Identification of Drug-Disease Associations Using a Random Walk with Restart Method and Supervised Learning.
Computational and mathematical methods in medicine(2022)
摘要
Drug-disease correlations play an important role in revealing the mechanism of disease, finding new indications of available drugs, or drug repositioning. A variety of computational approaches were proposed to find drug-disease correlations and achieve good performances. However, these methods used a variety of network information, but integrated networks were rarely used. In addition, the role of known drug-disease association data has not been fully played. In this work, we designed a combination algorithm of random walk and supervised learning to find the drug-disease correlations. We used an integrated network to update the model and selected a gene set as the start of random walk based on the known drug-disease correlations data. The experimental results show that the proposed method can effectively find the correlation between drugs and diseases, and the prediction accuracy is 82.7%. We found that there are 8 pairs of drug-disease relationships that have not yet been reported, and 5 of them have pharmacodynamic effects on Parkinson's disease. We also found that a key linkage between Parkinson's disease and phenylhexol, a drug for the treatment of Parkinson's disease -synuclein and tau protein, provides a useful exploration for the effectiveness of the treatment of Parkinson's disease.
更多查看译文
关键词
random walk,associations,learning,drug-disease
AI 理解论文
溯源树
样例
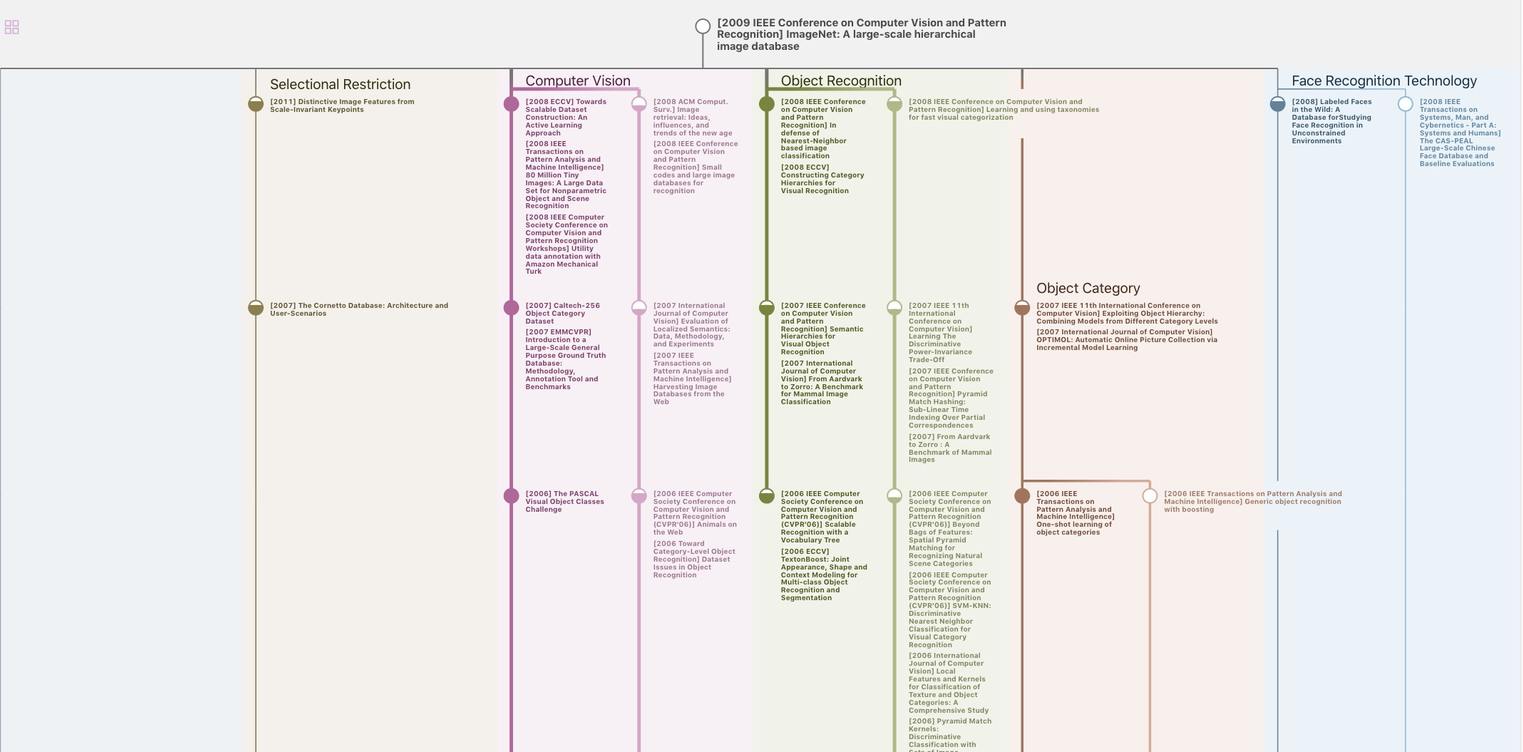
生成溯源树,研究论文发展脉络
Chat Paper
正在生成论文摘要