A Parted-based Method for Unsupervised Visible-Infrared Re-identification.
International Conference on Intelligent Systems and Knowledge Engineering(2023)
摘要
Unsupervised Visible-Infrared Person Re-identification (USL- VI-ReID) problem is the common embranchment of supervised Visible-Infrared Person Re-identification (VI-ReID) and single modality Unsupervised Learning Person Re-identification (USL-ReID). To better target USL-VI-ReID problem, some specific methods are proposed. However, existing methods only mine image features from a global perspective and lack exploration of local details. To fill this gap, we designed a two-staged framework, which consists of a Global and Local Joint Learning (GLJL) framework and Part-based Cross-Modality Alignment (PCMA) method. GLJL aims to build basic local idea application. It segments the global image features into parts to extract local features. These local features are combined with global features for training, which enables the model to focus both global information and local details. With GLJL, the model has better performance in intra-modality learning stage. Based on GLJL, we design PCMA method to further overcome cross-modality differences. PCMA matches cross-modality pseudo labels at both global and local scale, which not only reduces modality gap, but also avoids the model being affected by environmental noise due to excessive attention to local details. With PCMA, the model shows effective improvement compared to GLJL. Extensive experiments prove that our method is effective in improving the performance compared other USL-VI-ReID methods.
更多查看译文
关键词
person re-identification,unsupervised learning,cross modality,local segmentation
AI 理解论文
溯源树
样例
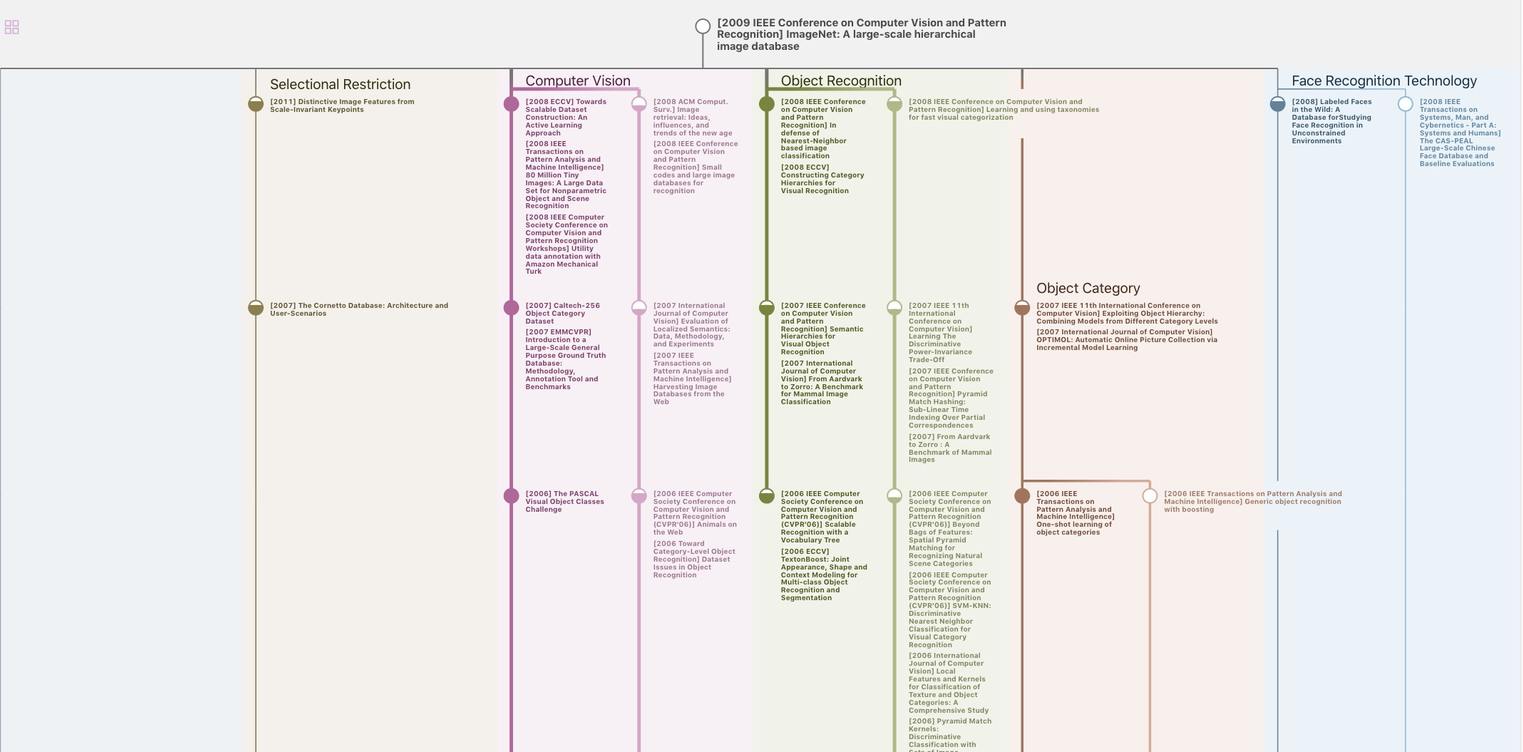
生成溯源树,研究论文发展脉络
Chat Paper
正在生成论文摘要