MaskArmor: Confidence Masking-based Defense Mechanism for GNN against MIA
Information Sciences(2024)
摘要
Graph neural networks (GNNs) have demonstrated remarkable performance in diverse graph-related tasks, including node classification, graph classification, link prediction, etc. Previous research has indicated that GNNs are vulnerable to membership inference attacks (MIA). These attacks enable malevolent parties to deduce whether the data points are part of the training set by identifying the output distribution, giving rise to noteworthy privacy apprehensions, especially when the graph contains sensitive data. There have been some studies to defend against graph MIA so far, but they have issues like high computational cost and decreased model accuracy. In this paper, we introduce a novel defense framework called MaskArmor, designed to bolster the privacy and security of GNNs against MIA. The MaskArmor framework encompasses four distinct masking strategies: AdjMask, DTMask, ATMask, and SigMask. These strategies leverage message-passing mechanisms, distillation temperature, hybrid masking, and the Sigmoid function, respectively. The MaskArmor framework effectively obscures the distribution of the model on both the training and non-training samples, rendering it challenging for attackers to ascertain whether particular samples have undergone training. Additionally, MaskArmor sustains the model's precision with negligible computational overhead. Our experiments are implemented across seven benchmark datasets and four GNN networks against shadow-based and threshold-based MIAs, showcasing that MaskArmor substantially heightens GNNs' resilience against MIA while simultaneously preserving accuracy on the initial tasks. It also demonstrates adeptness in countering threshold-based MIA through strategies like AdjMask and ATMask. Exhaustive experimental results substantiate that MaskArmor outperforms alternative existing approaches, maintaining effectiveness and applicability across diverse datasets and attack scenarios.
更多查看译文
关键词
GNNs,membership inference attack,privacy defense
AI 理解论文
溯源树
样例
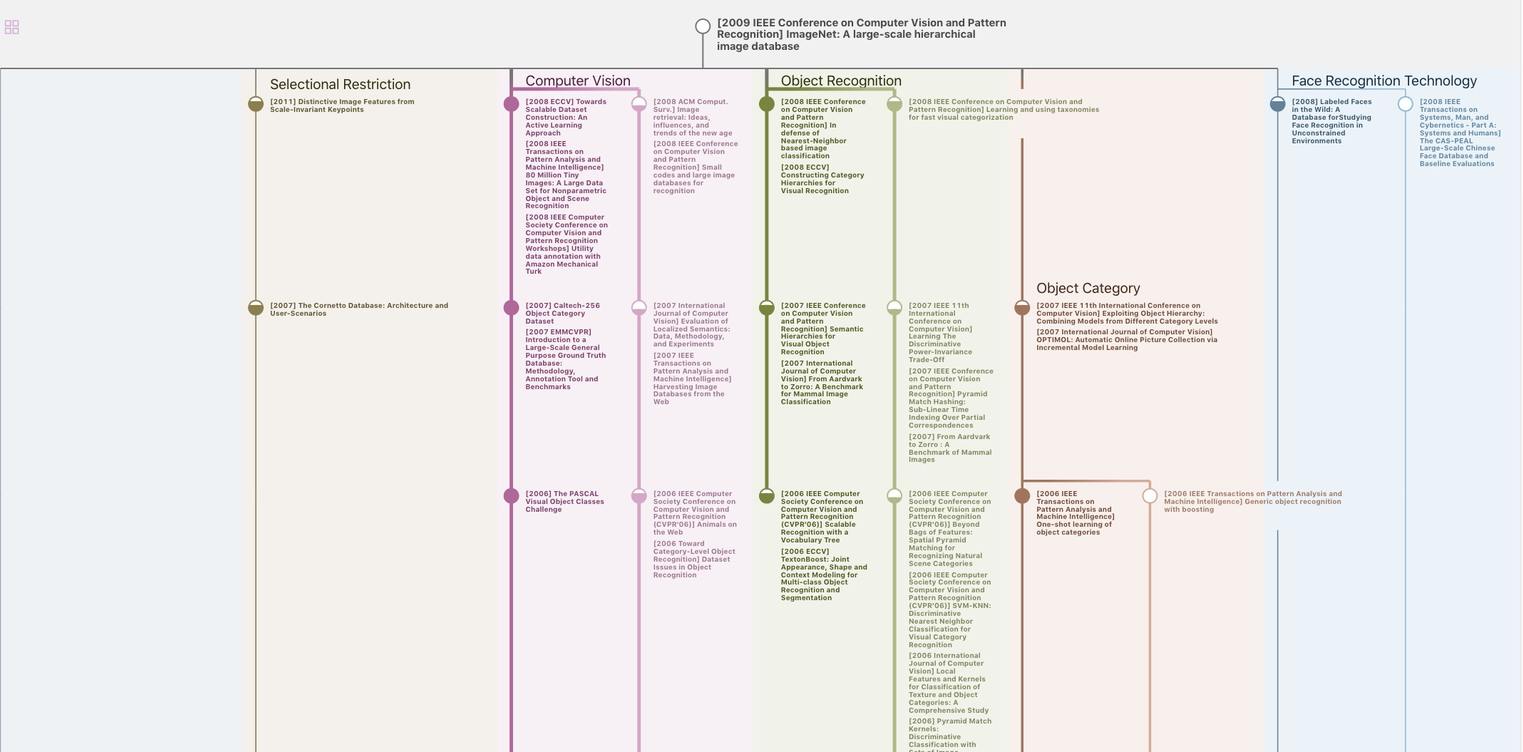
生成溯源树,研究论文发展脉络
Chat Paper
正在生成论文摘要