Audio-deepfake detection: Adversarial attacks and countermeasures
Expert Systems with Applications(2024)
摘要
Audio has always been a powerful resource for biometric authentication: thus, numerous AI-based audio authentication systems (classifiers) have been proposed. While these classifiers are effective in identifying legitimate human-generated input their security, to the best of our knowledge, has not been explored thoroughly when confronted with advanced attacks that leverage AI-generated deepfake audio. This issue presents a serious concern regarding the security of these classifiers because, e.g., samples generated using adversarial attacks might fool such classifiers, resulting in incorrect classification. In this study, we prove the point: we demonstrate that state-of-the-art audio deepfake classifiers are vulnerable to adversarial attacks. In particular, we design two adversarial attacks on a state-of-the-art audio-deepfake classifier, i.e., the Deep4SNet classification model, which achieves 98.5% accuracy in detecting fake audio samples. The designed adversarial attacks11The code of the attacks will be released open-source in the camera ready. leverage a generative adversarial network architecture and reduce the detector’s accuracy to nearly 0%. In particular, under graybox attack scenarios, we demonstrate that when starting from random noise, we can reduce the accuracy of the state-of-the-art detector from 98.5% to only 0.08%. To mitigate the effect of adversarial attacks on audio-deepfake detectors, we propose a highly generalizable, lightweight, simple, and effective add-on defense mechanism that can be implemented in any audio-deepfake detector. Finally, we discuss promising research directions.
更多查看译文
关键词
Authentication,Adversarial attacks,Audio deepfake,Fake voice detection,GAN,Biometrics,Security
AI 理解论文
溯源树
样例
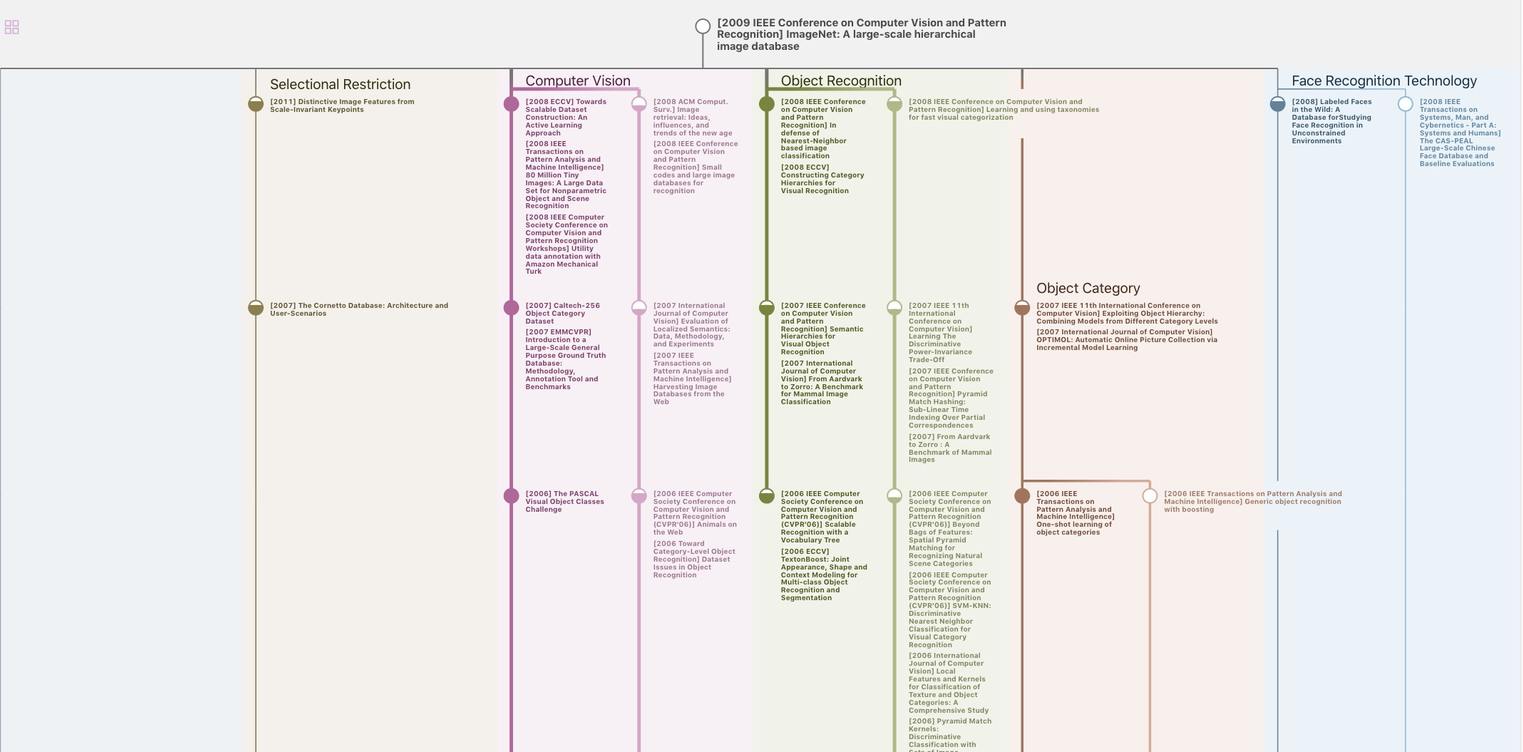
生成溯源树,研究论文发展脉络
Chat Paper
正在生成论文摘要