A Multi-attention Based CNN-BiLSTM Intrusion Detection Model for In-vehicle Networks.
Parallel and Distributed Processing with Applications(2023)
摘要
The development of vehicular networking technology continuously enhances the internet connectivity of modern vehicles. However, for in-vehicle networks, constant communication with the outside world dramatically increases vehicle security risks, posing serious security threats to automotive systems and occupants. This paper proposes a CNN-BiLSTM vehicular network intrusion detection model based on multi-head attention by analyzing the information security vulnerabilities in the Controller Area Network (CAN) bus. By extracting only the communication features of CAN messages, including CAN ID and Data field, this paper conducted a multiclass classification study on the real-time traffic dataset CarHacking. The model achieved a detection accuracy of 99.992%. The reduction of features effectively simplifies the model and improves its computation efficiency.
更多查看译文
关键词
deep learning,control area network,anomaly detection,in-vehicle network
AI 理解论文
溯源树
样例
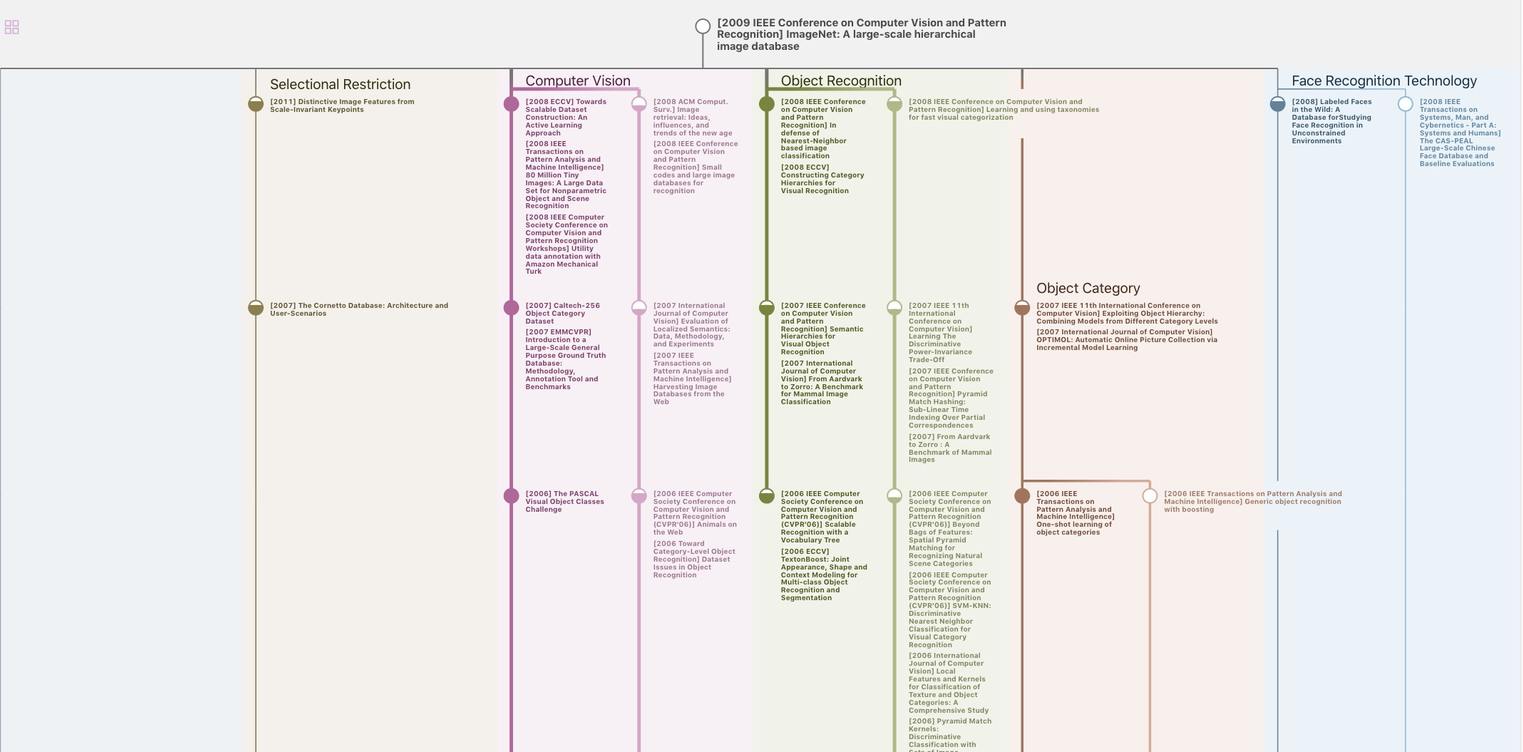
生成溯源树,研究论文发展脉络
Chat Paper
正在生成论文摘要