Breast Cancer Detection Using Deep Convolutional Neural Network: A pre-processing-Driven Approach
2023 30th National and 8th International Iranian Conference on Biomedical Engineering (ICBME)(2023)
摘要
Breast cancer is the leading cause of mortality among women worldwide. Early detection of breast cancer is crucial in preventing the spread of cancerous cells to adjacent tissues. Digital mammography has emerged as a pivotal tool for detecting breast cancer at its early stage. Recent advancements in deep learning have shown remarkable potential for automating breast cancer detection. This paper introduces a completely automated framework that assists radiologists in efficiently diagnosing breast lesions. The proposed framework comprises two phases: a pre-processing phase and a model design phase. The pre-processing phase involves essential steps, format conversion, artifact removal, Wiener denoising, contrast enhancement via CLAHE, ROI patching, resizing, and augmentation, to prepare mammograms for model training. In the model design phase, four pre-trained Deep Convolutional Neural Networks - ResNet34, DenseNet121, Inception V1, and Efficient B0 - are developed to accurately classify breast lesions in mammography images as normal or abnormal. The proposed model is evaluated using the Mammographic Image Analysis Society (MIAS) dataset, demonstrating significant achievements. The achieved metrics for accuracy, precision, specificity, sensitivity, F1-score, and AUC are 99.2%, 99.2%, 99.2%, 99.2%, 99.2%, and 99.1%, respectively, outperforming state-of-the-art methodologies in breast cancer detection.
更多查看译文
关键词
Breast cancer,Deep learning,Image classification,Mammography,Pre-processing
AI 理解论文
溯源树
样例
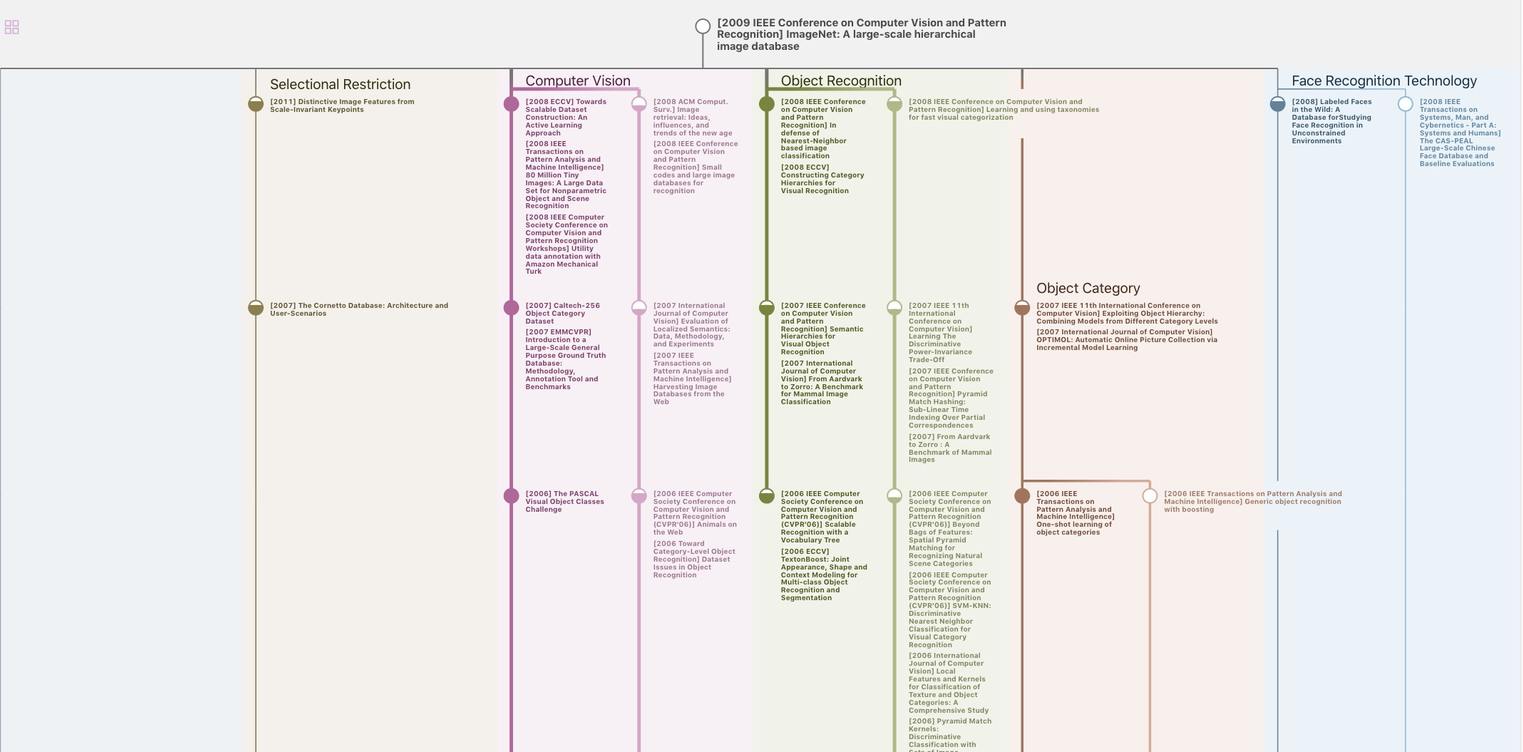
生成溯源树,研究论文发展脉络
Chat Paper
正在生成论文摘要