Merging Counter-Propagation and Back-Propagation Algorithms: Overcoming the Limitations of Counter-Propagation Neural Network Models
INTERNATIONAL JOURNAL OF MOLECULAR SCIENCES(2024)
摘要
Artificial neural networks (ANNs) are nowadays applied as the most efficient methods in the majority of machine learning approaches, including data-driven modeling for assessment of the toxicity of chemicals. We developed a combined neural network methodology that can be used in the scope of new approach methodologies (NAMs) assessing chemical or drug toxicity. Here, we present QSAR models for predicting the physical and biochemical properties of molecules of three different datasets: aqueous solubility, acute fish toxicity toward fat head minnow, and bio-concentration factors. A novel neural network modeling method is developed by combining two neural network algorithms, namely, the counter-propagation modeling strategy (CP-ANN) with the back-propagation-of-errors algorithm (BPE-ANN). The advantage is a short training time, robustness, and good interpretability through the initial CP-ANN part, while the extension with BPE-ANN improves the precision of predictions in the range between minimal and maximal property values of the training data, regardless of the number of neurons in both neural networks, either CP-ANN or BPE-ANN.
更多查看译文
关键词
counter-propagation CP-ANN,back-propagation-error BPE-ANN,machine learning,cheminformatics tool,QSAR model,water solubility,drug design,acute fish toxicity,bio-concentration factor
AI 理解论文
溯源树
样例
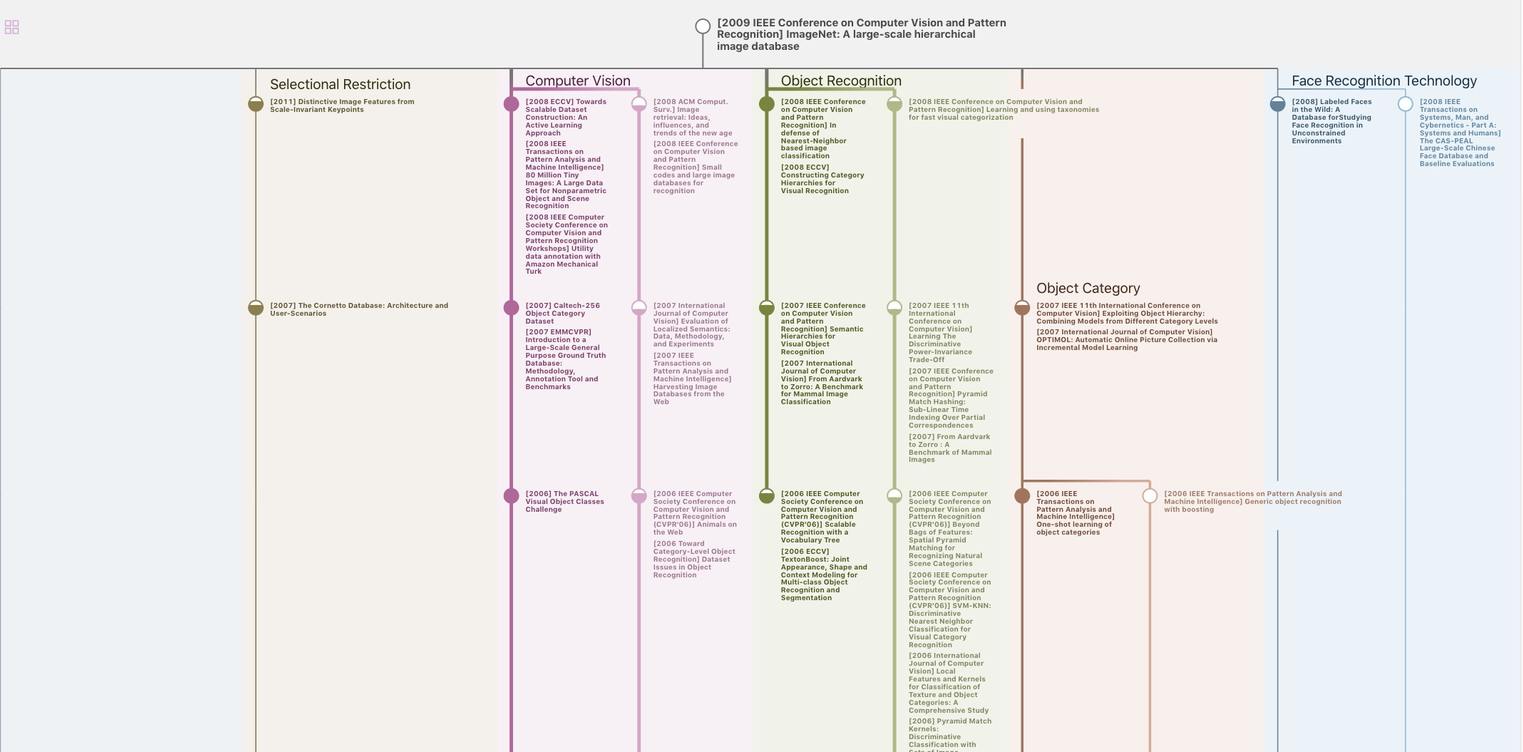
生成溯源树,研究论文发展脉络
Chat Paper
正在生成论文摘要