Calculating the High-Latitude Ionospheric Electrodynamics Using a Machine Learning-Based Field-Aligned Current Model
SPACE WEATHER-THE INTERNATIONAL JOURNAL OF RESEARCH AND APPLICATIONS(2024)
摘要
We introduce a new framework called Machine Learning (ML) based Auroral Ionospheric electrodynamics Model (ML-AIM). ML-AIM solves a current continuity equation by utilizing the ML model of Field Aligned Currents of Kunduri et al. (2020, ), the FAC-derived auroral conductance model of Robinson et al. (2020, ), and the solar irradiance conductance model of Moen and Brekke (1993, ). The ML-AIM inputs are 60-min time histories of solar wind plasma, interplanetary magnetic fields (IMF), and geomagnetic indices, and its outputs are ionospheric electric potential, electric fields, Pedersen/Hall currents, and Joule Heating. We conduct two ML-AIM simulations for a weak geomagnetic activity interval on 14 May 2013 and a geomagnetic storm on 7-8 September 2017. ML-AIM produces physically accurate ionospheric potential patterns such as the two-cell convection pattern and the enhancement of electric potentials during active times. The cross polar cap potentials (phi PC) from ML-AIM, the Weimer (2005, ) model, and the Super Dual Auroral Radar Network (SuperDARN) data-assimilated potentials, are compared to the ones from 3204 polar crossings of the Defense Meteorological Satellite Program F17 satellite, showing better performance of ML-AIM than others. ML-AIM is unique and innovative because it predicts ionospheric responses to the time-varying solar wind and geomagnetic conditions, while the other traditional empirical models like Weimer (2005, ) designed to provide a quasi-static ionospheric condition under quasi-steady solar wind/IMF conditions. Plans are underway to improve ML-AIM performance by including a fully ML network of models of aurora precipitation and ionospheric conductance, targeting its characterization of geomagnetically active times. Auroral ionospheric electrodynamics, originating from the interaction of solar wind with Earth's magnetosphere-ionosphere system, is vital in space weather forecasting, as it influences satellite communication, satellite drag, navigation, and power distribution systems on Earth. Machine learning (ML), a subset of artificial intelligence, has demonstrated considerable potential within the realm of space weather research, yielding promising outcomes for forecasting operations. In this work, we have developed a new ML-based auroral ionospheric electrodynamics model (ML-AIM) by extending an existing ML-based model for ionospheric currents. ML-AIM uses 60-min time history of solar and geomagnetic input conditions to nowcast the ionospheric electrodynamics. The present model is capable of better representing the ionospheric response to the time-varying solar input conditions. This work laid a successful groundwork for integrating various advanced ML models within a single framework to accurately model the space weather relevant quantities. ML-AIM predicts high-latitude ionospheric electrodynamics based on 1-hr time histories of SW/IMF conditions and geomagnetic indices ML-AIM performs better in predicting cross polar cap potentials than the Weimer (2005) and SuperDARN data-assimilated models ML-AIM considers gradual ionospheric responses to consistently varying SW/IMF conditions, unlike traditional empirical models
更多查看译文
关键词
auroral electrodynamics,magnetosphere-ionosphere coupling,cross polar cap potential,field aligned currents,auroral conductance,machine learning
AI 理解论文
溯源树
样例
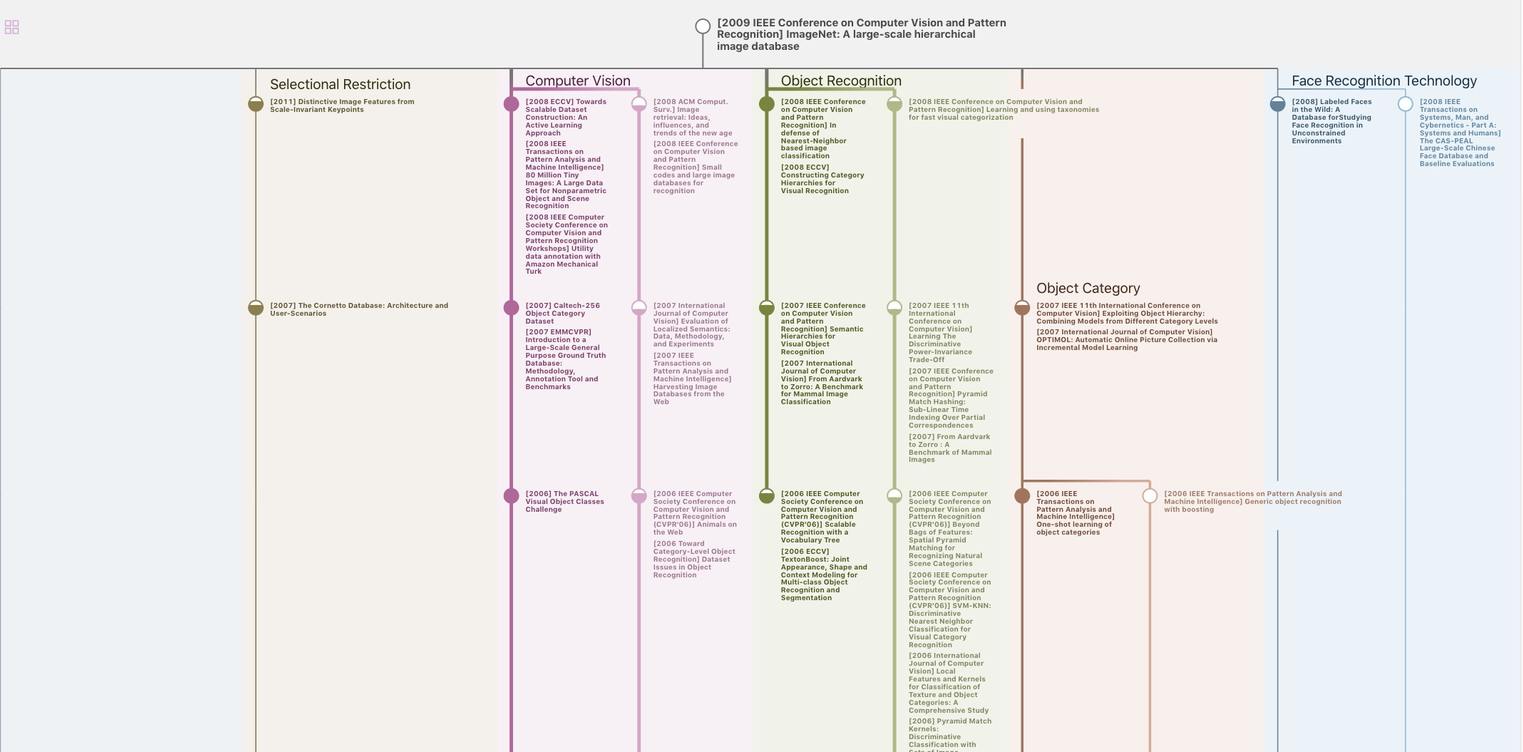
生成溯源树,研究论文发展脉络
Chat Paper
正在生成论文摘要