Onboard Processing of Hyperspectral Imagery: Deep Learning Advancements, Methodologies, Challenges, and Emerging Trends
arxiv(2024)
摘要
Recent advancements in deep learning techniques have spurred considerable
interest in their application to hyperspectral imagery processing. This paper
provides a comprehensive review of the latest developments in this field,
focusing on methodologies, challenges, and emerging trends. Deep learning
architectures such as Convolutional Neural Networks (CNNs), Autoencoders, Deep
Belief Networks (DBNs), Generative Adversarial Networks (GANs), and Recurrent
Neural Networks (RNNs) are examined for their suitability in processing
hyperspectral data. Key challenges, including limited training data and
computational constraints, are identified, along with strategies such as data
augmentation and noise reduction using GANs. The paper discusses the efficacy
of different network architectures, highlighting the advantages of lightweight
CNN models and 1D CNNs for onboard processing. Moreover, the potential of
hardware accelerators, particularly Field Programmable Gate Arrays (FPGAs), for
enhancing processing efficiency is explored. The review concludes with insights
into ongoing research trends, including the integration of deep learning
techniques into Earth observation missions such as the CHIME mission, and
emphasizes the need for further exploration and refinement of deep learning
methodologies to address the evolving demands of hyperspectral image
processing.
更多查看译文
AI 理解论文
溯源树
样例
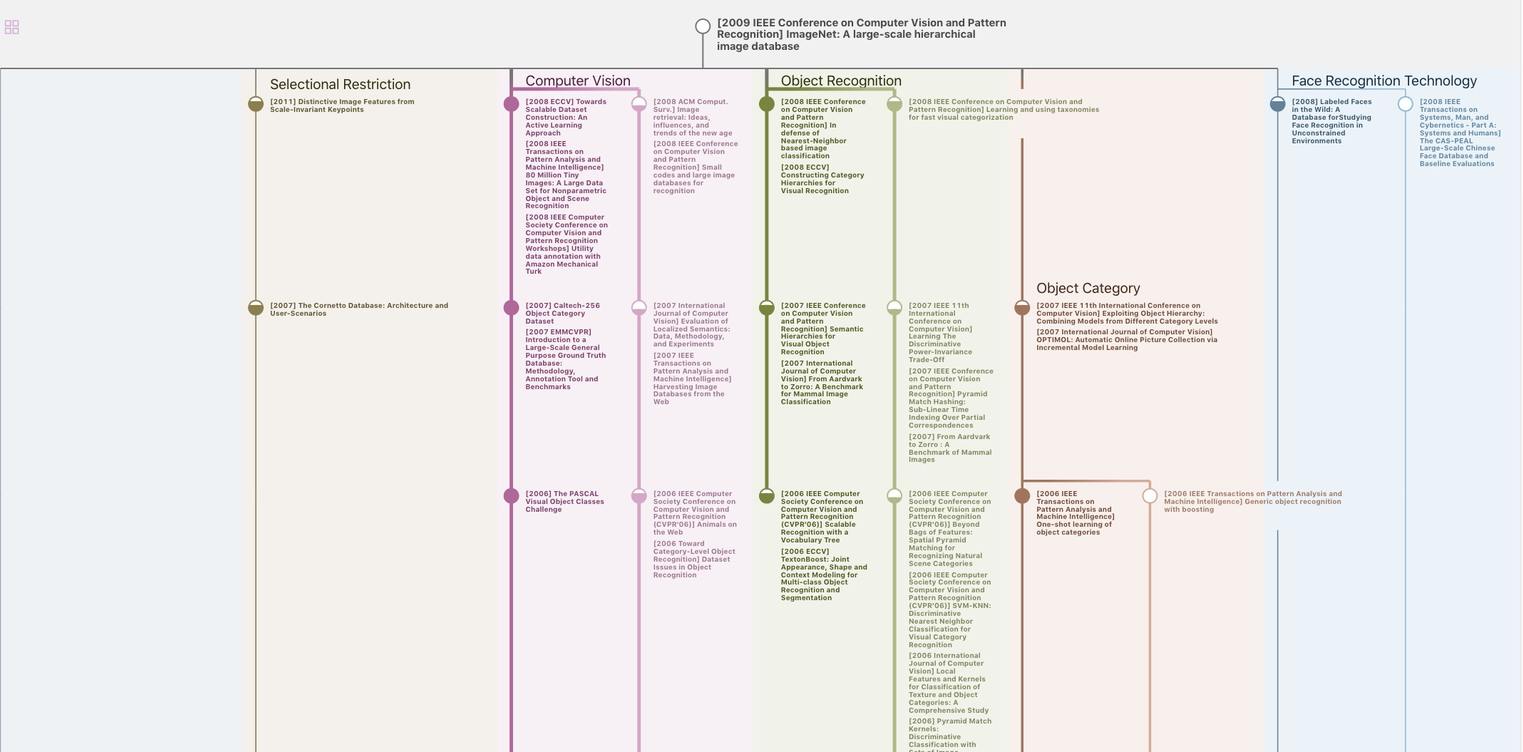
生成溯源树,研究论文发展脉络
Chat Paper
正在生成论文摘要