1-bit Quantized On-chip Hybrid Diffraction Neural Network Enabled by Authentic All-optical Fully-connected Architecture
arxiv(2024)
摘要
Optical Diffraction Neural Networks (DNNs), a subset of Optical Neural
Networks (ONNs), show promise in mirroring the prowess of electronic networks.
This study introduces the Hybrid Diffraction Neural Network (HDNN), a novel
architecture that incorporates matrix multiplication into DNNs, synergizing the
benefits of conventional ONNs with those of DNNs to surmount the modulation
limitations inherent in optical diffraction neural networks. Utilizing a
singular phase modulation layer and an amplitude modulation layer, the trained
neural network demonstrated remarkable accuracies of 96.39
recognition tasks in simulation and experiment, respectively. Additionally, we
develop the Binning Design (BD) method, which effectively mitigates the
constraints imposed by sampling intervals on diffraction units, substantially
streamlining experimental procedures. Furthermore, we propose an on-chip HDNN
that not only employs a beam-splitting phase modulation layer for enhanced
integration level but also significantly relaxes device fabrication
requirements, replacing metasurfaces with relief surfaces designed by 1-bit
quantization. Besides, we conceptualized an all-optical HDNN-assisted lesion
detection network, achieving detection outcomes that were 100
simulation predictions. This work not only advances the performance of DNNs but
also streamlines the path towards industrial optical neural network production.
更多查看译文
AI 理解论文
溯源树
样例
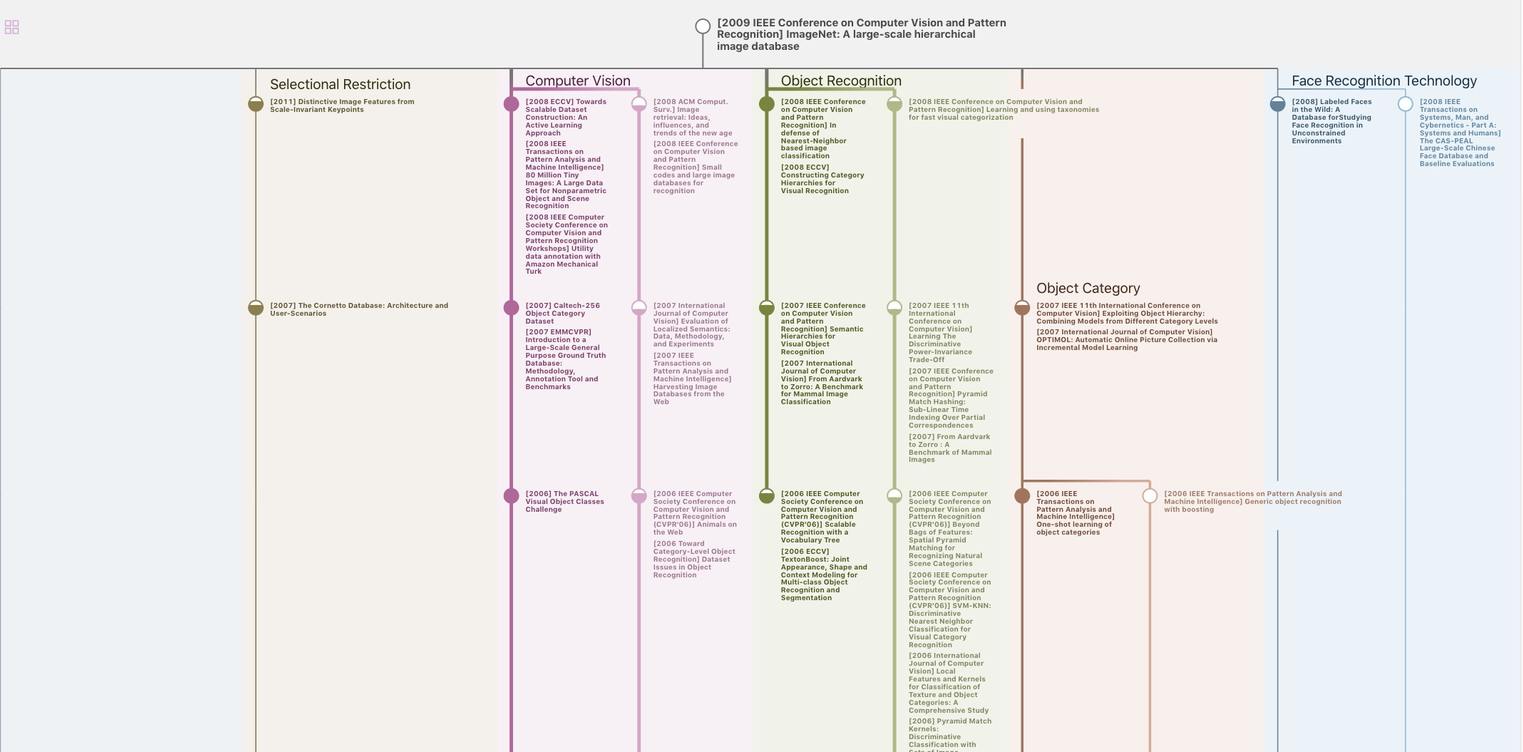
生成溯源树,研究论文发展脉络
Chat Paper
正在生成论文摘要