Doubly stochastic subdomain mining with sample reweighting for unsupervised domain adaptive person re-identification.
AI Commun.(2024)
摘要
Clustering-based unsupervised domain adaptive person re-identification methods have achieved remarkable progress. However, existing works are easy to fall into local minimum traps due to the optimization of two variables, feature representation and pseudo labels. Besides, the model can also be hurt by the inevitable false assignment of pseudo labels. In order to solve these problems, we propose the Doubly Stochastic Subdomain Mining (DSSM) to prevent the nonconvex optimization from falling into local minima in this paper. And we also design a novel reweighting algorithm based on the similarity correlation coefficient between samples which is referred to as Maximal Heterogeneous Similarity (MHS), it can reduce the adverse effect caused by noisy labels. Extensive experiments on two popular person re-identification datasets demonstrate that our method outperforms other state-of-the-art works. The source code is available at https://github.com/Tchunansheng/DSSM .
更多查看译文
关键词
Unsupervised domain adaptation,person re-identification,sample reweighting,feature learning
AI 理解论文
溯源树
样例
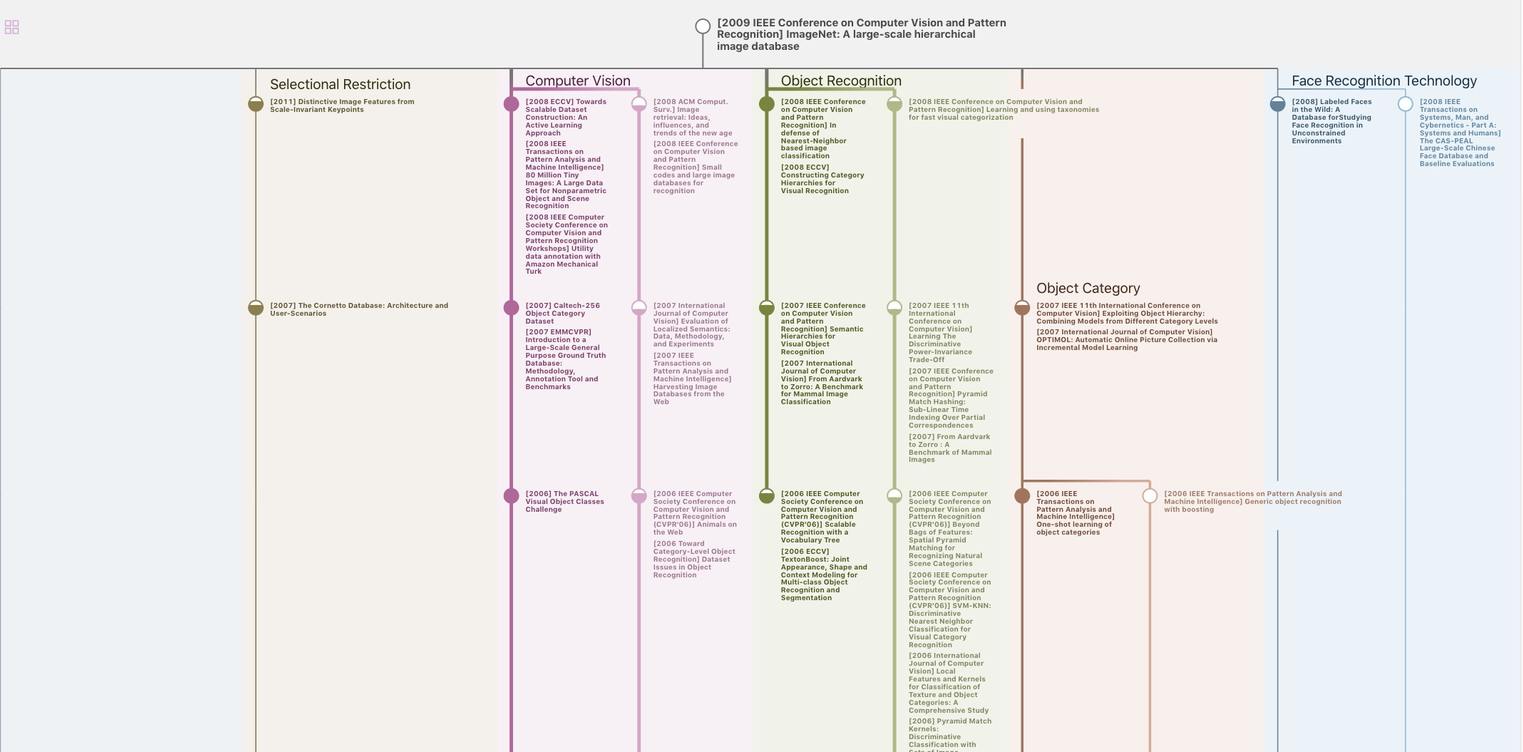
生成溯源树,研究论文发展脉络
Chat Paper
正在生成论文摘要