Intelligent fault diagnosis of photovoltaic systems based on deep digital twin
MEASUREMENT SCIENCE AND TECHNOLOGY(2024)
摘要
The energy loss and substantial costs associated with faults in photovoltaic (PV) systems impose significant limitations on their efficiency and reliability. Addressing current issues in PV fault diagnosis such as the lack of typical fault data, imbalanced data distribution, and poor diagnostic performance, this paper proposes an intelligent fault diagnosis method for PV systems, deep digital twins (DDT) with information gain stacking sparse autoencoders (IGSSAEs). Initially, the method designs a novel DDT modeling framework tailored to actual PV system specifications. This framework utilizes a mechanism simulation model to generate typical data under various states. Simultaneously, a deep data model is constructed to learn the distribution characteristics of the mechanism model and complete data diversification, achieving the fusion and complementation of data from both models. Subsequently, a diagnostic network using IGSSAE is introduced. This network utilizes information gain ratio to assess feature classification contributions, enabling automatic feature selection. Based on the input features, a stacked sparse autoencoder fault classification network is designed, incorporating multi-level feature compression to enhance the model's stability and diagnostic accuracy. Finally, a case study is conducted using a 250 kW grid-connected PV system, thoroughly validating the method's effectiveness with a diagnostic accuracy of 98.4%.
更多查看译文
关键词
photovoltaic system,digital twin,intelligent fault diagnosis,deep learning
AI 理解论文
溯源树
样例
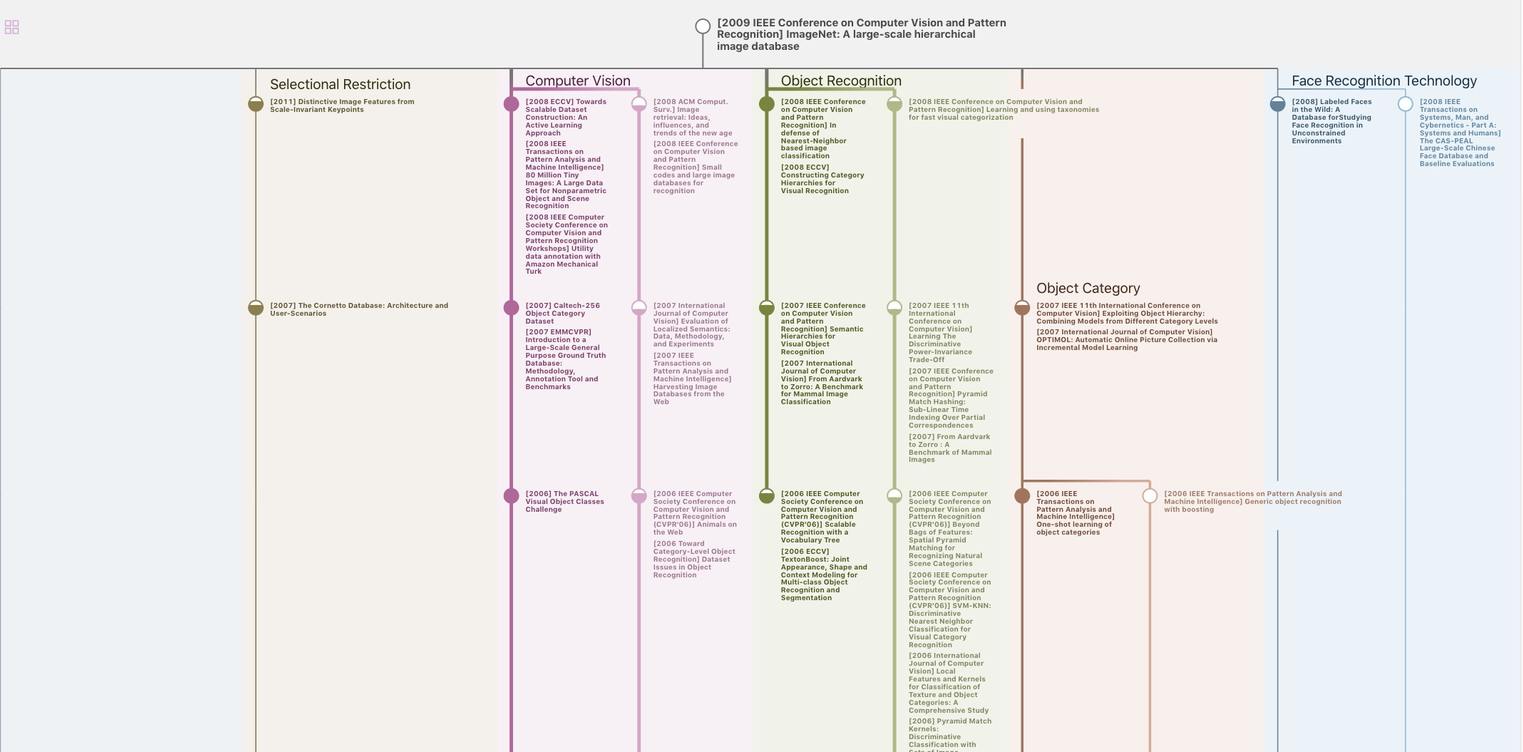
生成溯源树,研究论文发展脉络
Chat Paper
正在生成论文摘要