The Power in Communication: Power Regularization of Communication for Autonomy in Cooperative Multi-Agent Reinforcement Learning
arxiv(2024)
摘要
Communication plays a vital role for coordination in Multi-Agent
Reinforcement Learning (MARL) systems. However, misaligned agents can exploit
other agents' trust and delegated power to the communication medium. In this
paper, we propose power regularization as a method to limit the adverse effects
of communication by misaligned agents, specifically communication which impairs
the performance of cooperative agents. Power is a measure of the influence one
agent's actions have over another agent's policy. By introducing power
regularization, we aim to allow designers to control or reduce agents'
dependency on communication when appropriate, and make them more resilient to
performance deterioration due to misuses of communication. We investigate
several environments in which power regularization can be a valuable capability
for learning different policies that reduce the effect of power dynamics
between agents during communication.
更多查看译文
AI 理解论文
溯源树
样例
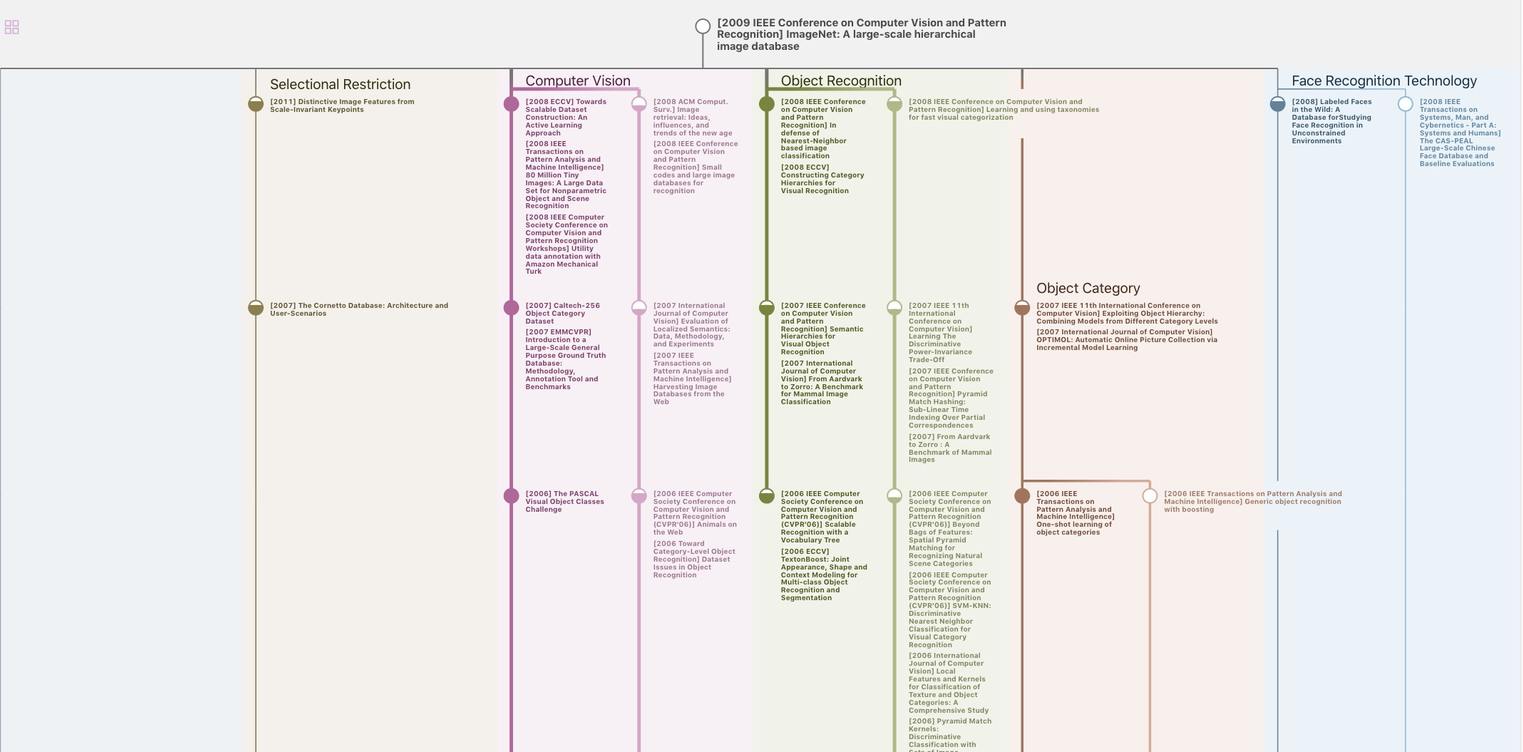
生成溯源树,研究论文发展脉络
Chat Paper
正在生成论文摘要