups. Before and after the intervention period, the following variables were measured: Isotonic power, isometric strength, twitch contractile properties, muscle-activity, -architecture, and -quality, neuromuscular fatigue resistance of the knee extensors, and thigh muscle volume.
Results
Peak power was increased in both the Fast-group (+24 %, P < 0.01, d = 0.65) and Slow-group (+12 %, P < 0.05, d = 0.33) but not in the Control-group. Training increased pennation angle of the vastus lateralis in both the Fast-group (+8 %, P < 0.01, d = 0.42) and Slow-group (+8 %, P < 0.01, d = 0.42), while only the Fast-group showed increase in pennation angle of the rectus femoris (+12 %, P < 0.01, d = 0.64) and thigh muscle volume (+16 %, P < 0.01, d = 0.52). There was no time × group interaction effect for the other neuromuscular measures.
Conclusions
Unsupervised, body mass- and home-based resistance training performed at either fast or slow speeds can improve muscle power in older adults, while fast-speed exercise may be preferable over slow-speed owing to the relatively greater improvement of muscle-power, −volume, −architecture, and better time efficiency.
更多查看译文
关键词
Muscle architecture,Body mass-based training,Sit-to-stand,Isotonic contraction
AI 理解论文
溯源树
样例
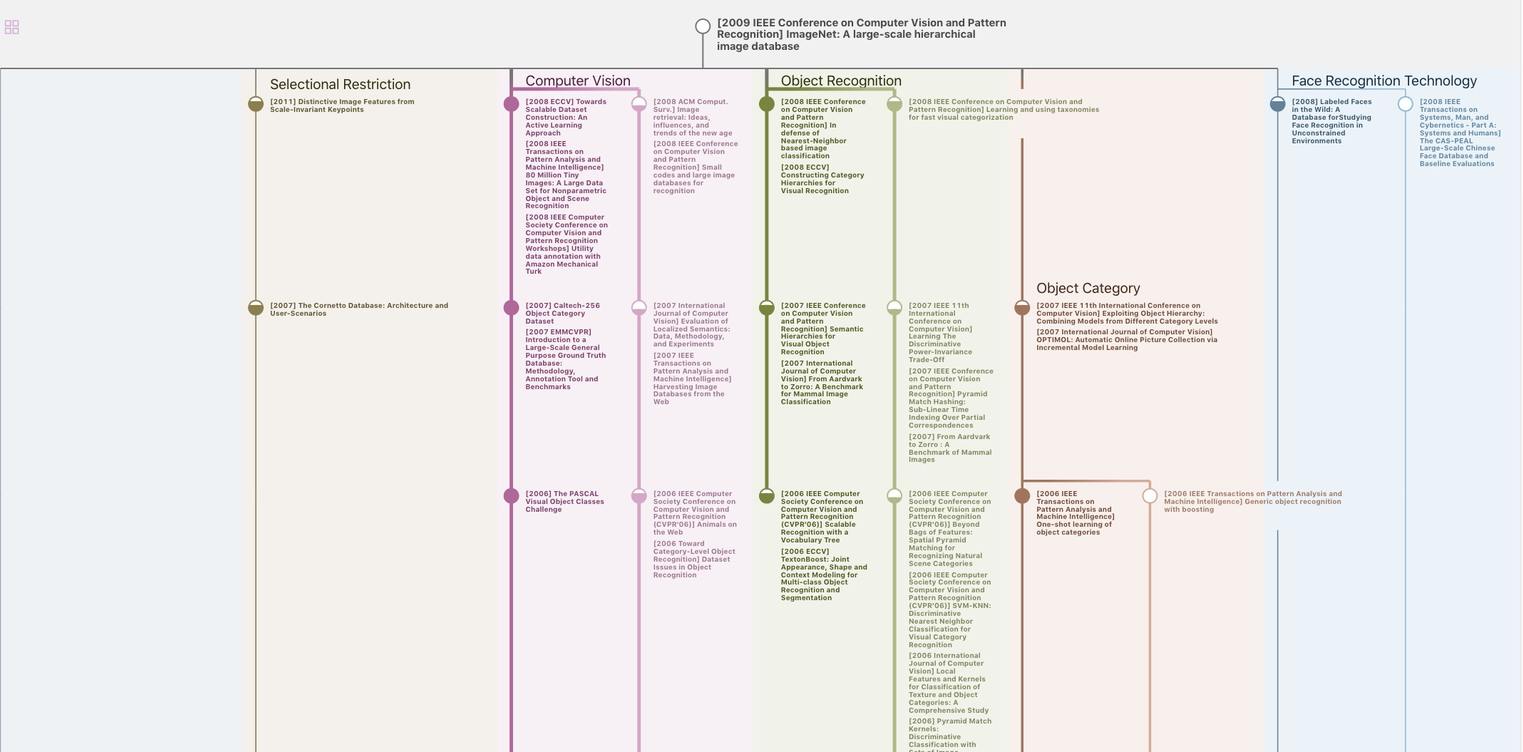
生成溯源树,研究论文发展脉络
Chat Paper
正在生成论文摘要