MAF-Net: A multi-attention fusion network for power transmission line extraction from aerial images
Expert Systems with Applications(2024)
摘要
Automatic extraction of power transmission lines from remote sensing images is important for intelligent power inspection. However, this task faces many challenges, such as complex backgrounds and varying illumination, which make accurate segmentation difficult. Additionally, the severe class imbalance issue arising from the smaller pixel proportion of transmission lines compared to background makes segmentation even more challenging. To address these challenges, a multi-attention fusion network, named MAF-Net, is put forward to achieve accurate end-to-end segmentation of remote sensing images of transmission lines. Firstly, aimed at the inadequate process of local features, a multi-scale context fusion (MCF) block is put forward for effective feature aggregation of multi-scale features. Meanwhile, a dense block is also introduced for feature enhancement to acquire multi-scale contexts. Secondly, considering the pooling operation will bring the problem of information loss, a three-branch pooling (TP) block is proposed to take the best of the advantages of different pooling operations. In addition, the multi-scale input unit is also proposed to support deep layers for extracting the discriminative context information. Furthermore, to tackle the class imbalance issue, a multi-attention block (MA) is used to optimize the simple skip connections to fully aggregate spatial attention, pixel attention, and channel attention features, allowing this segmentation model to focus on the key areas containing power transmission lines. Comparison experiments and ablation experiments are carried out on the remote aerial sensing images for performance analysis. Experiments indicate that proposed MAF-Net could achieve aa superior segmentation ability.
更多查看译文
关键词
Power transmission line detection,Deep network,U-Net,Feature aggregation
AI 理解论文
溯源树
样例
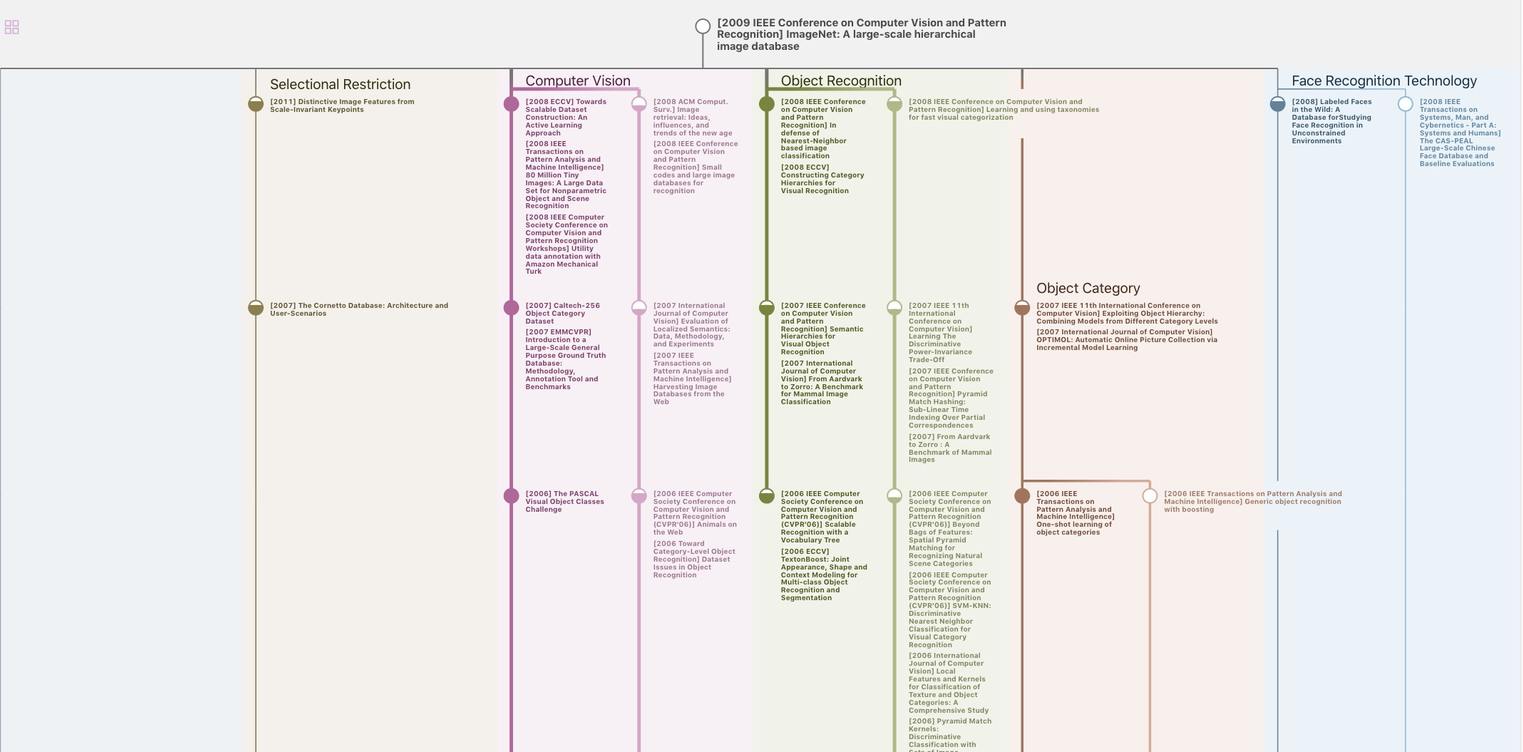
生成溯源树,研究论文发展脉络
Chat Paper
正在生成论文摘要