Community detection in attributed networks using neighborhood information
Cluster Computing(2024)
摘要
Community detection is a crucial aspect in network analysis. Real-world networks are often enriched with attributes providing extensive information for nodes beyond mere topology. Integrating these nodal attributes into community detection for attributed networks poses notable challenges and remains an active research field. In this paper, we propose a novel method that incorporates structural information into fused attributes. This is achieved by defining a fusion similarity between nodes, which is a convex combination of topology similarity, pairwise attribute similarity, and attribute similarity with their immediate neighbors. One advantage of the proposed method is its flexibility in identifying communities in disassortative networks, where nodes exhibit more connections between different groups than within their own group. We employ an iterative spectral clustering technique to discover communities and assess the influence of various attributes within these communities. Our experimental results validate the effectiveness of this approach, demonstrating its utility in leveraging node attributes in diverse simulated and real-world network datasets.
更多查看译文
关键词
Community detection,Disassortative stochastic block model,Spectral clustering,Fusion attribute similarity
AI 理解论文
溯源树
样例
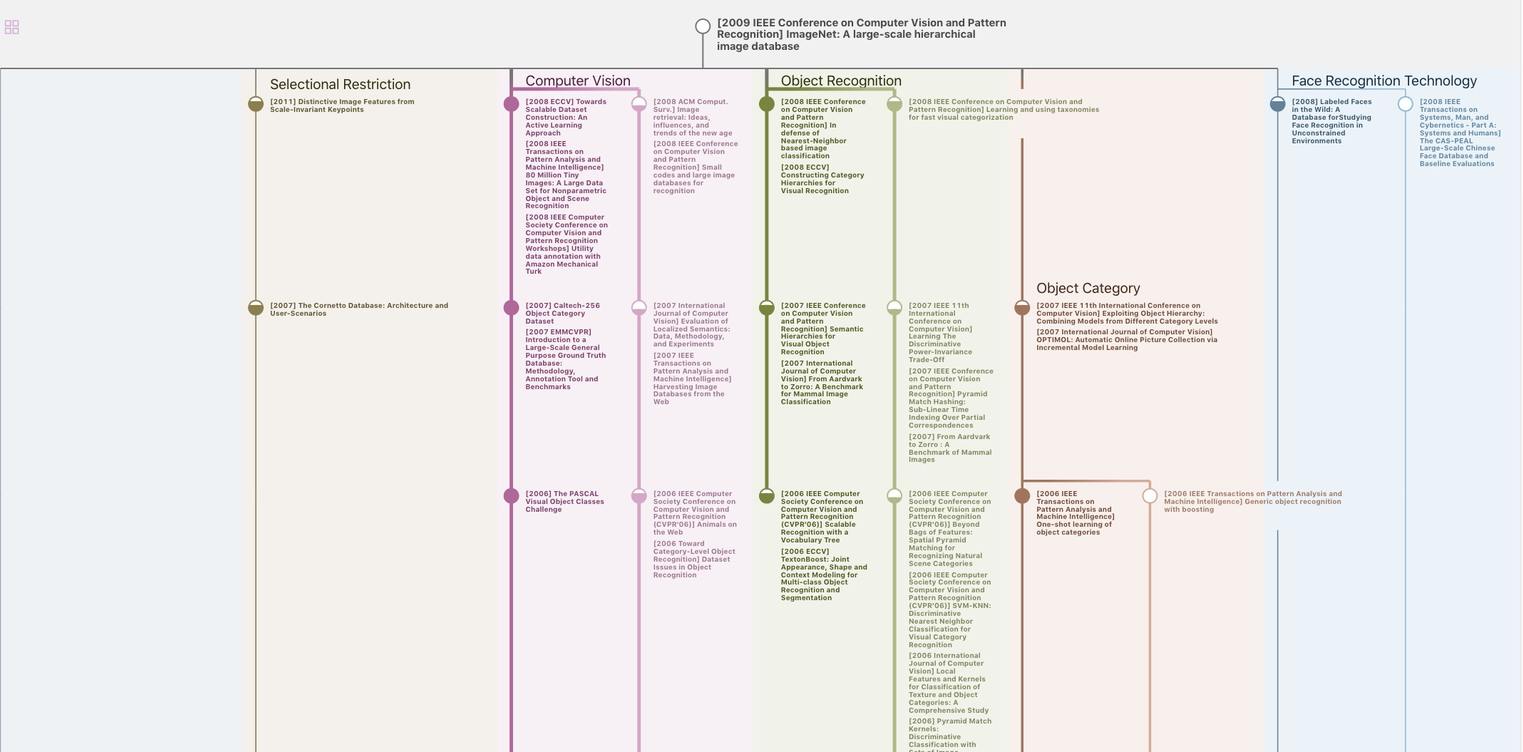
生成溯源树,研究论文发展脉络
Chat Paper
正在生成论文摘要