Multi-object behaviour recognition based on object detection cascaded image classification in classroom scenes
Applied Intelligence(2024)
摘要
For multi-object behaviour recognition in classroom scenes, crowded objects have heavy occlusion, invisible keypoints, scale variation, which directly overwhelms the recognition performance. Due to the dense student objects and similar student behaviours, multi-object behaviour recognition brings great challenges. Therefore, we proposed multi-object behaviour recognition based on object detection cascaded image classification. Specifically, object detection extracts student objects, followed by Vision Transformer (ViT) classification of student behaviour. To ensure the accuracy of behaviour recognition, it is first necessary to improve the detection performance of object detection. This paper proposes the Shallow Auxiliary Module for object detection to assist the backbone network in extracting hybrid multi-scale feature information. The multi-scale and multi-channel feature information is fused to alleviate object overlap and scale variation. We propose a Scale Assignment Fusion Mechanism that non-heuristically guides objects to learn the optimal feature layer. Furthermore, the Anchor-free Dynamic Label Assignment can suppress the prediction of low-quality bounding boxes, stabling training and improving detection performance. The proposed student object detector achieves the state-of-the-art mAP ^50 of 88.03 and AP _l of 57.64, outperforming state-of-the-art object detection methods. Our multi-object behaviour recognition method achieves the recognition of four behaviour classes, which is significantly better than the results of other comparison methods.
更多查看译文
关键词
Classroom scene,Behaviour recognition,Object detection,Image classification,Vision transformer
AI 理解论文
溯源树
样例
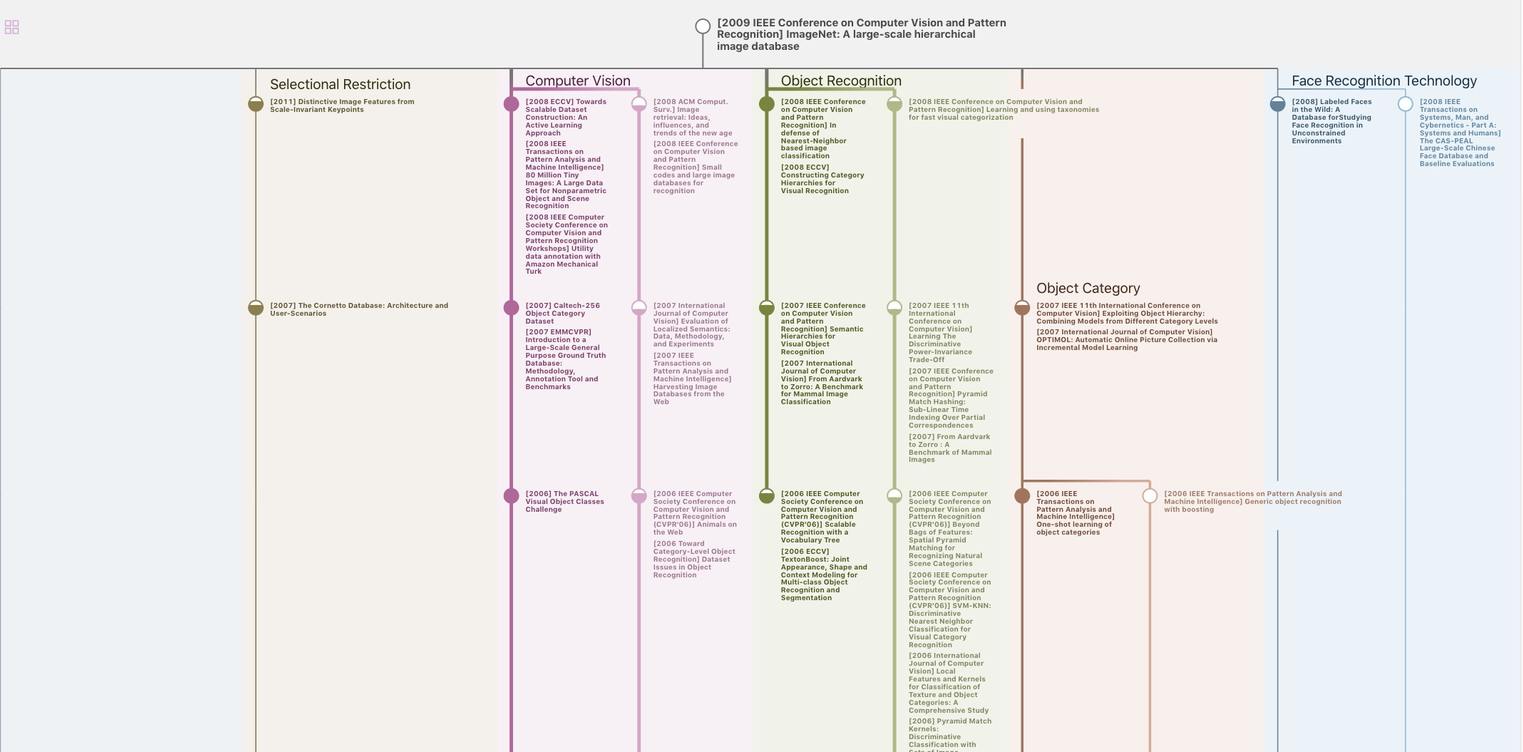
生成溯源树,研究论文发展脉络
Chat Paper
正在生成论文摘要