Full Range Feature Extraction Network Based on Quality-Quantity-Balance Sample Enhancement for Hyperspectral Image Classification
IEEE Transactions on Geoscience and Remote Sensing(2024)
摘要
Hyperspectral remote sensing images exhibit fine spectral curves, but they are also susceptible to spectral variations caused by factors like cloud and haze. It is evident that these issues become more pronounced when there is a limited number of labeled samples available. Thus, a full range feature extraction network (FRFENet) based on quality-quantity-balance sample enhancement is proposed for hyperspectral image classification. First, the full-range feature extraction method combines local-range, short-range, and long-range spatial-spectral features to address spectral variability and ensure accurate feature extraction, particularly in scenarios with limited labeled samples. Furthermore, the approach of balancing quality and quantity for pseudo-labeled samples allows for an increased number of pseudo-labels while maintaining their quality, effectively leveraging unlabeled samples. Additionally, the utilization of superpixel region homogeneity directly contributes to an expanded training sample set, resulting in improved classification performance of the algorithm. Experiments on three HSI datasets indicate that the FRFENet can obtain better classification performance when compared with the other ten state-of-the-art methods.
更多查看译文
关键词
Hyperspectral image classification,full range feature extraction,graph convolution,convolutional neural network,sample enhancement
AI 理解论文
溯源树
样例
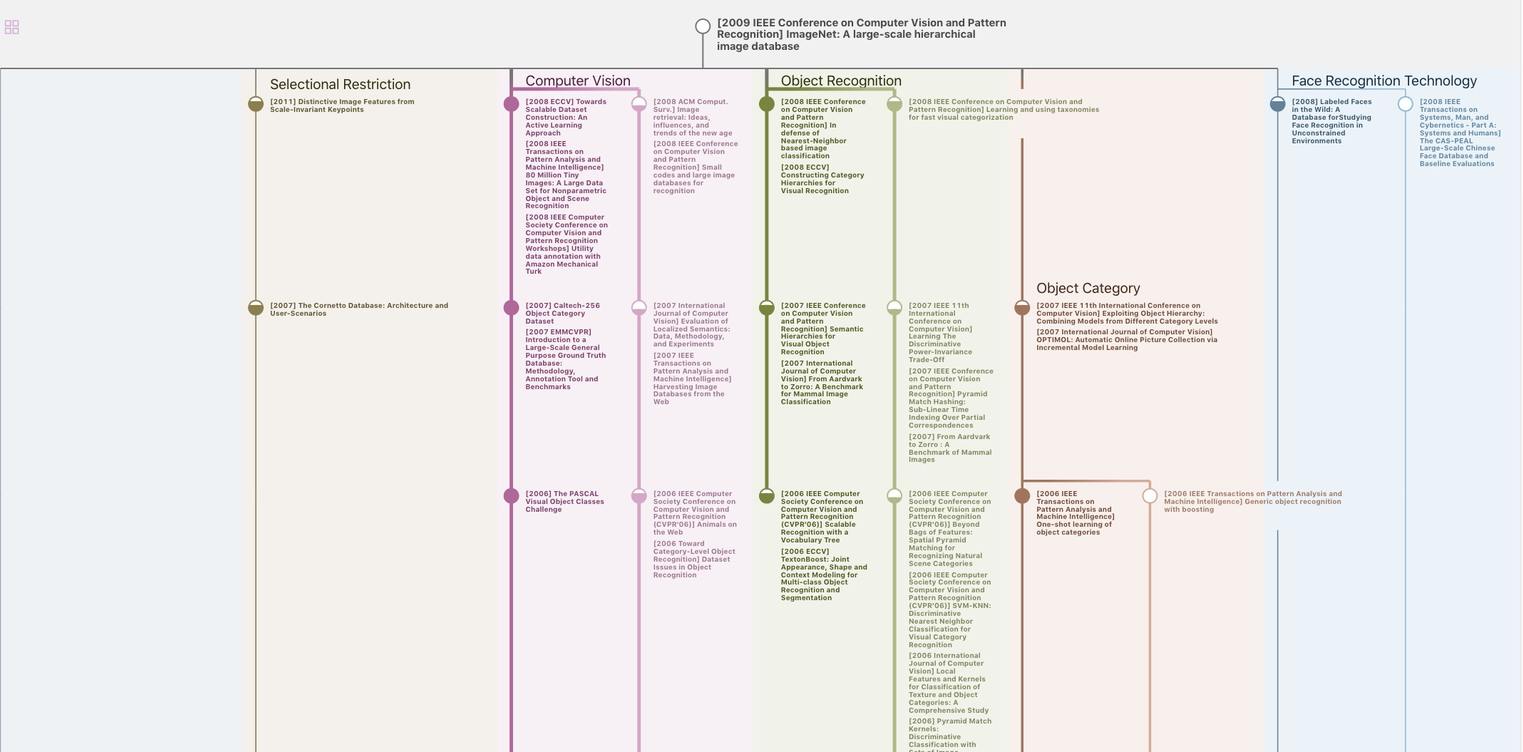
生成溯源树,研究论文发展脉络
Chat Paper
正在生成论文摘要