Transforming Surgical Training: A Systematic Review of AI Techniques for Assessment and Evaluation (Preprint)
crossref(2024)
摘要
BACKGROUND Artificial intelligence (AI) has introduced novel opportunities for assessment and evaluation in surgical training, offering potential improvements that could surpass traditional educational methods. OBJECTIVE This systematic review explores the integration of AI in surgical training, assessment, and evaluation with the objective of determining how AI technologies can enhance trainees’ learning paths and performance by incorporating data-driven insights and predictive analytics. Moreover, this study review aims to examine the current state and applications of AI algorithms in this field, identifying potential areas for future research. METHODS Adhering to the Preferred Reporting Items for Systematic Reviews and Meta-Analyses (PRISMA) guidelines and software engineering review protocols, this systematic review searched PubMed, Scopus, and Web of Science databases for relevant studies published from 2020 to 2024. The review is structured around four research questions: the specific surgical procedures to which AI is applied, the AI techniques used, how AI assesses and improves surgical training, and its effects on trainees' learning curves. RESULTS From an initial 1,400 records, 56 studies met the inclusion criteria and were analyzed. AI applications have been used extensively in the fields of minimally invasive surgery (MIS), neurosurgery, and laparoscopy, with machine learning (ML) and deep learning (DL) being the most common techniques used in the field. Moreover, simulation training was identified as the predominant setup. Several studies also reported significant improvements in trainees' learning curves when AI was incorporated into training protocols, although some authors pointed out the importance of further studies in this area. CONCLUSIONS The use of AI in surgical training has exhibited the capacity to augment the process of learning and acquiring skills, hence facilitating the transition towards educational models that are more efficient, personalized, and adaptive. Future studies should aim to clarify AI methodologies and focus on scalable solutions that align with universal education goals. Moreover, simulation training emerges as a critical area for ongoing research and development, particularly including ML and DL techniques to design more insightful experiences.
更多查看译文
AI 理解论文
溯源树
样例
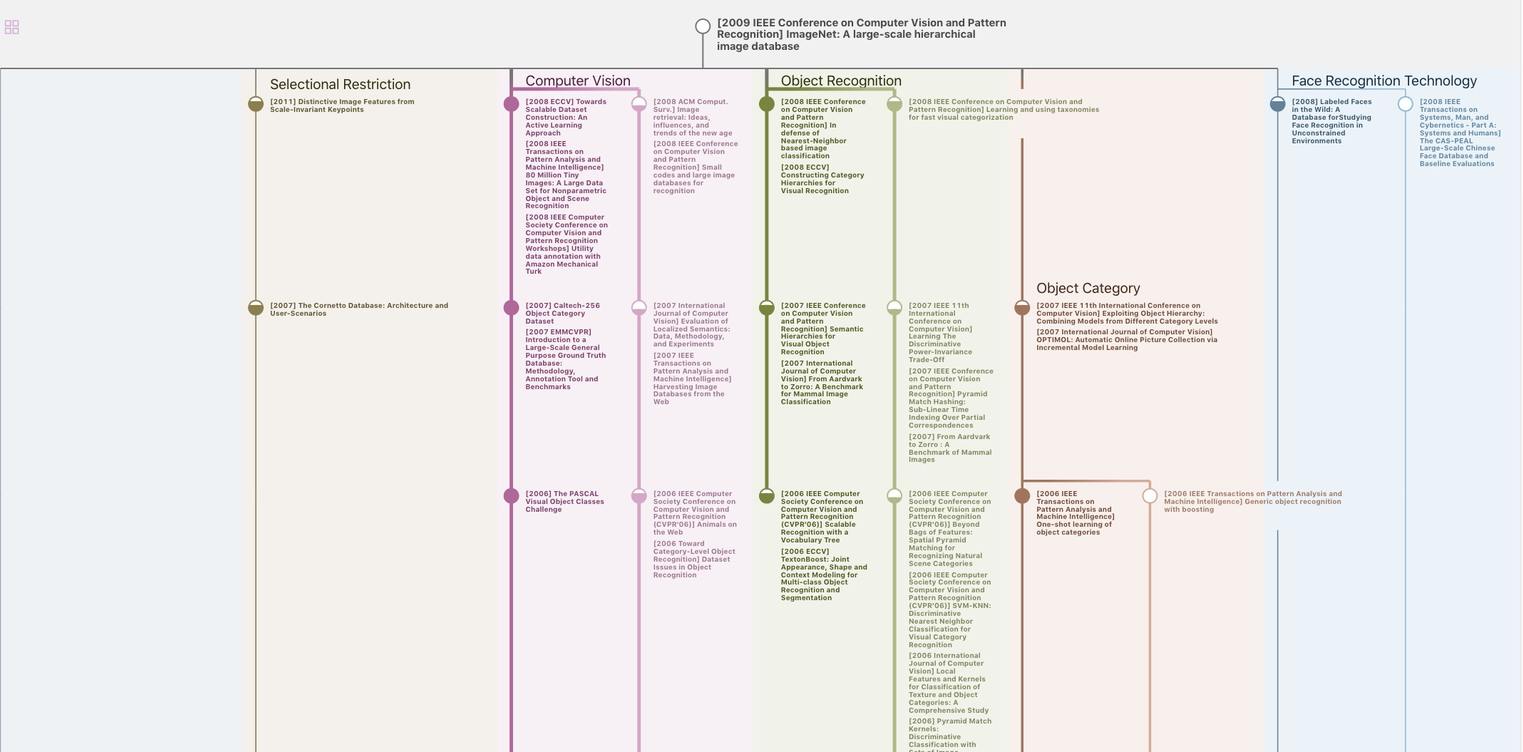
生成溯源树,研究论文发展脉络
Chat Paper
正在生成论文摘要