Weighted Differential Gradient Method for Filling Pits in Light Detection and Ranging (LiDAR) Canopy Height Model
REMOTE SENSING(2024)
摘要
The canopy height model (CHM) derived from LiDAR point cloud data is usually used to accurately identify the position and the canopy dimension of single tree. However, local invalid values (also called data pits) are often encountered during the generation of CHM, which results in low-quality CHM and failure in the detection of treetops. For this reason, this paper proposes an innovative method, called "pixels weighted differential gradient", to filter these data pits accurately and improve the quality of CHM. First, two characteristic parameters, gradient index (GI) and Z-score value (ZV) are extracted from the weighted differential gradient between the pit pixels and their eight neighbors, and then GIs and ZVs are commonly used as criterion for initial identification of data pits. Secondly, CHMs of different resolutions are merged, using the image processing algorithm developed in this paper to distinguish either canopy gaps or data pits. Finally, potential pits were filtered and filled with a reasonable value. The experimental validation and comparative analysis were carried out in a coniferous forest located in Triangle Lake, United States. The experimental results showed that our method could accurately identify potential data pits and retain the canopy structure information in CHM. The root-mean-squared error (RMSE) and mean bias error (MBE) from our method are reduced by between 73% and 26% and 76% and 28%, respectively, when compared with six other methods, including the mean filter, Gaussian filter, median filter, pit-free, spike-free and graph-based progressive morphological filtering (GPMF). The average F1 score from our method could be improved by approximately 4% to 25% when applied in single-tree extraction.
更多查看译文
关键词
LiDAR,canopy height model,gradient,pit filling,forest
AI 理解论文
溯源树
样例
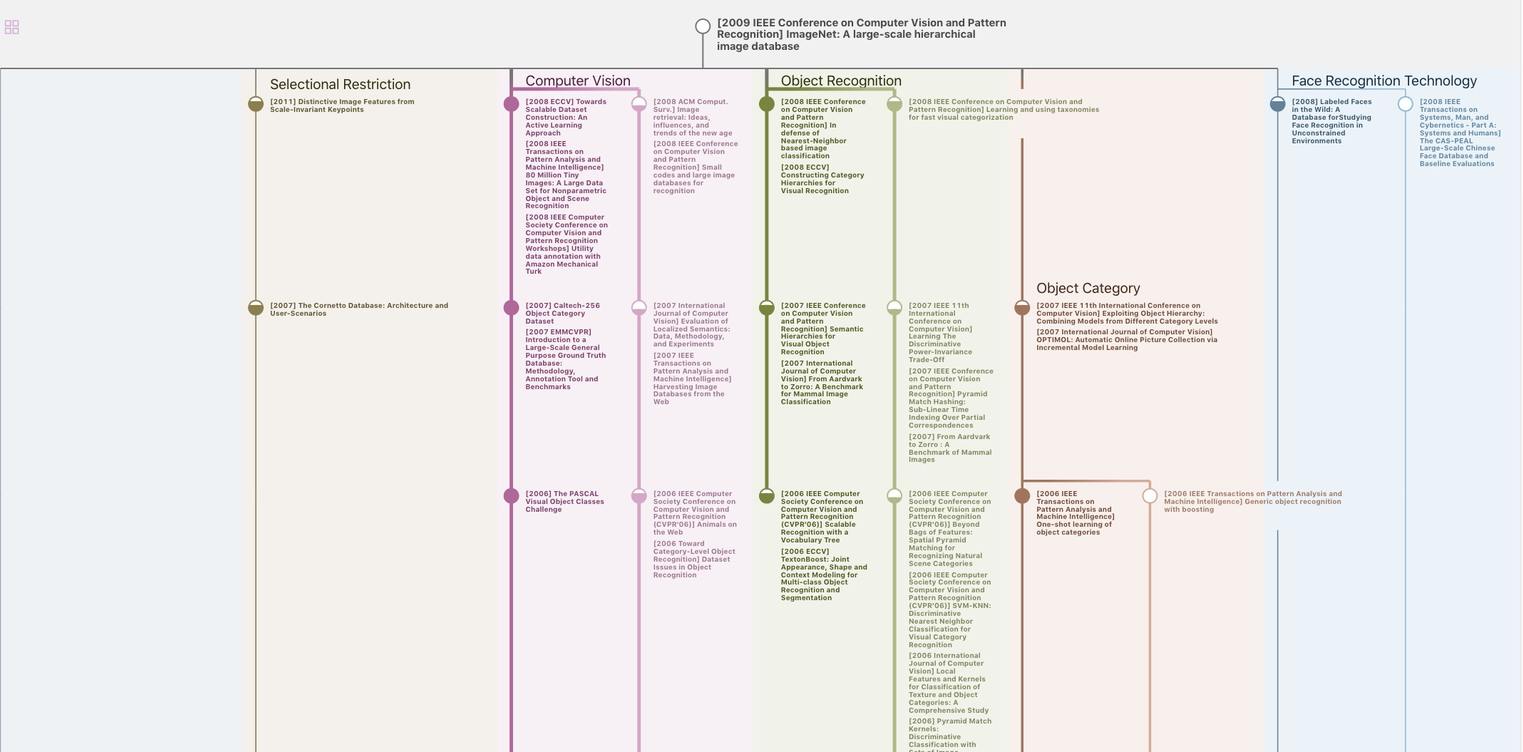
生成溯源树,研究论文发展脉络
Chat Paper
正在生成论文摘要