SoftAct: A High-Precision Softmax Architecture for Transformers Supporting Nonlinear Functions
IEEE Transactions on Circuits and Systems for Video Technology(2024)
摘要
Transformer-based deep learning networks are revolutionizing our society. The convolution and attention co-designed (CAC) Transformers have demonstrated superior performance compared to the conventional Transformer-based networks. However, CAC Transformer networks contain various nonlinear functions, such as softmax and complex activation functions, which require high precision hardware design yet typically with significant cost in area and power consumption. To address these challenges,
SoftAct
, a compact and high-precision algorithm-hardware co-designed architecture, is proposed to implement both softmax and nonlinear activation functions in CAC Transformer accelerators. An improved softmax algorithm with penalties is proposed to maintain precision in hardware. A stage-wise full zero detection method is developed to skip redundant computation in softmax. A compact and reconfigurable architecture with a symmetrically designed linear fitting module is proposed to achieve nonlinear functions. The
SoftAct
architecture is designed in an industrial 28-nm CMOS technology with the MobileViT-xxs network classifying the ImageNet-1k dataset as the benchmark. Compared with the state of the art,
SoftAct
improves up to 5.87% network accuracy under 8-bit quantization, 153.2× area efficiency, and 1435× overall efficiency.
更多查看译文
关键词
Transformer-based networks,nonlinear functions,softmax,sparsity detection,overall efficiency
AI 理解论文
溯源树
样例
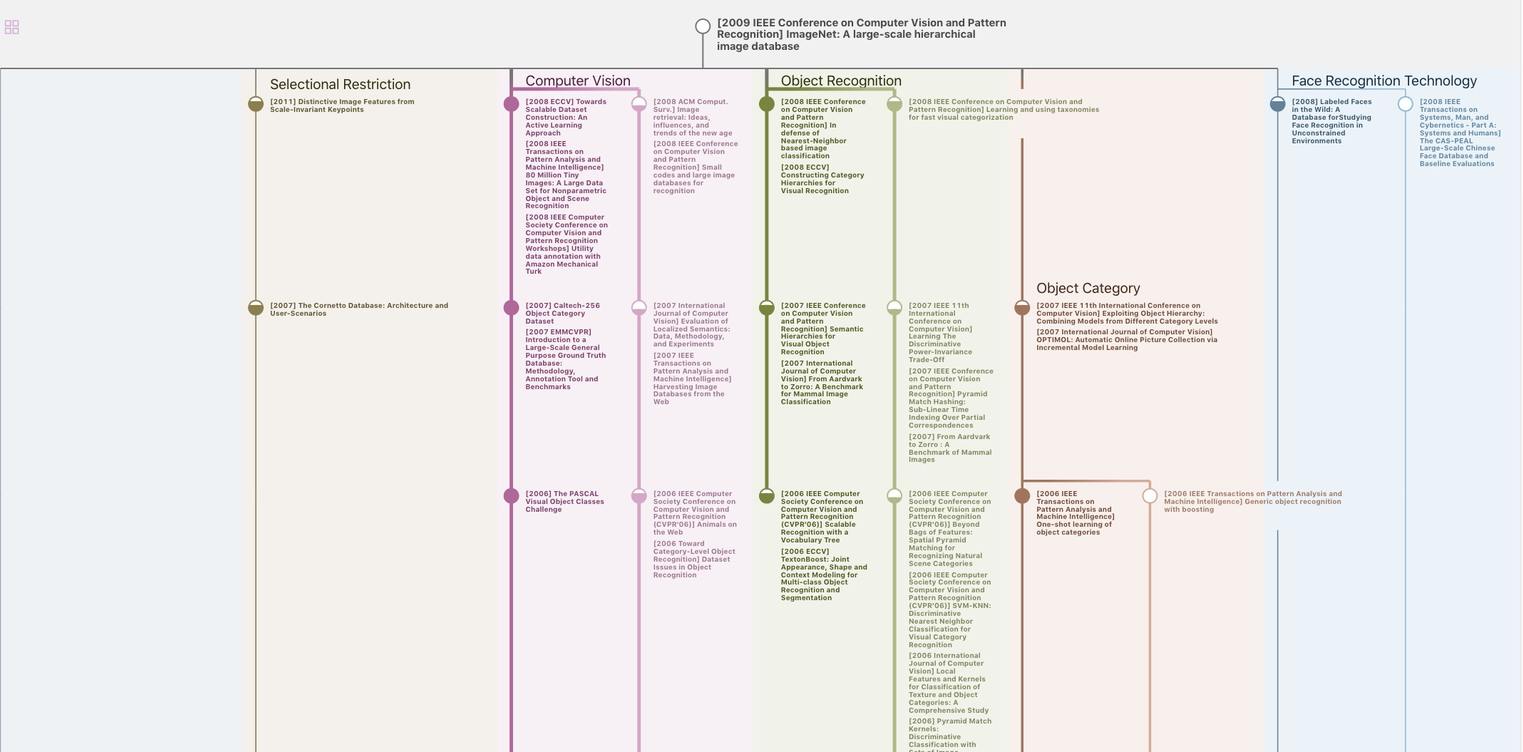
生成溯源树,研究论文发展脉络
Chat Paper
正在生成论文摘要