Extracting Clean and Balanced Subset for Noisy Long-tailed Classification
arxiv(2024)
摘要
Real-world datasets usually are class-imbalanced and corrupted by label
noise. To solve the joint issue of long-tailed distribution and label noise,
most previous works usually aim to design a noise detector to distinguish the
noisy and clean samples. Despite their effectiveness, they may be limited in
handling the joint issue effectively in a unified way. In this work, we develop
a novel pseudo labeling method using class prototypes from the perspective of
distribution matching, which can be solved with optimal transport (OT). By
setting a manually-specific probability measure and using a learned transport
plan to pseudo-label the training samples, the proposed method can reduce the
side-effects of noisy and long-tailed data simultaneously. Then we introduce a
simple yet effective filter criteria by combining the observed labels and
pseudo labels to obtain a more balanced and less noisy subset for a robust
model training. Extensive experiments demonstrate that our method can extract
this class-balanced subset with clean labels, which brings effective
performance gains for long-tailed classification with label noise.
更多查看译文
AI 理解论文
溯源树
样例
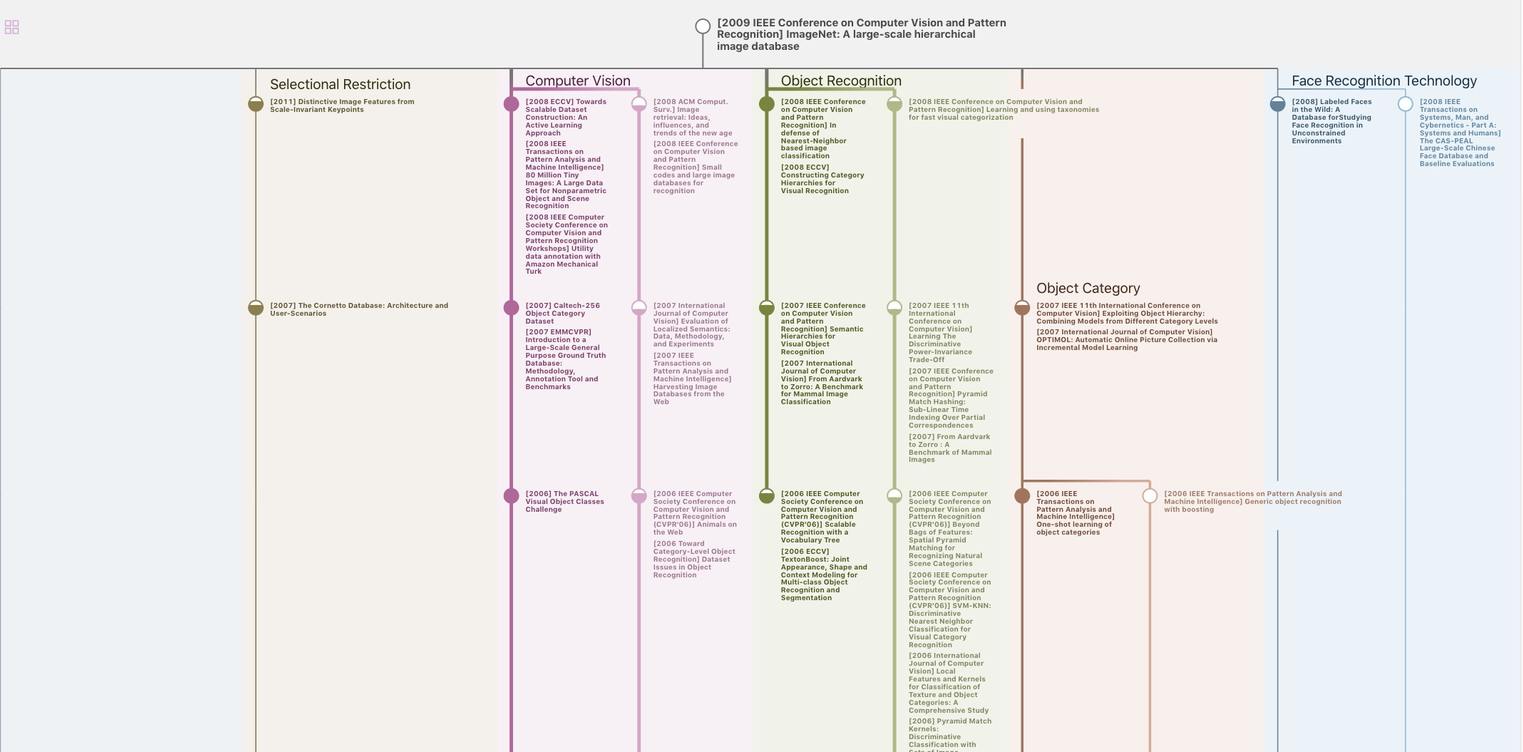
生成溯源树,研究论文发展脉络
Chat Paper
正在生成论文摘要