Optimizing Dynamic Cache Allocation in Vehicular Edge Networks: A Method Combining Multisource Data Prediction and Deep Reinforcement Learning
IEEE INTERNET OF THINGS JOURNAL(2024)
摘要
Currently, most studies on content caching strategies for vehicular networks rely on the Zipf distribution model of content popularity. This approach, however, often fails to accommodate the time-varying nature of content popularity and vehicular traffic. Although some studies have begun considering time-varying content popularity, they overlook the implications of time-varying vehicle traffic, which significantly influences content allocation caching policies and could potentially reduce edge server rental costs for service providers. Addressing this gap, our study takes both time-varying content popularity and vehicle traffic data into account. From the perspective of content service providers, we need to strike a tradeoff in optimizing the hit rate (HR) based on the foundation of utility enhancement. Accordingly, we model the problem as a multiobjective issue that jointly optimizes HR, utility, and replacement cost. By employing a scalar function, we transform this multiobjective problem into a single-objective one, allowing for the modulation of optimization objective priorities via weight tuning. We employ two gated recurrent unit networks to predict both vehicle traffic volume and content popularity for the next moment. We use the predicted area traffic flow volume and content popularity as the system state for our cache allocation decision optimization, which is accomplished using a deep reinforcement learning model. We conducted a series of evaluation experiments using real data sets, and our proposed approach significantly outperforms the baseline scheme.
更多查看译文
关键词
Content caching,deep reinforcement learning (DRL),gated recurrent unit (GRU),vehicular edge networks
AI 理解论文
溯源树
样例
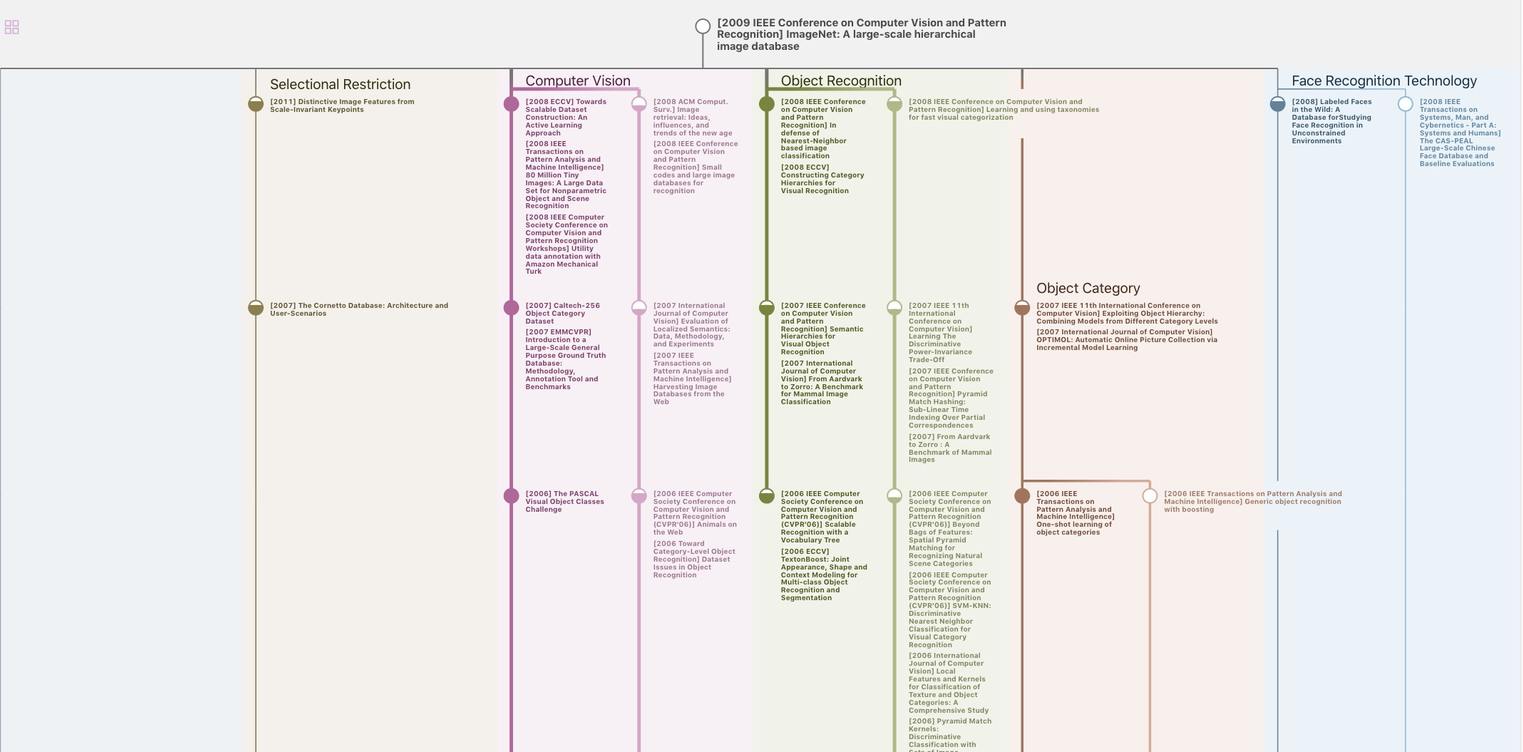
生成溯源树,研究论文发展脉络
Chat Paper
正在生成论文摘要