Multi-Task Foreground-Aware Network with Depth Completion for Enhanced RGB-D Fusion Object Detection Based on Transformer
SENSORS(2024)
摘要
Fusing multiple sensor perceptions, specifically LiDAR and camera, is a prevalent method for target recognition in autonomous driving systems. Traditional object detection algorithms are limited by the sparse nature of LiDAR point clouds, resulting in poor fusion performance, especially for detecting small and distant targets. In this paper, a multi-task parallel neural network based on the Transformer is constructed to simultaneously perform depth completion and object detection. The loss functions are redesigned to reduce environmental noise in depth completion, and a new fusion module is designed to enhance the network's perception of the foreground and background. The network leverages the correlation between RGB pixels for depth completion, completing the LiDAR point cloud and addressing the mismatch between sparse LiDAR features and dense pixel features. Subsequently, we extract depth map features and effectively fuse them with RGB features, fully utilizing the depth feature differences between foreground and background to enhance object detection performance, especially for challenging targets. Compared to the baseline network, improvements of 4.78%, 8.93%, and 15.54% are achieved in the difficult indicators for cars, pedestrians, and cyclists, respectively. Experimental results also demonstrate that the network achieves a speed of 38 fps, validating the efficiency and feasibility of the proposed method.
更多查看译文
关键词
point cloud data,YOLO,Transformer,multi-source feature fusion,depth completion
AI 理解论文
溯源树
样例
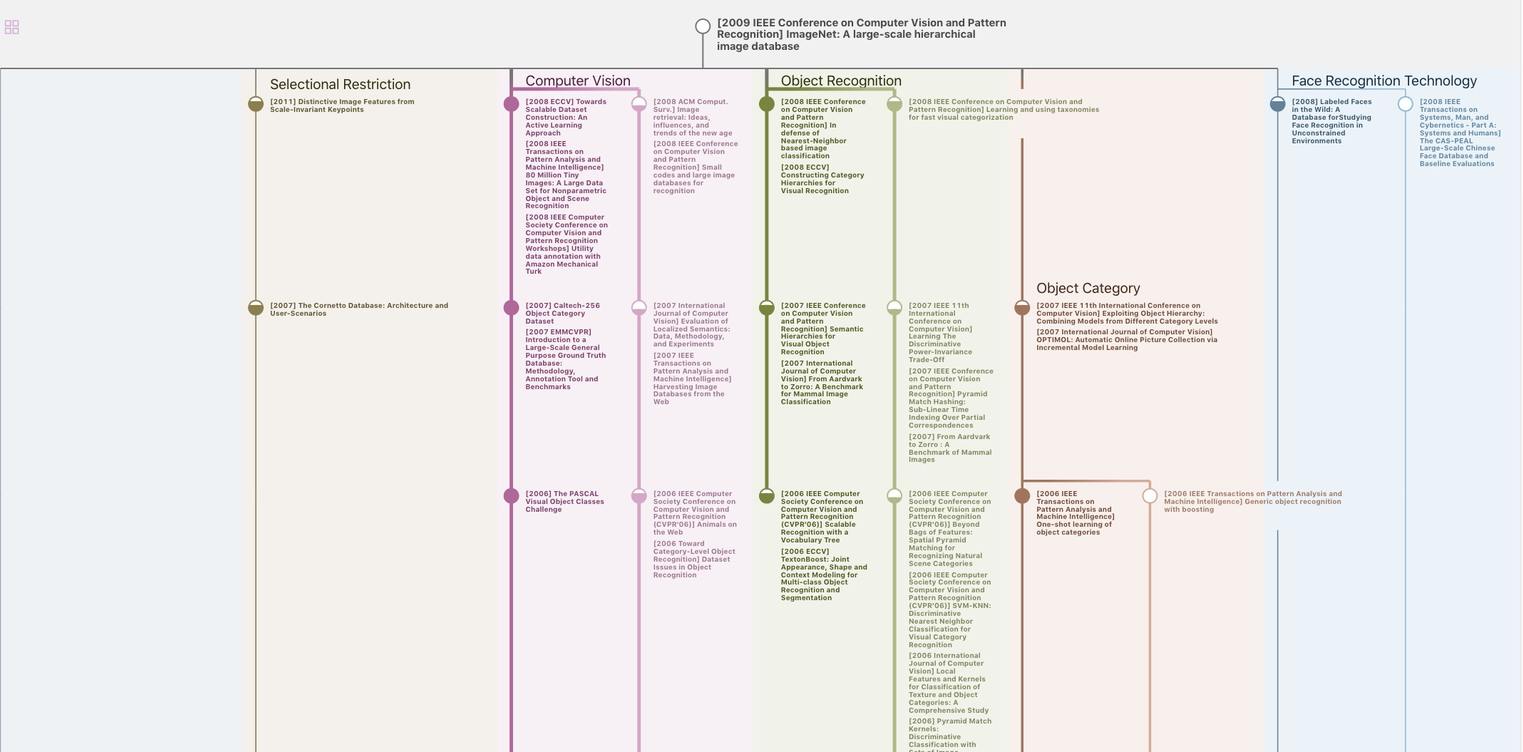
生成溯源树,研究论文发展脉络
Chat Paper
正在生成论文摘要