A deep learning-based approach for automated two-stage femur segmentation
crossref(2024)
摘要
Abstract Objectives The purpose of this study was to develop a deep learning-based two-stage network based on deep learning tenets designed for swift delineation of regions of interest (ROIs) and semantic segmentation of the femur with accuracy and precision. Methods The deep learning-based two-stage segmentation network proposed in this paper performs femur segmentation on full lower limb CT images. The experimental dataset utilized herein is sourced from Hospital, encompassing a total of 100 experimental datasets—partitioned into 85 datasets for training, 8 for validation, and 7 for testing. Results In the first stage, the average IoU reached 0.9671, and the mAP reached 0.9656, indicating that the femoral region was effectively and accurately extracted. In the second stage, the average Dice coefficient, sensitivity, specificity, and pixel accuracy were 0.953, 0.965, 0.998, and 0.996, respectively, indicating accurate segmentation of the femur. Compared with the non-two-stage network, it converges more easily during training, has a shorter inference time, has higher accuracy, and exhibits superior performance. Conclusion This deep learning-based, two-stage segmentation network is adept at executing femur segmentation tasks with remarkable speed, precision, and autonomy across expansive medical datasets. Boasting minimal computational requisites. With low computational constraints, it has broad applicability and scalability. It holds promise as an effective tool for improving femur image segmentation and facilitating clinical diagnosis.
更多查看译文
AI 理解论文
溯源树
样例
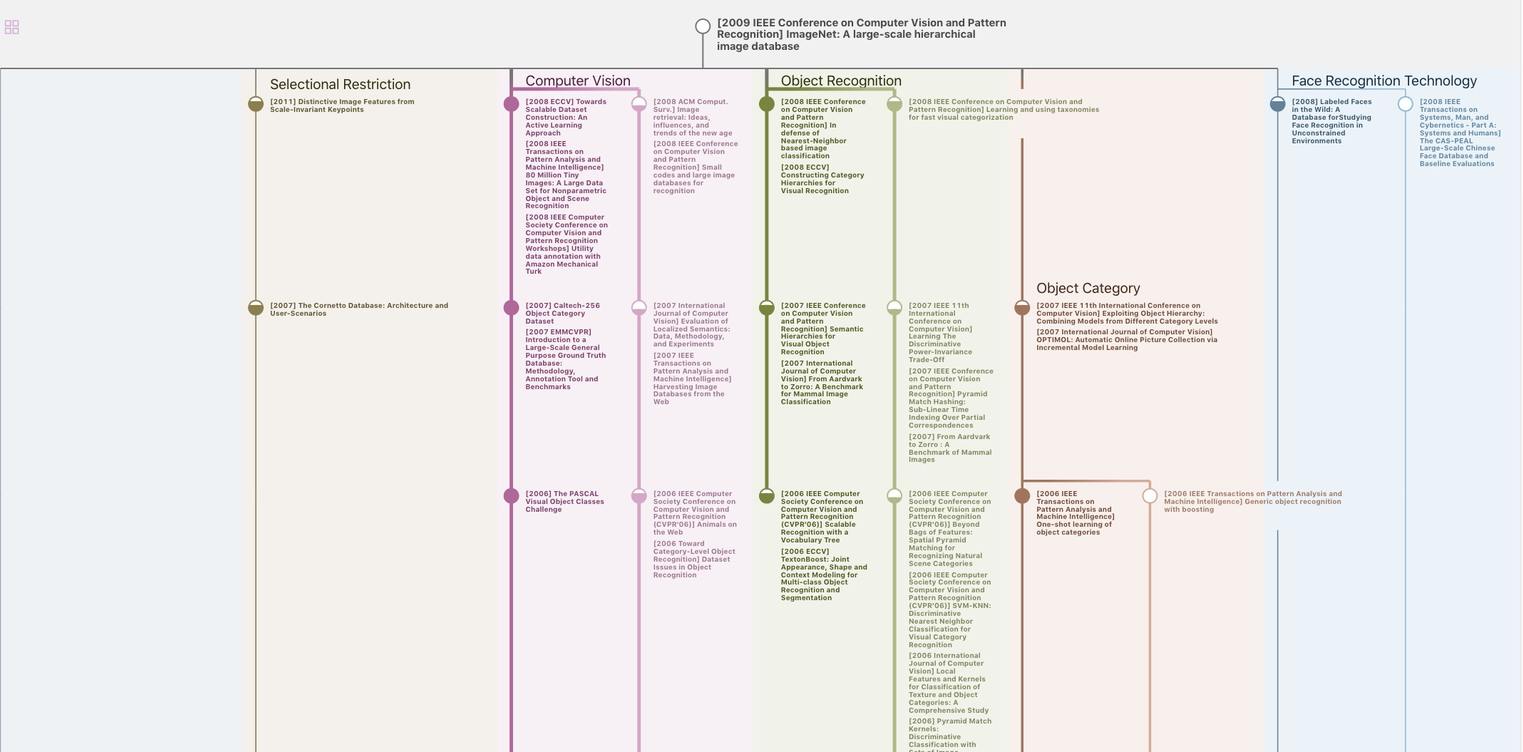
生成溯源树,研究论文发展脉络
Chat Paper
正在生成论文摘要