Resource Allocation for Cognitive Network Slicing in PD-SCMA System Based on Two-Way Deep Reinforcement Learning
CHINA COMMUNICATIONS(2024)
摘要
In this paper, we propose the Two-way Deep Reinforcement Learning (DRL)-Based resource allocation algorithm, which solves the problem of resource allocation in the cognitive downlink network based on the underlay mode. Secondary users (SUs) in the cognitive network are multiplexed by a new Power Domain Sparse Code Multiple Access (PD-SCMA) scheme, and the physical resources of the cognitive base station are virtualized into two types of slices: enhanced mobile broadband (eMBB) slice and ultrareliable low latency communication (URLLC) slice. We design the Double Deep Q Network (DDQN) network output the optimal codebook assignment scheme and simultaneously use the Deep Deterministic Policy Gradient (DDPG) network output the optimal power allocation scheme. The objective is to jointly optimize the spectral efficiency of the system and the Quality of Service (QoS) of SUs. Simulation results show that the proposed algorithm outperforms the CNDDQN algorithm and modified JEERA algorithm in terms of spectral efficiency and QoS satisfaction. Additionally, compared with the Power Domain Non-orthogonal Multiple Access (PD-NOMA) slices and the Sparse Code Multiple Access (SCMA) slices, the PD-SCMA slices can dramatically enhance spectral efficiency and increase the number of accessible users.
更多查看译文
关键词
cognitive radio,deep reinforcement learn- ing,network slicing,Power-domain Non-orthogonal Multiple Access,resource allocation
AI 理解论文
溯源树
样例
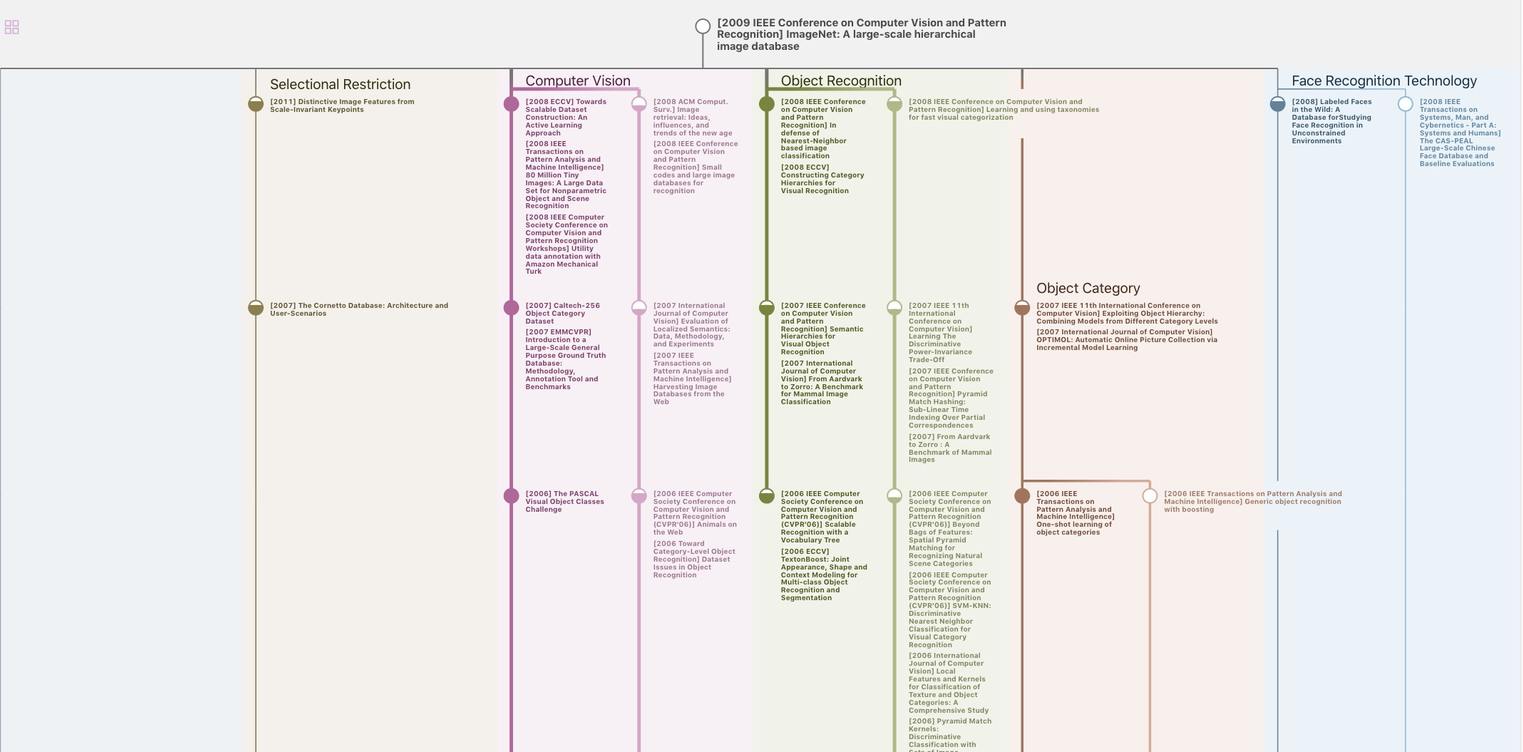
生成溯源树,研究论文发展脉络
Chat Paper
正在生成论文摘要