Towards Few-Label Vertical Federated Learning
ACM Transactions on Knowledge Discovery from Data(2024)
摘要
Federated Learning (FL) provided a novel paradigm for privacy-preserving machine learning, enabling multiple clients to collaborate on model training without sharing private data. To handle multi-source heterogeneous data, vertical federated learning (VFL) has been extensively investigated. However, in the context of VFL, the label information tends to be kept in one authoritative client and is very limited. This poses two challenges for model training in the VFL scenario: On the one hand, a small number of labels cannot guarantee to train a well VFL model with informative network parameters, resulting in unclear boundaries for classification decisions; On the other hand, the large amount of unlabeled data is dominant and should not be discounted, and it’s worthwhile to focus on how to leverage them to improve representation modeling capabilities. In order to address the above two challenges, Firstly, we introduce supervised contrastive loss to enhance the intra-class aggregation and inter-class estrangement, which is to deeply explore label information and improve the effectiveness of downstream classification tasks. Secondly, for unlabeled data, we introduce a pseudo-label-guided consistency mechanism to induce the classification results coherent across clients, which allows the representations learned by local networks to absorb the knowledge from other clients, and alleviates the disagreement between different clients for classification tasks. We conduct sufficient experiments on four commonly used datasets, and the experimental results demonstrate that our method is superior to the state-of-the-art methods, especially in the low-label rate scenario, and the improvement becomes more significant.
更多查看译文
AI 理解论文
溯源树
样例
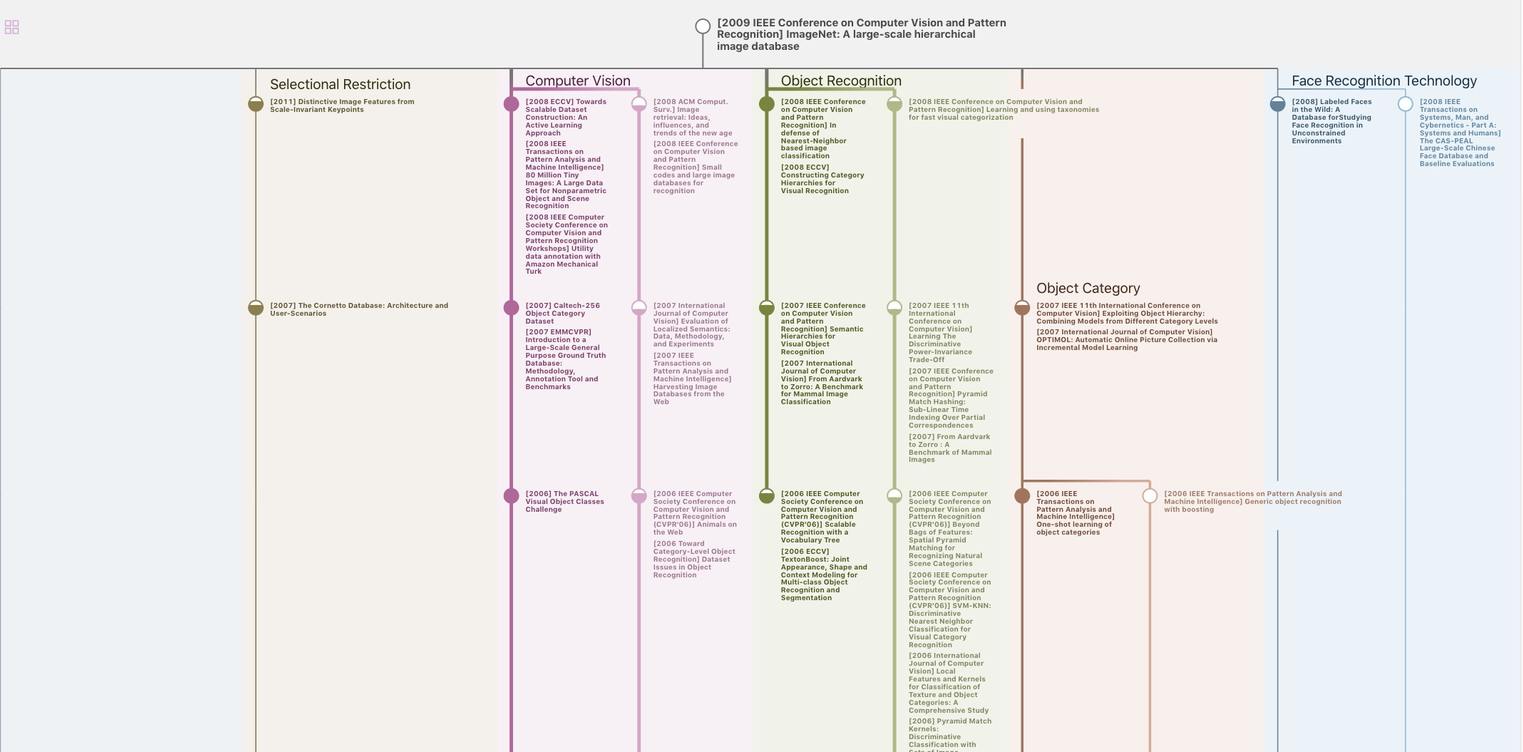
生成溯源树,研究论文发展脉络
Chat Paper
正在生成论文摘要