On-Line Measurement of Iron Grade in Iron Ore Slurry by LIBS Technique Combined With Gaussian Process Regression.
IEEE Trans. Instrum. Meas.(2024)
摘要
Online detection and analysis of slurry composition helps to determine the process performance of the flotation process, which is important for controlling production stability, environmental protection, and resource recovery. Our laboratory has developed a slurry analyzer based on the laser-induced breakdown spectroscopy (LIBS) technique, named SIA-LIBSlurry, which can monitor the mineral grade in slurry online. However, it is challenging to realize the high-accuracy analysis of iron grade at industrial sites due to a variety of factors, such as changes in the mineral matrix and nonlinear coupling relationships. In this paper, a modeling method based on Gaussian process regression (GPR) is proposed for accurate measurement of iron grade. By designing a combination of kernel functions and noise magnitudes, the GPR model can flexibly deal with nonlinear features between data, which is suitable for the LIBS spectral data of iron ore slurries. The results showed that the GPR model achieved the best results with the highest coefficient of determination and the lowest RMSE of prediction compared with conventional quantitative analysis models such as partial least squared regression (PLS), support vector regression (SVR), and ridge regression. The DotProduct+RBF combined kernel function designed in the study better handled the linear and nonlinear relationships among the data, proving that GPR is an effective modeling method. This helps to realize the fast and effective detection of iron elements in iron ore slurry by the SIA-LIBSlurry analyzer.
更多查看译文
关键词
Gaussian process regression,iron slurry,iron grade,laser-induced breakdown spectroscopy,spectroscopy
AI 理解论文
溯源树
样例
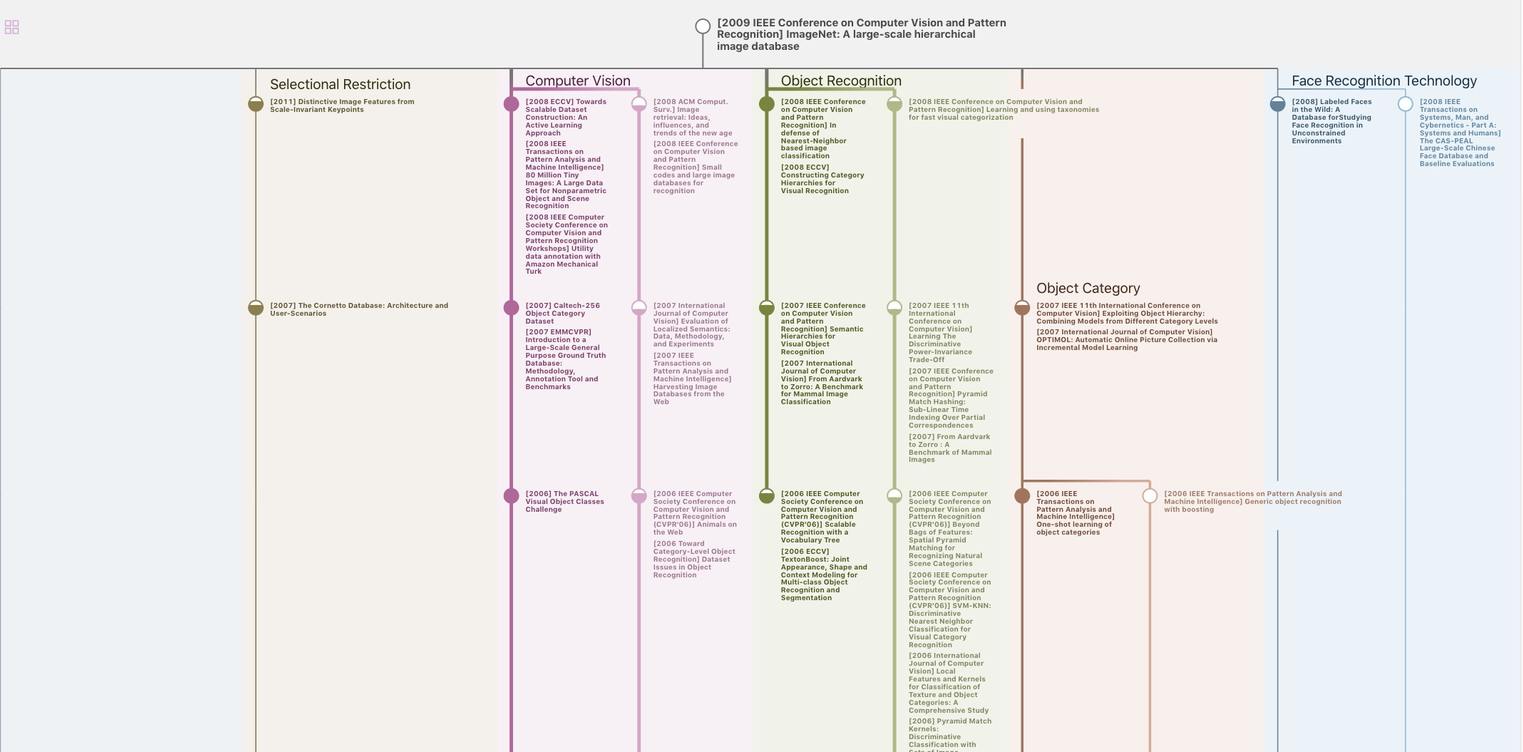
生成溯源树,研究论文发展脉络
Chat Paper
正在生成论文摘要