Context-aware Video Anomaly Detection in Long-Term Datasets
arxiv(2024)
摘要
Video anomaly detection research is generally evaluated on short, isolated
benchmark videos only a few minutes long. However, in real-world environments,
security cameras observe the same scene for months or years at a time, and the
notion of anomalous behavior critically depends on context, such as the time of
day, day of week, or schedule of events. Here, we propose a context-aware video
anomaly detection algorithm, Trinity, specifically targeted to these scenarios.
Trinity is especially well-suited to crowded scenes in which individuals cannot
be easily tracked, and anomalies are due to speed, direction, or absence of
group motion. Trinity is a contrastive learning framework that aims to learn
alignments between context, appearance, and motion, and uses alignment quality
to classify videos as normal or anomalous. We evaluate our algorithm on both
conventional benchmarks and a public webcam-based dataset we collected that
spans more than three months of activity.
更多查看译文
AI 理解论文
溯源树
样例
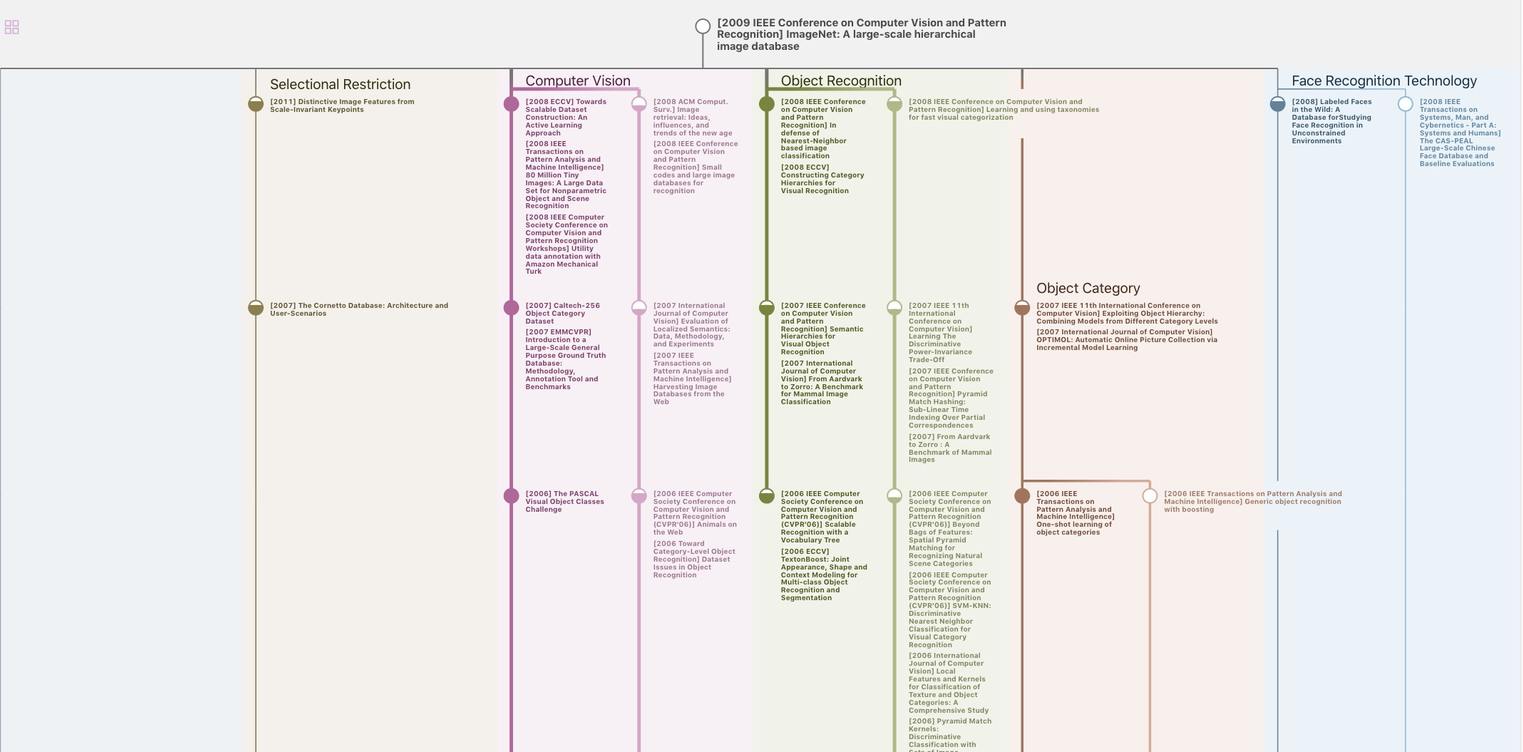
生成溯源树,研究论文发展脉络
Chat Paper
正在生成论文摘要