Collaborative Edge AI Inference over Cloud-RAN
IEEE Transactions on Communications(2024)
摘要
In this paper, a cloud radio access network (Cloud-RAN) based collaborative
edge AI inference architecture is proposed. Specifically, geographically
distributed devices capture real-time noise-corrupted sensory data samples and
extract the noisy local feature vectors, which are then aggregated at each
remote radio head (RRH) to suppress sensing noise. To realize efficient uplink
feature aggregation, we allow each RRH receives local feature vectors from all
devices over the same resource blocks simultaneously by leveraging an
over-the-air computation (AirComp) technique. Thereafter, these aggregated
feature vectors are quantized and transmitted to a central processor (CP) for
further aggregation and downstream inference tasks. Our aim in this work is to
maximize the inference accuracy via a surrogate accuracy metric called
discriminant gain, which measures the discernibility of different classes in
the feature space. The key challenges lie on simultaneously suppressing the
coupled sensing noise, AirComp distortion caused by hostile wireless channels,
and the quantization error resulting from the limited capacity of fronthaul
links. To address these challenges, this work proposes a joint transmit
precoding, receive beamforming, and quantization error control scheme to
enhance the inference accuracy. Extensive numerical experiments demonstrate the
effectiveness and superiority of our proposed optimization algorithm compared
to various baselines.
更多查看译文
关键词
Cloud radio access network,edge AI,edge inference,over-the-air computation
AI 理解论文
溯源树
样例
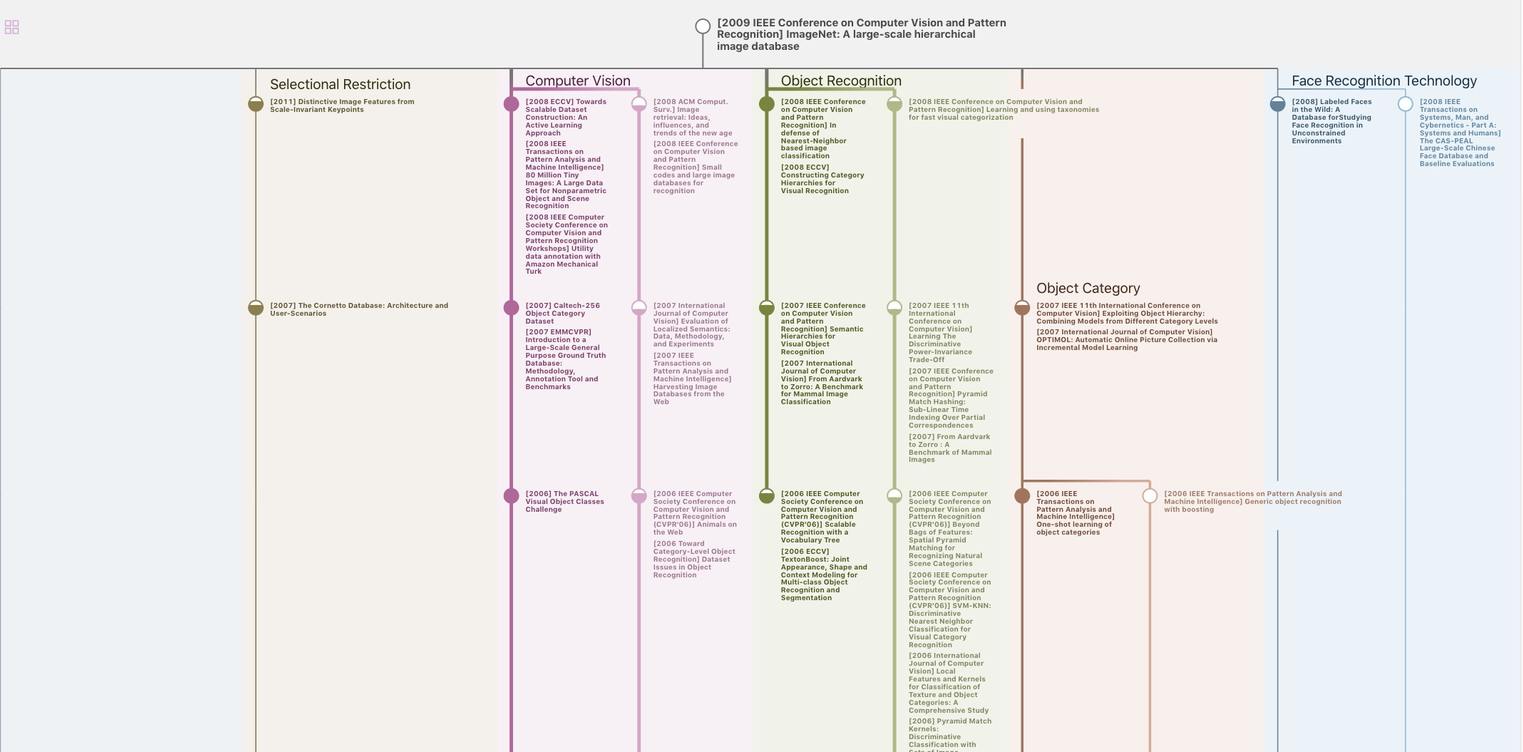
生成溯源树,研究论文发展脉络
Chat Paper
正在生成论文摘要