LensePro: label noise-tolerant prototype-based network for improving cancer detection in prostate ultrasound with limited annotations
International Journal of Computer Assisted Radiology and Surgery(2024)
摘要
The standard of care for prostate cancer (PCa) diagnosis is the histopathological analysis of tissue samples obtained via transrectal ultrasound (TRUS) guided biopsy. Models built with deep neural networks (DNNs) hold the potential for direct PCa detection from TRUS, which allows targeted biopsy and subsequently enhances outcomes. Yet, there are ongoing challenges with training robust models, stemming from issues such as noisy labels, out-of-distribution (OOD) data, and limited labeled data. This study presents LensePro, a unified method that not only excels in label efficiency but also demonstrates robustness against label noise and OOD data. LensePro comprises two key stages: first, self-supervised learning to extract high-quality feature representations from abundant unlabeled TRUS data and, second, label noise-tolerant prototype-based learning to classify the extracted features. Using data from 124 patients who underwent systematic prostate biopsy, LensePro achieves an AUROC, sensitivity, and specificity of 77.9 Through comprehensive experiments, LensePro demonstrates its state-of-the-art performance for TRUS-based PCa detection. Although further research is necessary to confirm its clinical applicability, LensePro marks a notable advancement in enhancing automated computer-aided systems for detecting prostate cancer in ultrasound.
更多查看译文
关键词
Ultrasound imaging,Image-guided interventions,Prostate biopsy,Noisy labels,Out of distribution data
AI 理解论文
溯源树
样例
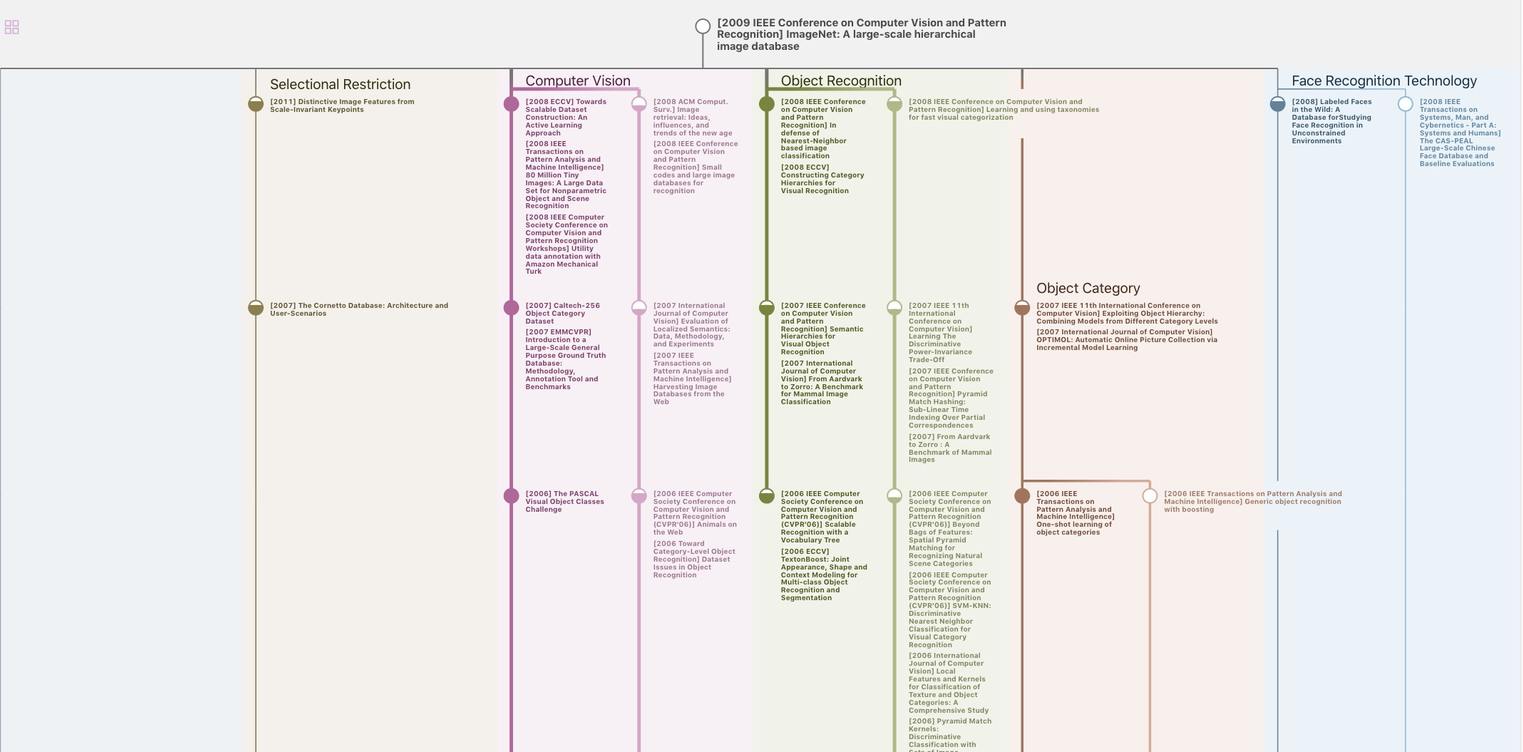
生成溯源树,研究论文发展脉络
Chat Paper
正在生成论文摘要