Re-Evaluating LiDAR Scene Flow.
IEEE/CVF Winter Conference on Applications of Computer Vision(2024)
摘要
Popular benchmarks for self-supervised LiDAR scene flow (stereoKITTI, and FlyingThings3D) have unrealistic rates of dynamic motion, unrealistic correspondences, and unrealistic sampling patterns. As a result, progress on these benchmarks is misleading and may cause researchers to focus on the wrong problems. We evaluate a suite of top methods on a suite of real-world datasets (Argoverse 2.0, Waymo, and NuScenes) and report several conclusions. First, we find that performance on stereoKITTI is negatively correlated with performance on real-world data. Second, we find that one of this task’s key components –removing the dominant ego-motion –is better solved by classic ICP than any tested method. Finally, we show that despite the emphasis placed on learning, most performance gains are caused by pre- and post-processing steps: piecewise-rigid refinement and ground removal. We demonstrate this through a baseline method that combines these processing steps with a learning-free test-time flow optimization. This baseline outperforms every evaluated method.
更多查看译文
关键词
Applications,Autonomous Driving,Algorithms,Datasets and evaluations
AI 理解论文
溯源树
样例
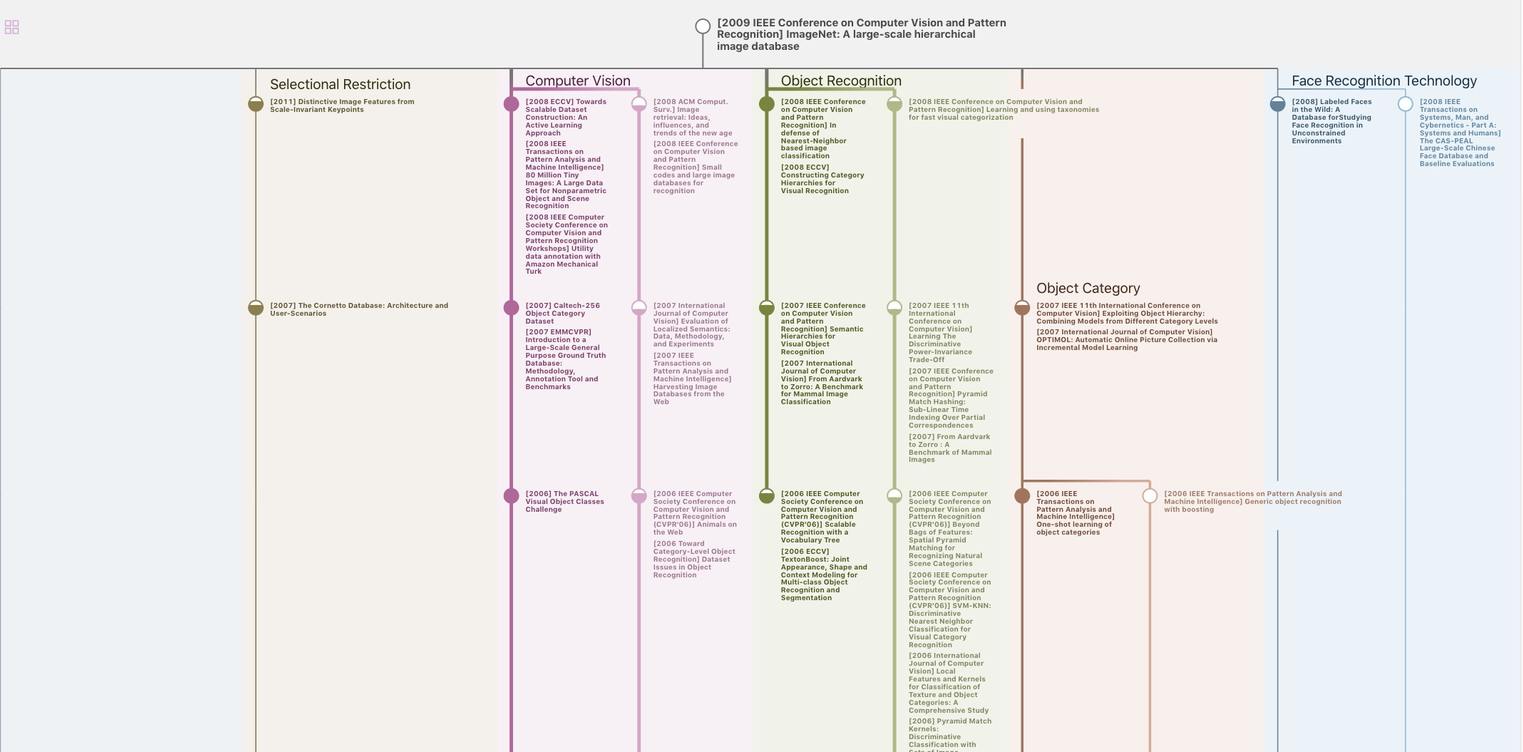
生成溯源树,研究论文发展脉络
Chat Paper
正在生成论文摘要