Dynamic Treatment Regimes with Replicated Observations Available for Error-prone Covariates: a Q-learning Approach
arxiv(2024)
摘要
Dynamic treatment regimes (DTRs) have received an increasing interest in
recent years. DTRs are sequences of treatment decision rules tailored to
patient-level information. The main goal of the DTR study is to identify an
optimal DTR, a sequence of treatment decision rules that yields the best
expected clinical outcome. Q-learning has been considered as one of the most
popular regression-based methods to estimate the optimal DTR. However, it is
rarely studied in an error-prone setting, where the patient information is
contaminated with measurement error. In this paper, we study the effect of
covariate measurement error on Q-learning and propose a correction method to
correct the measurement error in Q-learning. Simulation studies are conducted
to assess the performance of the proposed method in Q-learning. We illustrate
the use of the proposed method in an application to the sequenced treatment
alternatives to relieve depression data.
更多查看译文
AI 理解论文
溯源树
样例
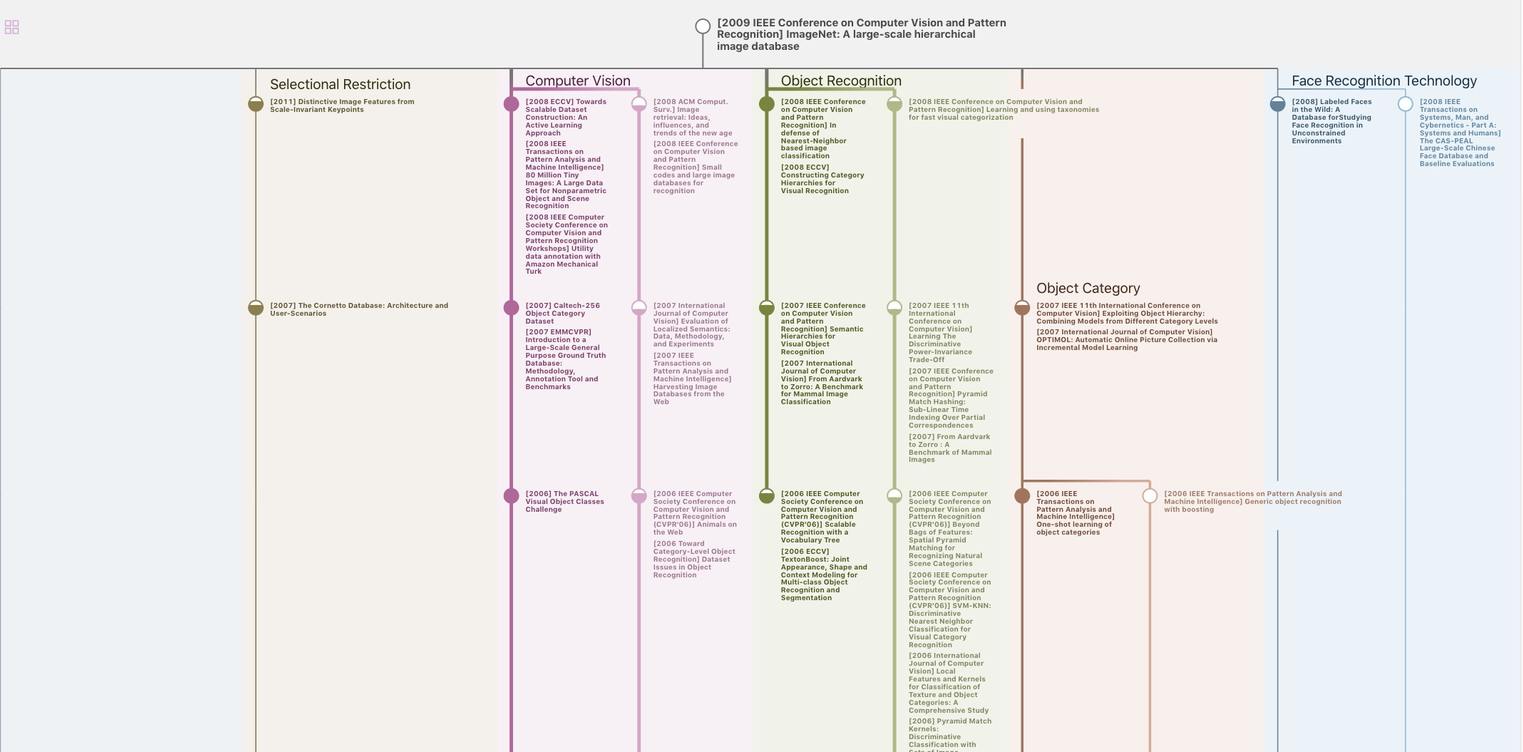
生成溯源树,研究论文发展脉络
Chat Paper
正在生成论文摘要