Inexact Policy Iteration Methods for Large-Scale Markov Decision Processes
arxiv(2024)
摘要
We consider inexact policy iteration methods for large-scale infinite-horizon
discounted MDPs with finite spaces, a variant of policy iteration where the
policy evaluation step is implemented inexactly using an iterative solver for
linear systems. In the classical dynamic programming literature, a similar
principle is deployed in optimistic policy iteration, where an a-priori
fixed-number of iterations of value iteration is used to inexactly solve the
policy evaluation step. Inspired by the connection between policy iteration and
semismooth Newton's method, we investigate a class of iPI methods that mimic
the inexact variants of semismooth Newton's method by adopting a parametric
stopping condition to regulate the level of inexactness of the policy
evaluation step. For this class of methods we discuss local and global
convergence properties and derive a practical range of values for the
stopping-condition parameter that provide contraction guarantees. Our analysis
is general and therefore encompasses a variety of iterative solvers for policy
evaluation, including the standard value iteration as well as more
sophisticated ones such as GMRES. As underlined by our analysis, the selection
of the inner solver is of fundamental importance for the performance of the
overall method. We therefore consider different iterative methods to solve the
policy evaluation step and analyze their applicability and contraction
properties when used for policy evaluation. We show that the contraction
properties of these methods tend to be enhanced by the specific structure of
policy evaluation and that there is margin for substantial improvement in terms
of convergence rate. Finally, we study the numerical performance of different
instances of inexact policy iteration on large-scale MDPs for the design of
health policies to control the spread of infectious diseases in epidemiology.
更多查看译文
AI 理解论文
溯源树
样例
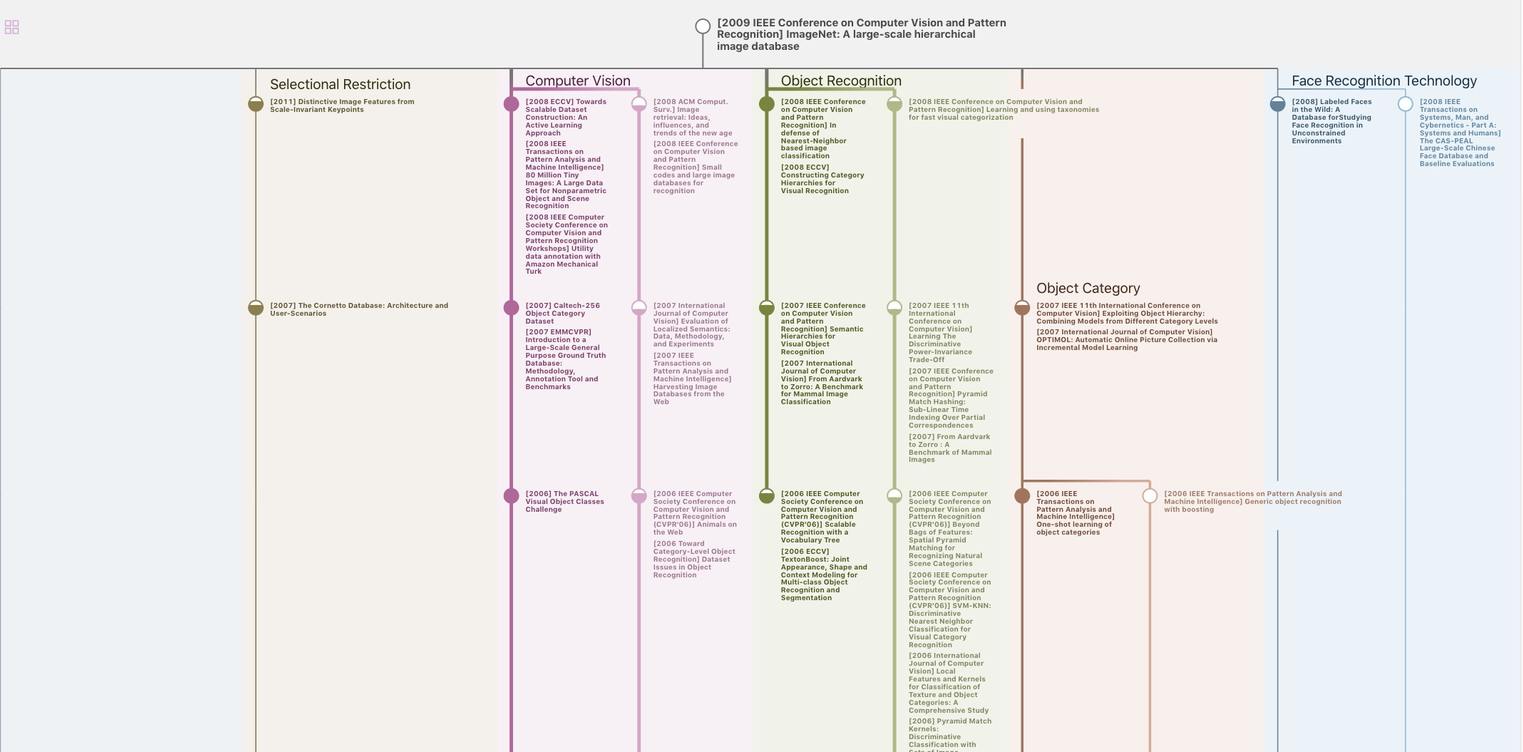
生成溯源树,研究论文发展脉络
Chat Paper
正在生成论文摘要