Hard Sample-aware Consistency for Low-resolution Facial Expression Recognition.
IEEE/CVF Winter Conference on Applications of Computer Vision(2024)
摘要
Facial expression recognition (FER) plays a pivotal role in computer vision applications, encompassing video understanding and human-computer interaction. Despite notable advancements in FER, performance still falters when handling low-resolution facial images encountered in real-world scenarios and datasets. While consistency constraint techniques have garnered attention for generating robust convolutional neural network models that accommodate input variations through augmentation, their efficacy is diminished in the realm of low-resolution FER. This decline in performance can be attributed to augmented samples that networks struggle to extract expressive features. In this paper, we identify hard samples that cause an overfitting problem when considering various degrees of resolution and propose novel hard sample-aware consistency (HSAC) loss functions, which include combined attention consistency and label distribution learning. The combined attention consistency aligns an attention map from multi-scale low-resolution images with an appropriate target attention map by combining activation maps from high-resolution and flipped low-resolution images. We measure the classification difficulty for low-resolution face images and adaptively apply label distribution learning by combining the original target and predictions of high-resolution input. Our HSAC empowers the network to achieve generalization by effectively managing hard samples. Extensive experiments on various FER datasets demonstrate the superiority of our proposed method over existing approaches for multiscale low-resolution images. Furthermore, we achieved a new state-of-the-art performance of 90.97% on the original RAF-DB dataset.
更多查看译文
关键词
Algorithms,Image recognition and understanding,Applications,Psychology and cognitive science
AI 理解论文
溯源树
样例
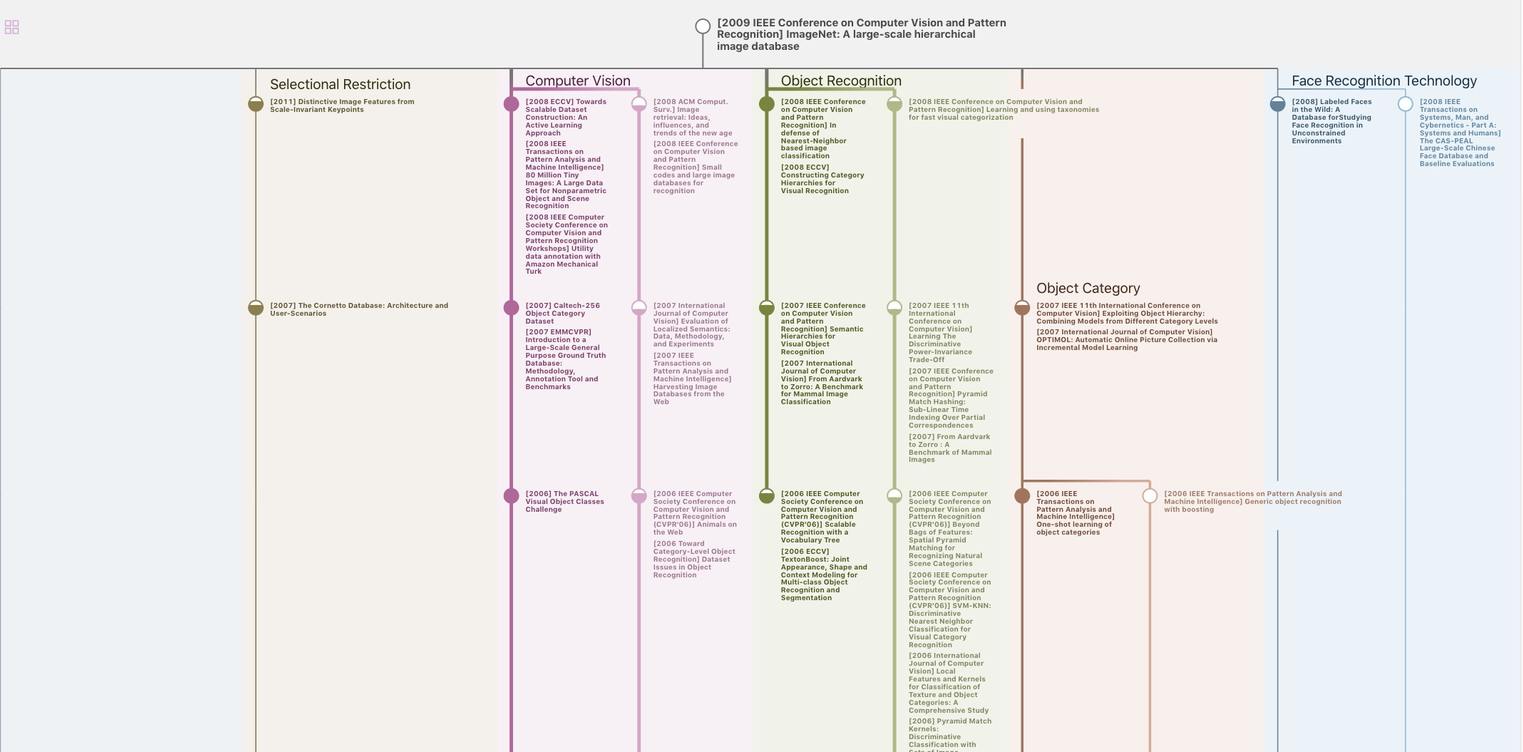
生成溯源树,研究论文发展脉络
Chat Paper
正在生成论文摘要