Golden Gemini is All You Need: Finding the Sweet Spots for Speaker Verification
IEEE/ACM Transactions on Audio, Speech, and Language Processing(2024)
摘要
The residual neural networks (ResNet) demonstrate the impressive performance in automatic speaker verification (ASV). They treat the time and frequency dimensions equally, following the default stride configuration designed for image recognition, where the horizontal and vertical axes exhibit similarities. This approach ignores the fact that time and frequency are asymmetric in speech representation. We address this issue and postulate
Golden-Gemini Hypothesis,
which posits the prioritization of temporal resolution over frequency resolution for ASV. The hypothesis is verified by conducting a systematic study on the impact of temporal and frequency resolutions on the performance, using a trellis diagram to represent the stride space. We further identify two optimal points, namely
Golden Gemini
, which serves as a guiding principle for designing 2D ResNet-based ASV models. By following the principle, a state-of-the-art ResNet baseline model gains a significant performance improvement on VoxCeleb, SITW, and CNCeleb datasets with 7.70%/11.76% average EER/minDCF reductions, respectively, across different network depths (ResNet18, 34, 50, and 101), while reducing the number of parameters by 16.5% and FLOPs by 4.1%. We refer to it as
Gemini
ResNet. Further investigation reveals the efficacy of the proposed
Golden Gemini
operating points across various training conditions and architectures. Furthermore, we present a new benchmark, namely the
Gemini
DF-ResNet, using a cutting-edge model.
Codes and pre-trained models are available at https://github.com/Tianchi-Liu9/Golden-Gemini-for-Speaker-Verification .
更多查看译文
关键词
Speaker verification,speaker recognition,2D CNN,ResNet,stride configuration,temporal resolution
AI 理解论文
溯源树
样例
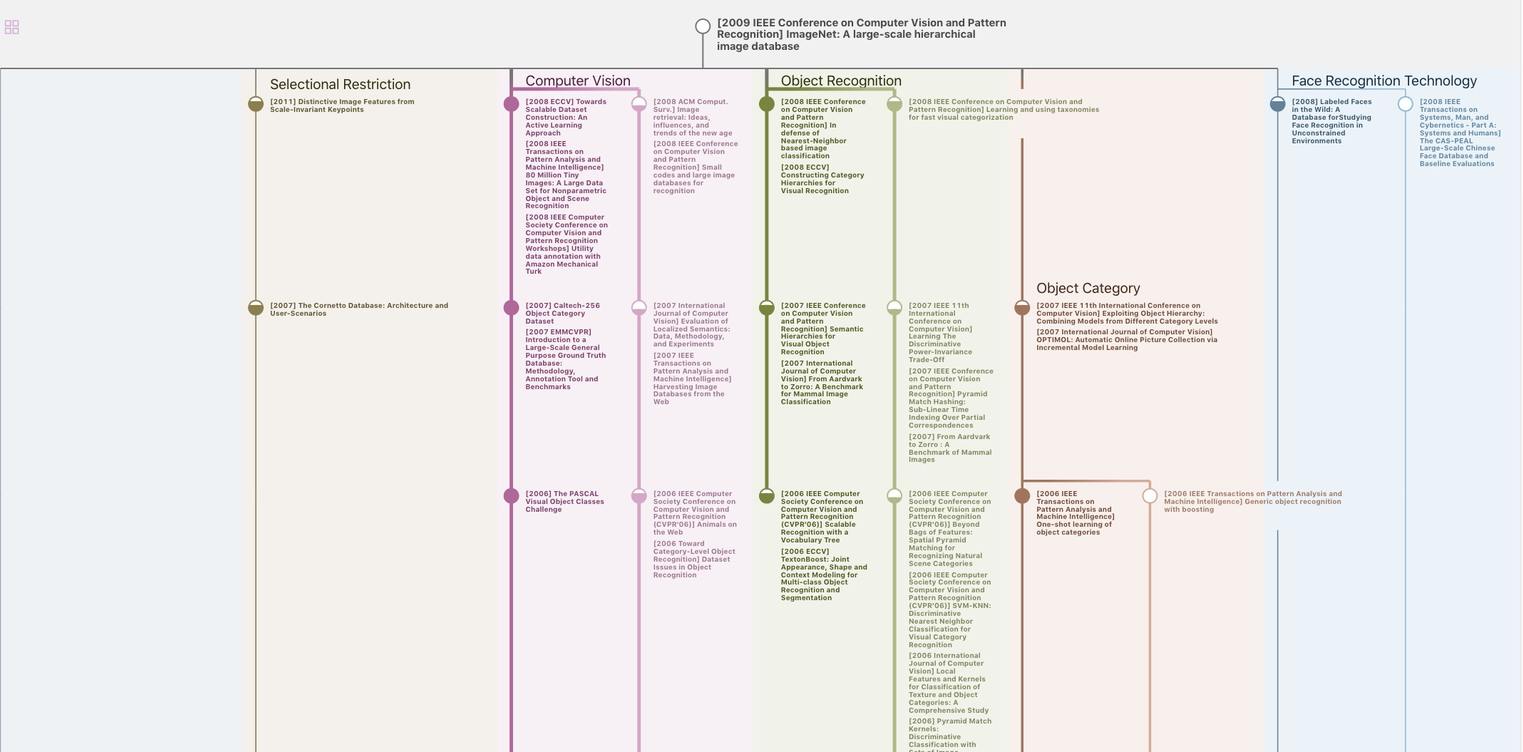
生成溯源树,研究论文发展脉络
Chat Paper
正在生成论文摘要