Frequency-Enhanced Geometric-Constrained Reconstruction for Localizing Myocardial Infarction in 12-Lead Electrocardiograms.
IEEE transactions on bio-medical engineering(2024)
摘要
Determining the location of myocardial infarction is crucial for clinical management and therapeutic stratagem. However, existing diagnostic tools either sacrifice ease of use or are limited by their spatial resolution. Addressing this, we aim to refine myocardial infarction localization via surface potential reconstruction of the ventricles in 12-lead electrocardiograms (ECG). A notable obstacle is the ill-posed nature of such reconstructions. To overcome this, we introduce the frequency-enhanced geometric-constrained iterative network (FGIN). FGIN begins by mining the latent features from ECG data across both time and frequency domains. Subsequently, it increases the data dimensionality of ECG and captures intricate features using convolutional layers. Finally, FGIN incorporates ventricular geometry as a constraint on surface potential distribution. It allocates variable weights to distinct edges. Experimental validation of FGIN confirms its efficacy over synthetic and clinical datasets. On the synthetic dataset, FGIN outperforms seven existing reconstruction methods, attaining the highest Pearson Correlation Coefficient of 0.8624, the lowest Root Mean Square Error of 0.1548, and the highest Structural Similarity Index Measure of 0.7988. On the clinical public dataset (2007 PhysioNet/Computers in Cardiology Challenge), FGIN achieves better localization results than other approaches, according to the clinical standard 17-segment model, achieving an average Segment Overlap of 87.2%. Clinical trials on 50 patients demonstrate FGIN's effectiveness, showing an average accuracy of 91.6% and an average Segment Overlap of 88.2%.
更多查看译文
关键词
Myocardial Infarction,12-Lead ECG,ECG Inverse Problem,Frequency-Enhanced Attention,Geometric Constraint
AI 理解论文
溯源树
样例
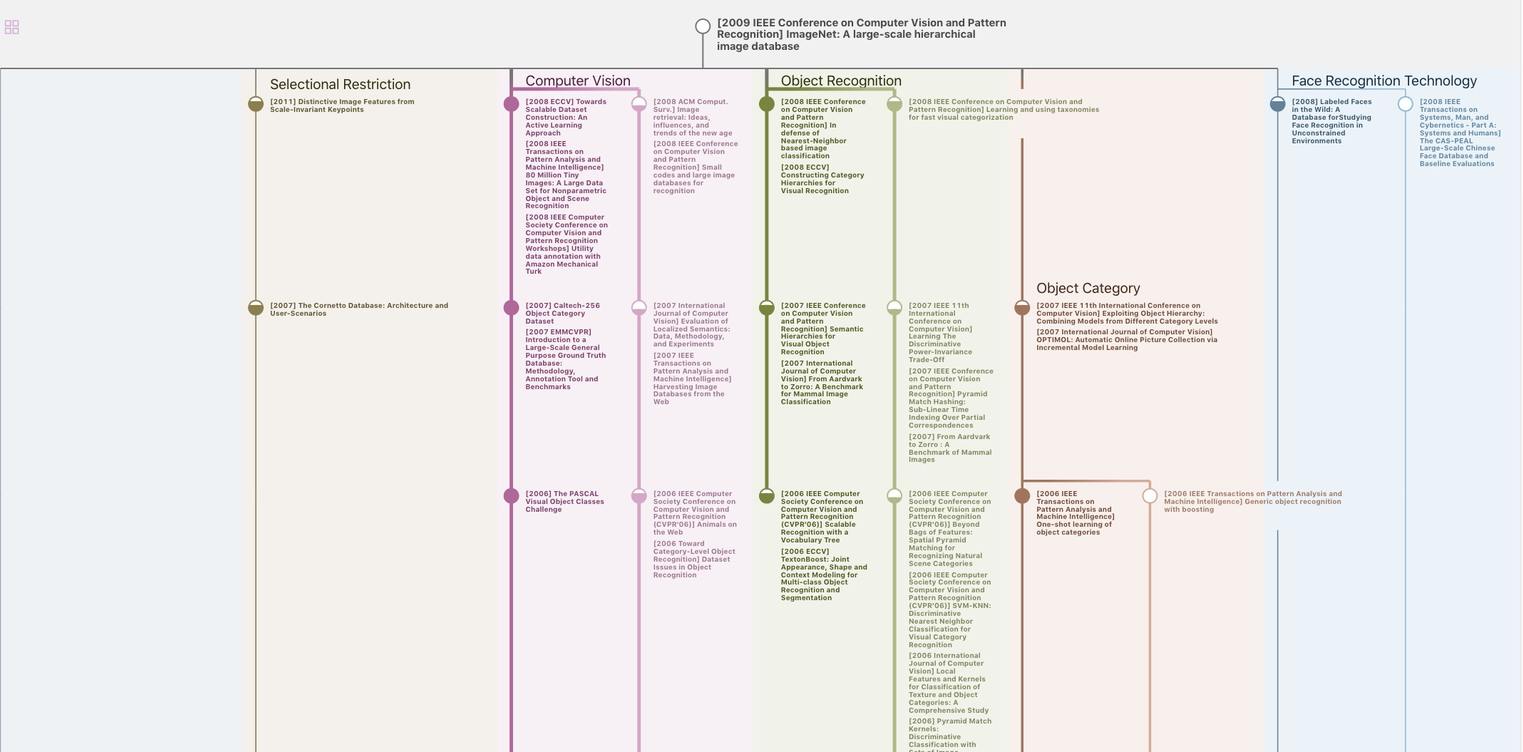
生成溯源树,研究论文发展脉络
Chat Paper
正在生成论文摘要