Modeling Implied Volatility Surface Using B-Splines with Time-Dependent Coefficients Predicted by Tree-Based Machine Learning Methods
MATHEMATICS(2024)
摘要
Implied volatility is known to have a string structure (smile curve) for a given time to maturity and can be captured by the B-spline. The parameters characterizing the curves can change over time, which complicates the modeling of the implied volatility surface. Although machine learning models could improve the in-sample fitting, they ignore the structure in common over time and might have poor prediction power. In response to these challenges, we propose a two-step procedure to model the dynamic implied volatility surface (IVS). In the first step, we construct the bivariate tensor-product B-spline (BTPB) basis to approximate cross-sectional structures, under which the surface can be represented by a vector of coefficients. In the second step, we allow for the time-dependent coefficients and model the dynamic coefficients with the tree-based method to provide more flexibility. We show that our approach has better performance than the traditional linear models (parametric models) and the tree-based machine learning methods (nonparametric models). The simulation study confirms that the tensor-product B-spline is able to capture the classical parametric model for IVS given different sample sizes and signal-to-noise ratios. The empirical study shows that our two-step approach outperforms the traditional parametric benchmark, nonparametric benchmark, and parametric benchmark with time-varying coefficients in predicting IVS for the S&P 500 index options in the US market.
更多查看译文
关键词
implied volatility surface,forecasting,tensor-product B-spline,tree-based machine learning methods
AI 理解论文
溯源树
样例
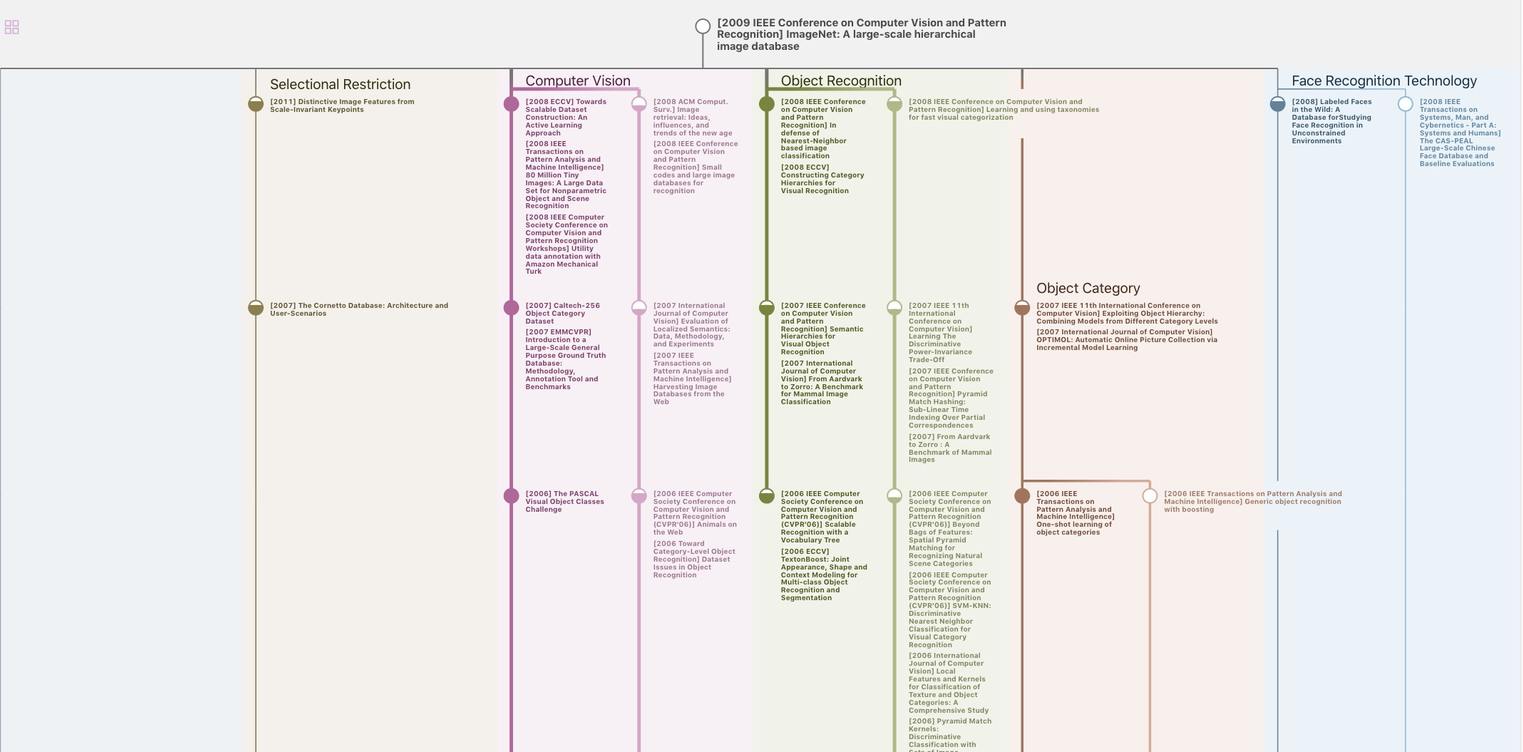
生成溯源树,研究论文发展脉络
Chat Paper
正在生成论文摘要