A DDPG-based Zero-Touch Dynamic Prioritization to Address Starvation of Services for Deploying Microservices-based VNFs
IEEE Transactions on Machine Learning in Communications and Networking(2024)
摘要
The sixth generation of mobile networks (6G) promises applications and services with faster data rates, ultra-reliability, and lower latency compared to the fifth-generation mobile networks (5G). These highly demanding 6G applications will burden the network by imposing stringent performance requirements. Network Function Virtualization (NFV) reduces costs by running network functions as Virtual Network Functions (VNFs) on commodity hardware. While NFV is a promising solution, it poses Resource Allocation (RA) challenges. To enhance RA efficiency, we addressed two critical subproblems: the requirement of dynamic service priority and a low-priority service starvation problem. We introduce ‘Dynamic Prioritization’ (DyPr), employing an ML model to emphasize macro- and microlevel priority for unseen services and address the existing starvation problem in current solutions and their limitations. We present ‘Adaptive Scheduling’ (AdSch), a three-factor approach (priority, threshold waiting time, and reliability) that surpasses traditional priority-based methods. In this context, starvation refers to extended waiting times and the eventual rejection of low-priority services due to a ‘delay. Also, to further investigate, a traffic-aware starvation and deployment problem is studied to enhance efficiency. We employed a Deep Deterministic Policy Gradient (DDPG) model for adaptive scheduling and an online Ridge Regression (RR) model for dynamic prioritization, creating a zero-touch solution. The DDPG model efficiently identified ‘Beneficial and Starving’ services, alleviating the starvation issue by deploying twice as many low-priority services. With an accuracy rate exceeding 80%, our online RR model quickly learns prioritization patterns in under 100 transitions. We categorized services as ‘High-Demand’ (HD) or ‘Not So High Demand’ (NHD) based on traffic volume, providing insight into high revenue-generating services. We achieved a nearly optimal resource allocation by balancing low-priority HD and low-priority NHD services, deploying twice as many low-priority HD services as a model without traffic awareness.
更多查看译文
关键词
6G,Machine Learning,Resource Allocation,Network Function Virtualization,Microservices
AI 理解论文
溯源树
样例
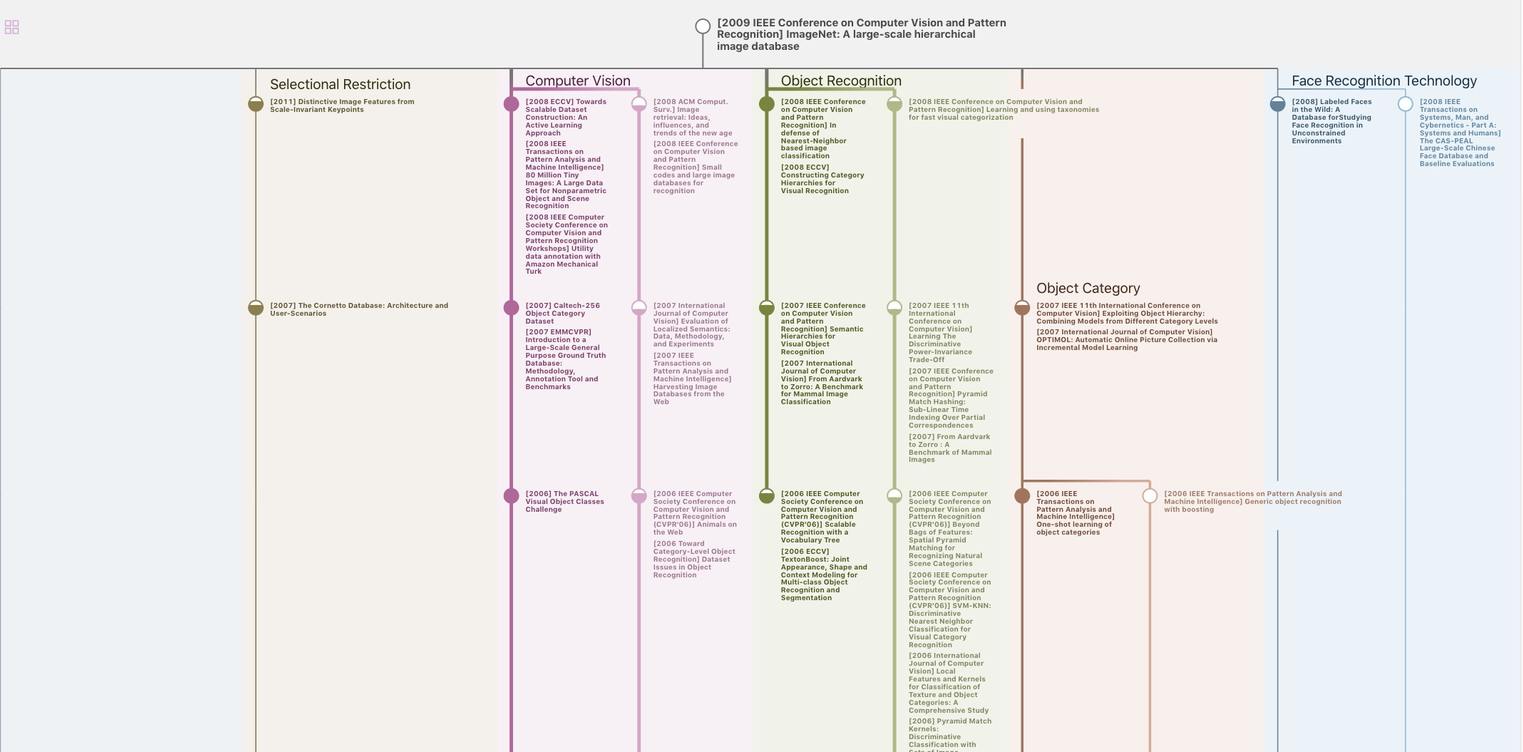
生成溯源树,研究论文发展脉络
Chat Paper
正在生成论文摘要