Neutrons Sensitivity of Deep Reinforcement Learning Policies on EdgeAI Accelerators
IEEE Transactions on Nuclear Science(2024)
摘要
Autonomous robots and their application are becoming popular in several different fields, including tasks where robots closely interact with humans. Therefore, the reliability of computation must be paramount. In this work, we measure the reliability of Google’s Coral Edge TPU executing three Deep Reinforcement Learning (DRL) models through an accelerated neutrons beam. We experimentally collect data that, when scaled to the natural neutron flux, accounts for more than 5 million years. Based on our extensive evaluation, we quantify and qualify the radiation-induced corruption on the correctness of DRL. Crucially, our data shows that the Edge TPU executing DRL has an error rate that is up to 18 times higher the limit imposed by international reliability standards. We found that, despite the feedback and intrinsic redundancy of DRL, the propagation of the fault induces the model to fail in the vast majority of cases or the model manages to finish but reports wrong metrics (i.e. speed, final position, reward). We provide insights on how radiation corrupts the model, on how the fault propagates in the computation, and about the failure characteristic of the controlled robot.
更多查看译文
AI 理解论文
溯源树
样例
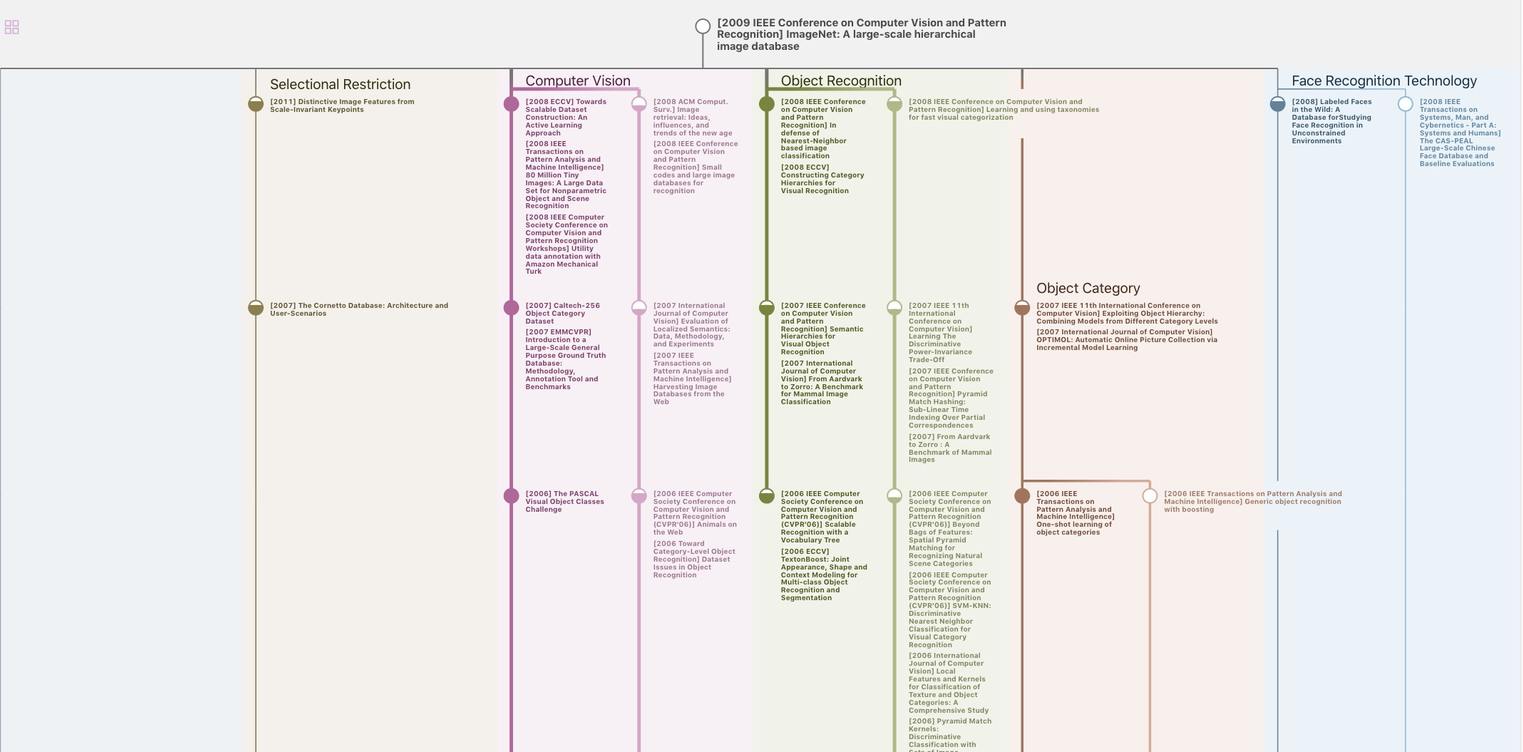
生成溯源树,研究论文发展脉络
Chat Paper
正在生成论文摘要