Neural Network Iterative Learning for SISO Non-Affine Control Systems
Electronics(2024)
摘要
This work introduces an identification scheme capable of obtaining the unknown dynamics of a nonlinear plant process. The proposed method employs an iterative algorithm that prevents confinement to a sole trajectory by fitting a neural network over a series of trajectories that span the desired subset of the state space. At the core of our contributions lie the applicability of our method to open-loop unstable systems and a novel way of generating the system’s reference trajectories, which aim at sufficiently stimulating the underlying dynamics. Following this, the prescribed performance control (PPC) technique is utilized to ensure accurate tracking of the aforementioned trajectories. The effectiveness of our approach is showcased through successful identification of the dynamics of a two-degree of freedom (DOF) robotic manipulator in both a simulation study and a real-life experiment.
更多查看译文
关键词
system identification,non-affine nonlinear systems,artificial neural networks,prescribed performance control,persistency of excitation
AI 理解论文
溯源树
样例
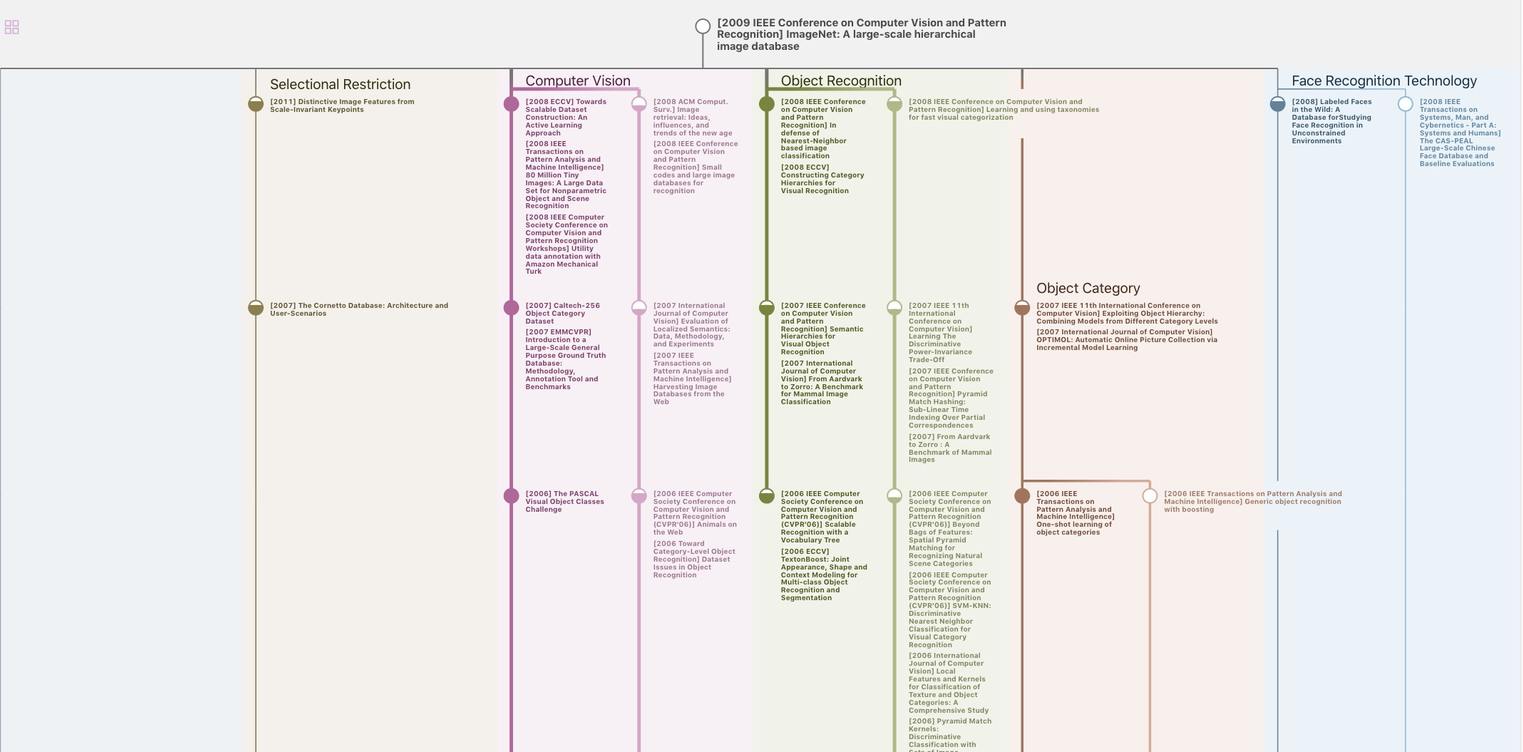
生成溯源树,研究论文发展脉络
Chat Paper
正在生成论文摘要