Fine-grained IoT device identification method based on self-supervised ViT.
Parallel and Distributed Processing with Applications(2023)
摘要
The proliferation of Internet of Things(IoT) technology has introduced challenges in device management and network security due to the heterogeneous nature of IoT devices and the inherent security risks they pose. Achieving timely “visibility” of devices becomes crucial in addressing these challenges. While prevailing research primarily relies on extensive labeled training data, which encounter limitations stemming from the rapid device iteration and cumbersome IoT labeling processes.To overcome these constraints, we propose a novel IoT device identification method based on a self-supervised Vision in Transformer(ViT) framework. This method translates the abstract traffic behaviors into concrete representations. Leveraging the power of self-supervised learning, the proposed approach capitalizes on unlabeled data to enable autonomous self-training. By employing the ViT model, the method effectively extracts salient features from the images, enabling end-to-end, automated, and high-precision device identification.Experimental results show that on real-world dataset, our method achieves 99.01% accuracy for the binary classification task of distinguishing IoT devices. Moreover, it achieves fine-grained identification accuracy for IoT devices of 98.44% with Macro-F1 improving to 98.91%. These results surpass the performance of other existing IoT device identification models, such as RF and CNN.
更多查看译文
关键词
Internet of Things,device identification,deep learning,self-supervised learning,Vision in Transformer
AI 理解论文
溯源树
样例
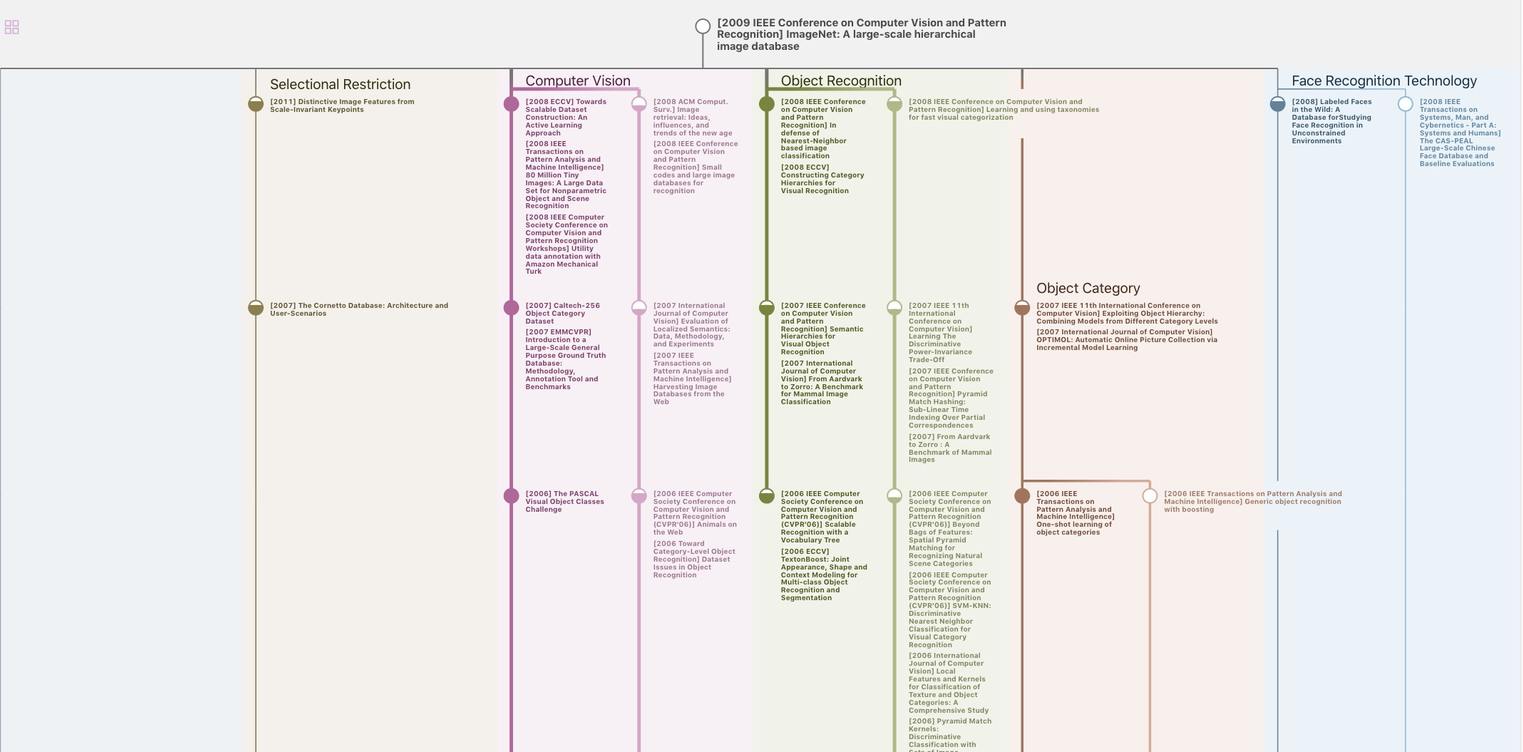
生成溯源树,研究论文发展脉络
Chat Paper
正在生成论文摘要