MTKSVCR: A novel multi-task multi-class support vector machine with safe acceleration rule
Neural Networks(2024)
摘要
Regularized multi-task learning (RMTL) has shown good performance in tackling multi-task binary problems. Although RMTL can be used to handle multi-class problems based on “one-versus-one” and “one-versus-rest” techniques, the information of the samples is not fully utilized and the class imbalance problem occurs. Motivated by the regularization technique in RMTL, we propose an original multi-task multi-class model termed MTKSVCR based on “one-versus-one-versus-rest” strategy to achieve better testing accuracy. Due to the utilization of the idea of RMTL, the related information included in multiple tasks is mined by setting different penalty parameters before task-common and task-specific regularization terms. However, the proposed MTKSVCR is time-consuming since it employs all samples in each optimization problem. Therefore, a multi-parameter safe acceleration rule termed SA is further presented to reduce the time consumption. It identifies and deletes most of the superfluous samples corresponding to 0 elements in the dual optimal solution before solving. Then, only a reduced dual problem is to be solved and the computational efficiency is improved accordingly. The biggest advantage of the proposed SA lies in safety. Namely, it derives an identical optimal solution to the primal problem without SA. In addition, our method remains effective when multiple parameters change simultaneously. Experiments on different artificial datasets and benchmark datasets verify the validity of the proposed methods.
更多查看译文
关键词
Multi-task,Multi-class,Safe screening,Support vector machine,Speedup
AI 理解论文
溯源树
样例
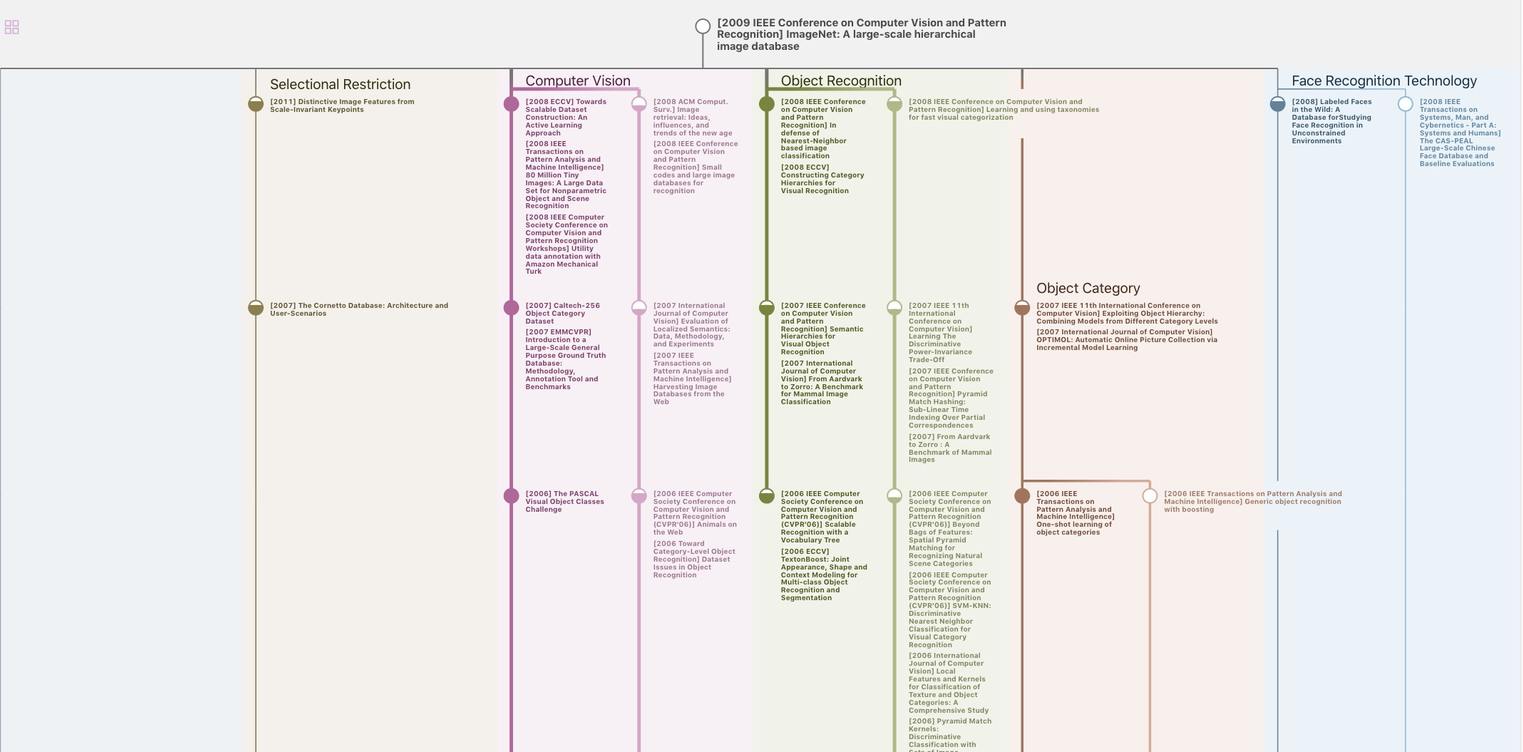
生成溯源树,研究论文发展脉络
Chat Paper
正在生成论文摘要