Multiple adaptive factors based interacting multiple model estimator
IET CONTROL THEORY AND APPLICATIONS(2024)
摘要
In the field of optoelectronic tracking, precisely modeling the motion equations of the tracked target is often challenging, and in some cases, they may even be entirely unknown. This necessitates the use of a robust state estimator for accurate state estimation. Additionally, atmospheric turbulence, variations in illumination, and intricate observation backgrounds may introduce a significant increase in observation noise for the tracked target. To address these challenges, one approach is to introduce adaptive factors, such as the Mahalanobis method, into the robust state estimator to enhance estimation accuracy. However, further exploration has revealed that adaptive factors designed using different methods offer unique advantages in scenarios with varying levels of noise amplification. In this paper, different adaptive factors are further combined using an interacting multiple model approach, allowing the designed state estimator to exhibit stronger adaptability to noise amplification. The stability and effectiveness of this algorithm are validated through program simulations, double reflection mirror experiment, and drone trace prediction, demonstrating its applicability and reliability in diverse scenarios. Challenges related to inaccuracies in the motion model and the sudden surge in observation noise pose significant issues in target tracking and state estimation. In response, this paper proposed an estimator that utilizes the Interacting Multiple Model (IMM) approach to integrate robust state estimators with various adaptive factors, ultimately enhancing estimation performance. image
更多查看译文
关键词
adaptive filters,noise,state estimation,tracking
AI 理解论文
溯源树
样例
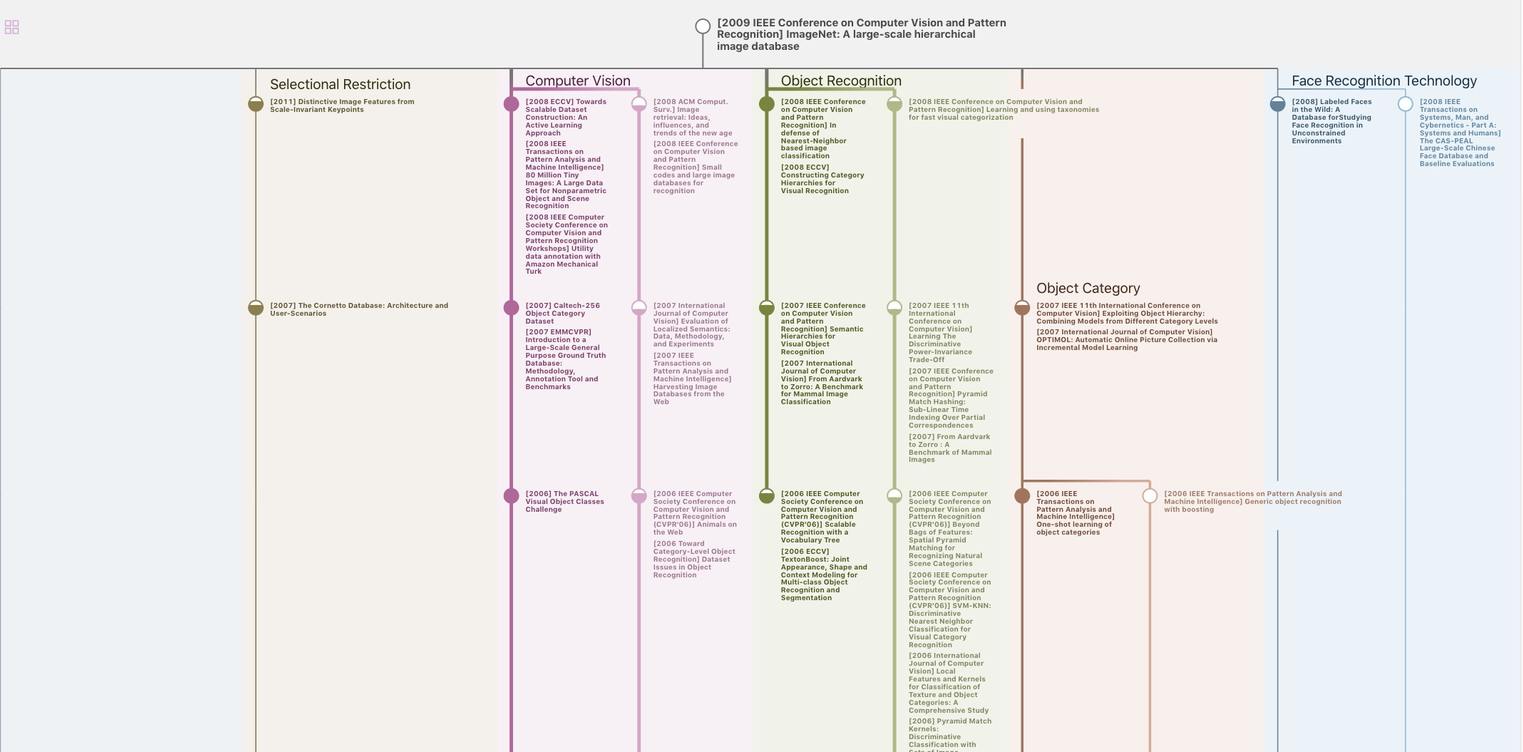
生成溯源树,研究论文发展脉络
Chat Paper
正在生成论文摘要