Linking convolutional kernel size to generalization bias in face analysis CNNs.
IEEE/CVF Winter Conference on Applications of Computer Vision(2024)
摘要
Training dataset biases are by far the most scrutinized factors when explaining algorithmic biases of neural networks. In contrast, hyperparameters related to the neural network architecture have largely been ignored even though different network parameterizations are known to induce different implicit biases over learned features. For example, convolutional kernel size is known to affect the frequency content of features learned in CNNs. In this work, we present a causal framework for linking an architectural hyperparameter to out-of-distribution algorithmic bias. Our framework is experimental, in that we train several versions of a network with an intervention to a specific hyperparameter, and measure the resulting causal effect of this choice on performance bias when a particular out-of-distribution image perturbation is applied. In our experiments, we focused on measuring the causal relationship between convolutional kernel size and face analysis classification bias across different subpopulations (race/gender), with respect to high-frequency image details. We show that modifying kernel size, even in one layer of a CNN, changes the frequency content of learned features significantly across data subgroups leading to biased generalization performance even in the presence of a balanced dataset.
更多查看译文
关键词
Algorithms,Explainable,fair,accountable,privacy-preserving,ethical computer vision,Algorithms,Adversarial learning,adversarial attack and defense methods,Applications,Social good
AI 理解论文
溯源树
样例
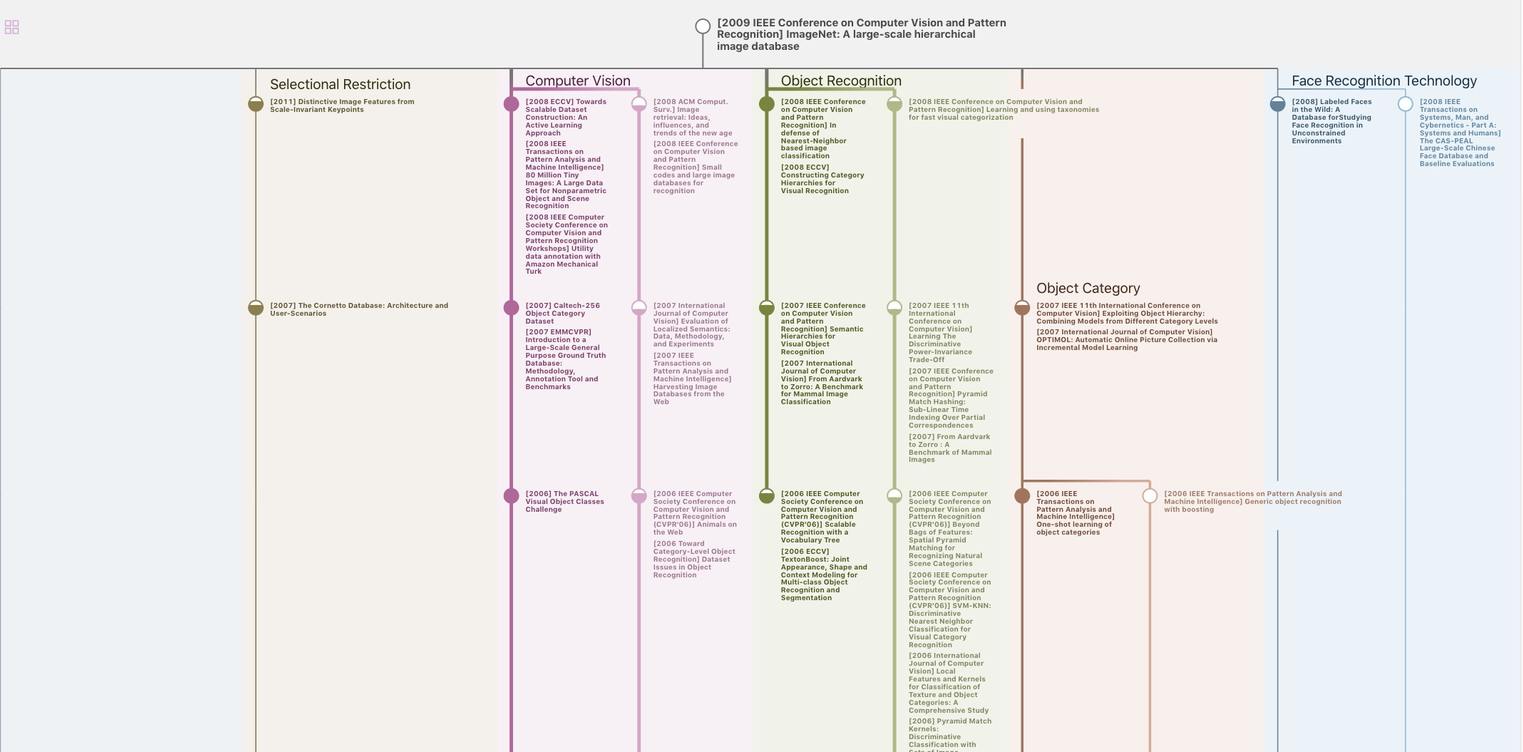
生成溯源树,研究论文发展脉络
Chat Paper
正在生成论文摘要