A Framework on Utilizing of Publicly Availability Stream Gauges Datasets and Deep Learning in Estimating Monthly Basin-scale Runoff in Ungauged Regions
Advances in Water Resources(2024)
摘要
This study introduces a framework that strategically applies a Long Short-Term Memory (LSTM)-based approach for monthly runoff prediction in South Africa and Central Asia. The framework is distinct in its utilization of newly developed Global Land Data Assimilation System (GLDAS)-derived climate dynamics variables and Global Streamflow Indices and Metadata Archives (GSIM)-derived static descriptors, ensuring a consistent evaluation of Deep Learning (DL) performance across multiple continents, including North and South America, and Western Europe. Seven LSTM models were trained using seven different datasets, each representing a combination of these data-rich regions. We assessed the sensitivity of these seven training data sets to LSTM models by testing these trained models in predicting monthly basin-scale runoff across 214 test catchments located in South Africa and Central Asia. Our results show that runoff predictions generated by LSTM within the test domain could exhibit better prediction skills compared to those derived from GLDAS datasets. The performance of the trained LSTMs appears to be linked to hydrological similarities between the data-rich regions and the test basins. Also, our results indicate the importance of selecting the appropriate input sources for the LSTM models to achieve accurate runoff predictions at the test region. We emphasize the possibility of utilizing LSTM models that are leveraging on either North American catchments or a combination of South American and Western European catchments to predict basin-scale runoff in the test regions. To this end, this study harnesses the burgeoning availability of publicly stream-gauge datasets and DL to enhance water information prediction in ungauged regions, responding to the challenge of geographically unbalanced stream gauge instruments.
更多查看译文
关键词
Runoff Prediction,Deep Learning,Basin-scale Runoff,Global Land Data Assimilation System,Climate Dynamic Variables
AI 理解论文
溯源树
样例
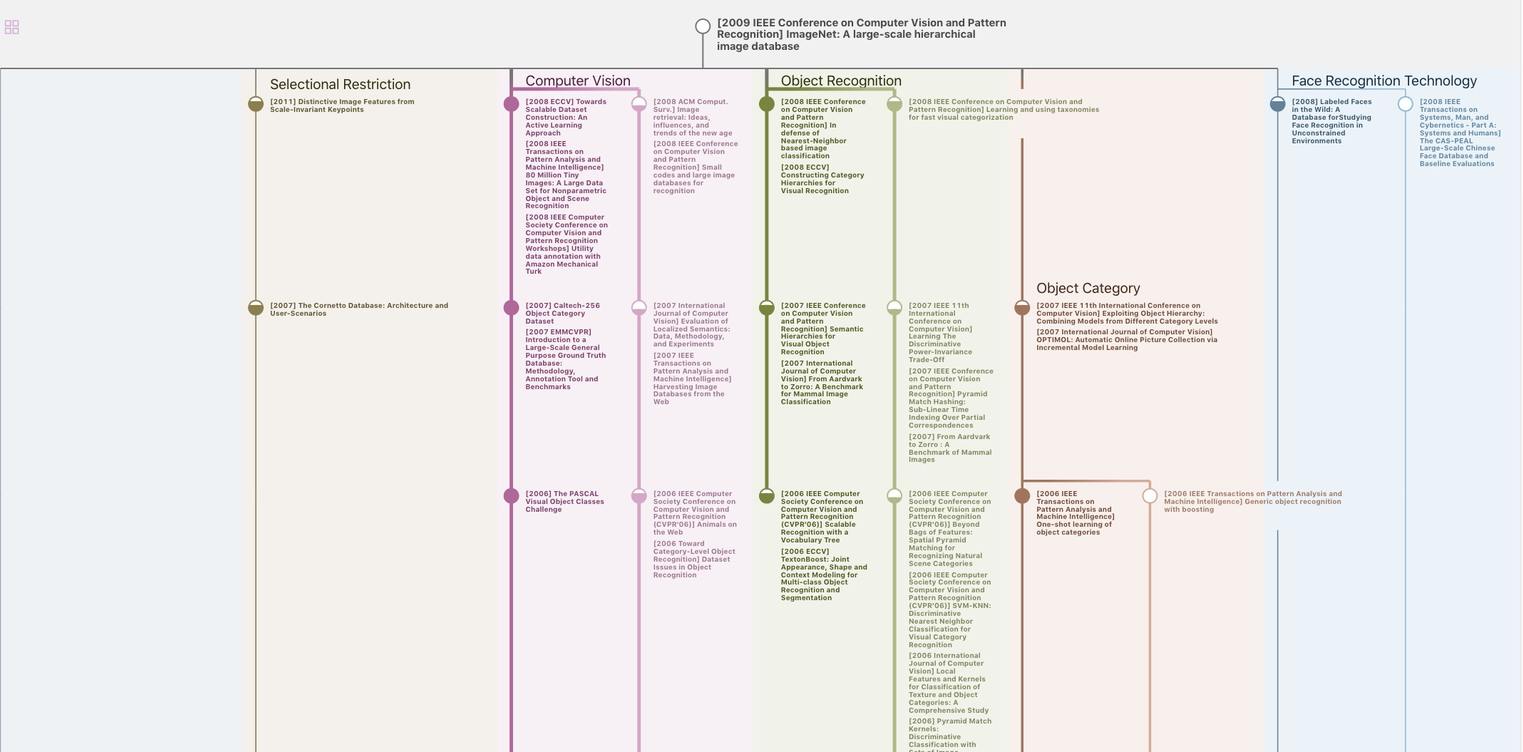
生成溯源树,研究论文发展脉络
Chat Paper
正在生成论文摘要