Cross-domain Few-shot Learning Based on Feature Disentanglement for Hyperspectral Image Classification
IEEE Transactions on Geoscience and Remote Sensing(2024)
摘要
Existing hyperspectral cross-domain few-shot learning (FSL) methods focus mainly on elaborating on training strategies or domain alignment algorithms, while paying less attention to the biased meta-knowledge introduced by a large amount of source data and the implicit encouragement of learning target domain-specific attributes. In this paper, from the perspective of disentangled representation learning, a novel cross-domain FSL method based on feature disentanglement (FDFSL) is proposed for hyperspectral image classification (HSIC). Specifically, to suppress the representation biased towards the source data and enable the model to implicitly focus on the inherent knowledge of the target domain, an orthogonal low-rank feature disentanglement method is employed to acquire desired features of source and target pipelines. Furthermore, to preserve more shared and discriminative information from the heterogeneous data space (i.e., the spectral dimensions of the source and target scenes are typically different), a multi-order spectral interaction block based on central position encoding (MICD) is proposed to fully integrate the respective features into the spectral domain, which allows the model to emphasize informative spectral dimensions in a data-driven manner. Finally, to diversify the feature representation space while preventing the model overfitting domain alignment task, a self-distillation scheme is developed to facilitate the acquisition of task-relevant feature components. Extensive experiments and analysis on three public HSI datasets suggest the superiority of the proposed method. The code will be available on the website at https://github.com/Qba-heu/FDFSL.
更多查看译文
关键词
Hyperspectral image classification,few-shot learning,cross-domain,feature disentanglement
AI 理解论文
溯源树
样例
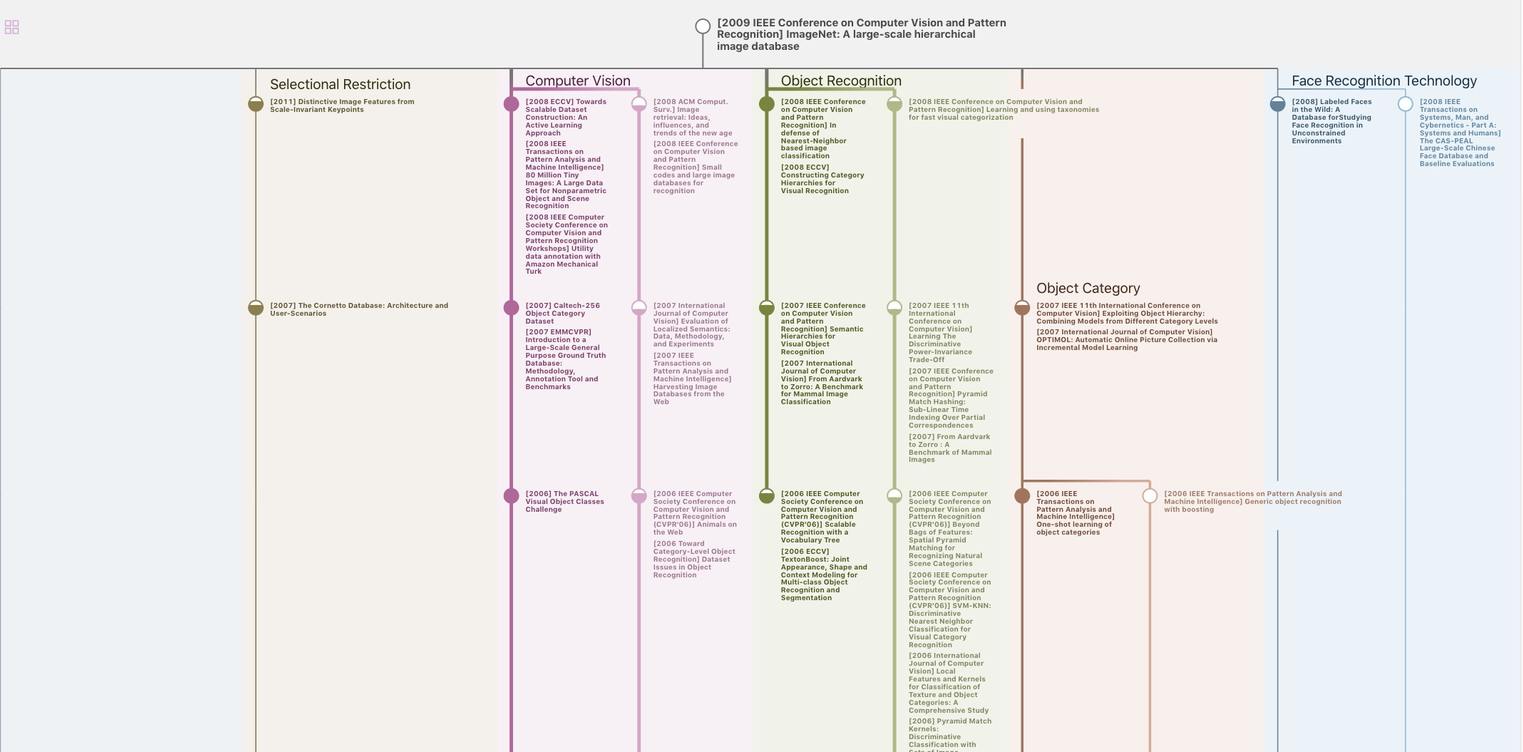
生成溯源树,研究论文发展脉络
Chat Paper
正在生成论文摘要