Assessment of explainable tree-based ensemble algorithms for the enhancement of Copernicus digital elevation model in agricultural lands
INTERNATIONAL JOURNAL OF IMAGE AND DATA FUSION(2024)
摘要
There has been a rapid evolution of tree-based ensemble algorithms which have outperformed deep learning in several studies, thus emerging as a competitive solution for many applications. In this study, ten tree-based ensemble algorithms (random forest, bagging meta-estimator, adaptive boosting (AdaBoost), gradient boosting machine (GBM), extreme gradient boosting (XGBoost), light gradient boosting (LightGBM), histogram-based GBM, categorical boosting (CatBoost), natural gradient boosting (NGBoost), and the regularised greedy forest (RGF)) were comparatively evaluated for the enhancement of Copernicus digital elevation model (DEM) in an agricultural landscape. The enhancement methodology combines elevation and terrain parameters alignment, with feature-level fusion into a DEM enhancement workflow. The training dataset is comprised of eight DEM-derived predictor variables, and the target variable (elevation error). In terms of root mean square error (RMSE) reduction, the best enhancements were achieved by GBM, random forest and the regularised greedy forest at the first, second and third implementation sites respectively. The computational time for training LightGBM was nearly five-hundred times faster than NGBoost, and the speed of LightGBM was closely matched by the histogram-based GBM. Our results provide a knowledge base for other researchers to focus their optimisation strategies on the most promising algorithms.
更多查看译文
关键词
Copernicus,global digital elevation model,LiDAR,machine learning,tree-based ensembles,bagging,boosting,gradient boosting,explainability,partial dependence
AI 理解论文
溯源树
样例
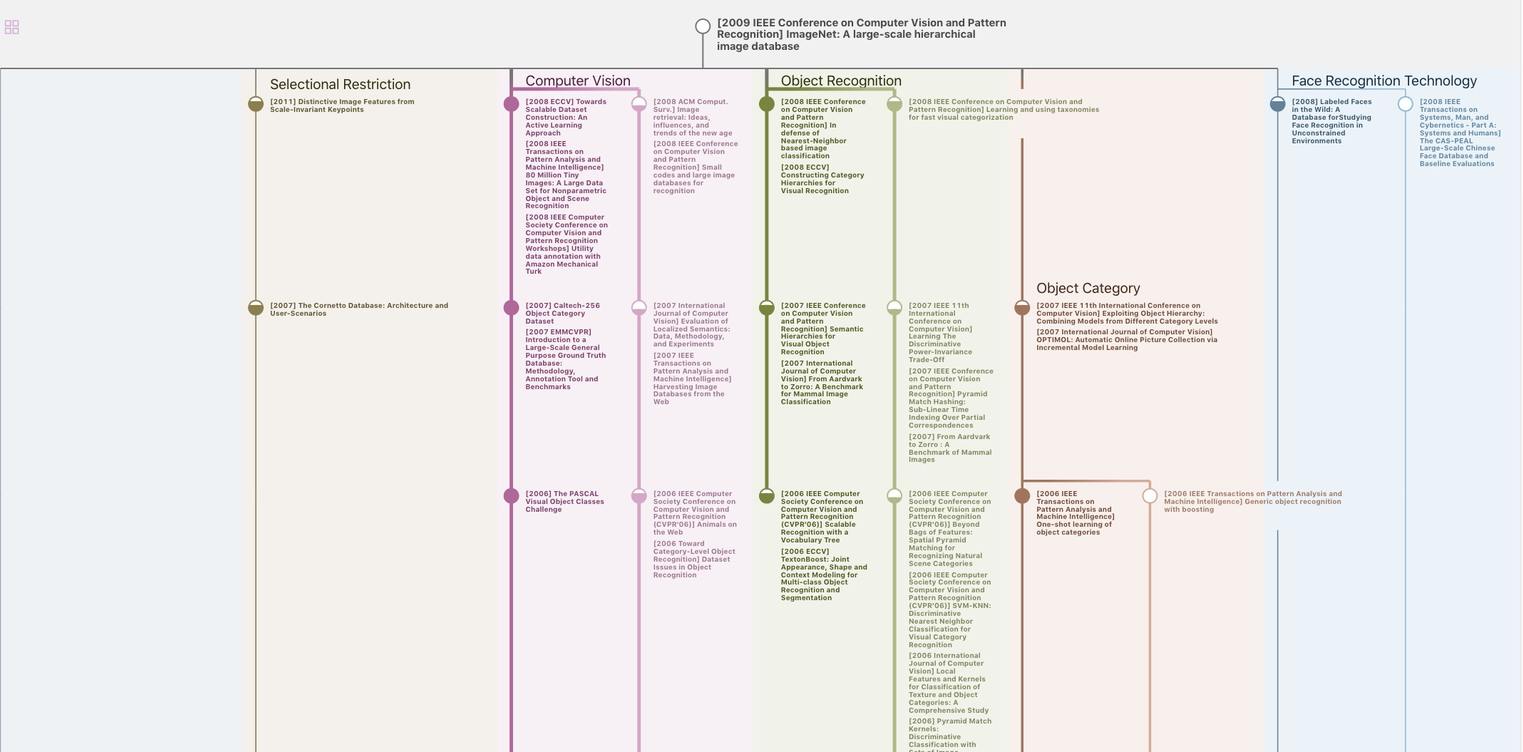
生成溯源树,研究论文发展脉络
Chat Paper
正在生成论文摘要