Prediction of polyspecificity from antibody sequence data by machine learning
FRONTIERS IN BIOINFORMATICS(2024)
摘要
Antibodies are generated with great diversity in nature resulting in a set of molecules, each optimized to bind a specific target. Taking advantage of their diversity and specificity, antibodies make up for a large part of recently developed biologic drugs. For therapeutic use antibodies need to fulfill several criteria to be safe and efficient. Polyspecific antibodies can bind structurally unrelated molecules in addition to their main target, which can lead to side effects and decreased efficacy in a therapeutic setting, for example via reduction of effective drug levels. Therefore, we created a neural-network-based model to predict polyspecificity of antibodies using the heavy chain variable region sequence as input. We devised a strategy for enriching antibodies from an immunization campaign either for antigen-specific or polyspecific binding properties, followed by generation of a large sequencing data set for training and cross-validation of the model. We identified important physico-chemical features influencing polyspecificity by investigating the behaviour of this model. This work is a machine-learning-based approach to polyspecificity prediction and, besides increasing our understanding of polyspecificity, it might contribute to therapeutic antibody development.
更多查看译文
关键词
neural network,immunoglobulin,immune repertoire,polyspecificity,antibody,therapeutic antibodies,deep learning,machine learning
AI 理解论文
溯源树
样例
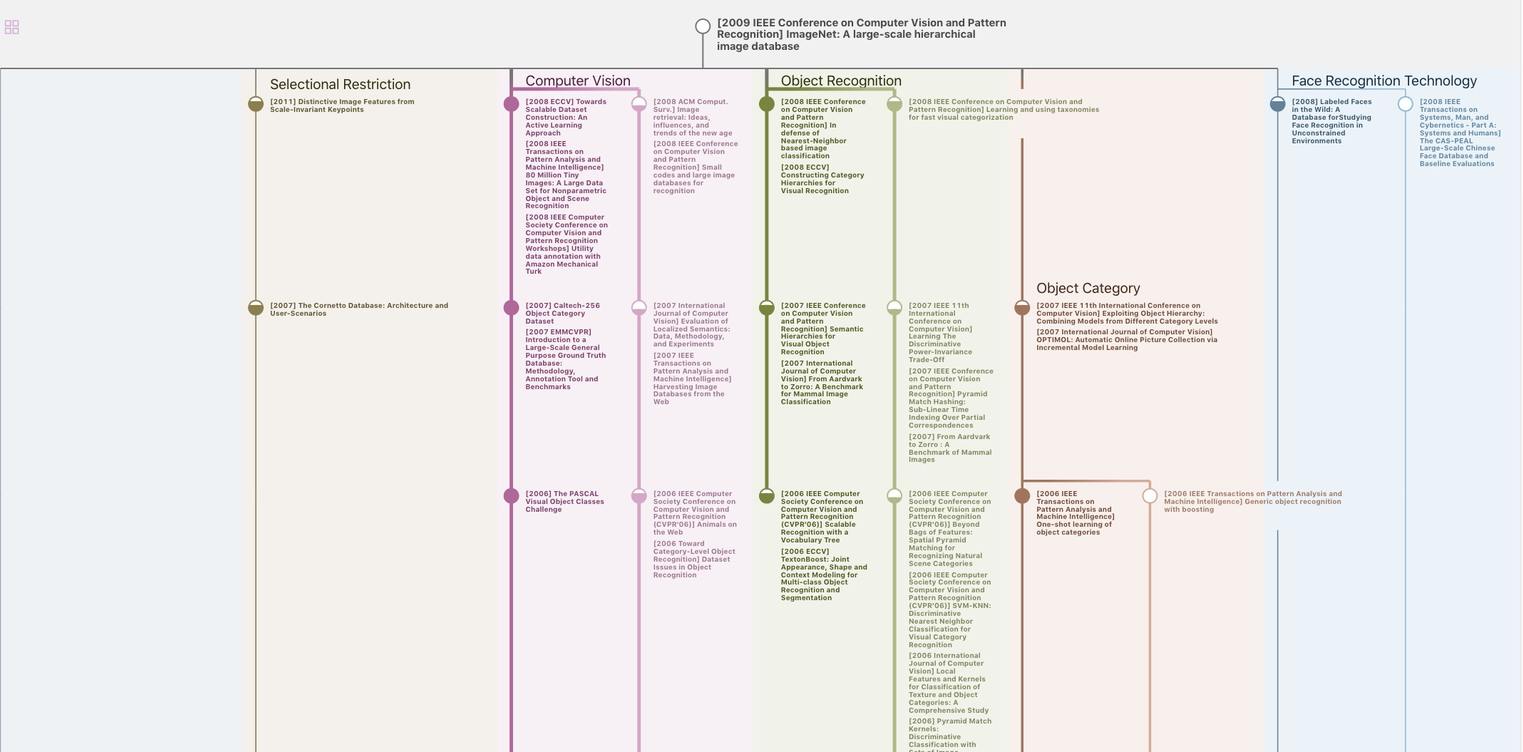
生成溯源树,研究论文发展脉络
Chat Paper
正在生成论文摘要